filipradenovic / Cnnimageretrieval
Programming Languages
Projects that are alternatives of or similar to Cnnimageretrieval
CNN Image Retrieval in MatConvNet: Training and evaluating CNNs for Image Retrieval in MatConvNet
This is a MATLAB toolbox that implements the training and testing of the approach described in our papers:
Deep Shape Matching,
Radenović F., Tolias G., Chum O.,
ECCV 2018 [arXiv]
Fine-tuning CNN Image Retrieval with No Human Annotation,
Radenović F., Tolias G., Chum O.,
TPAMI 2018 [arXiv]
CNN Image Retrieval Learns from BoW: Unsupervised Fine-Tuning with Hard Examples,
Radenović F., Tolias G., Chum O.,
ECCV 2016 [arXiv]
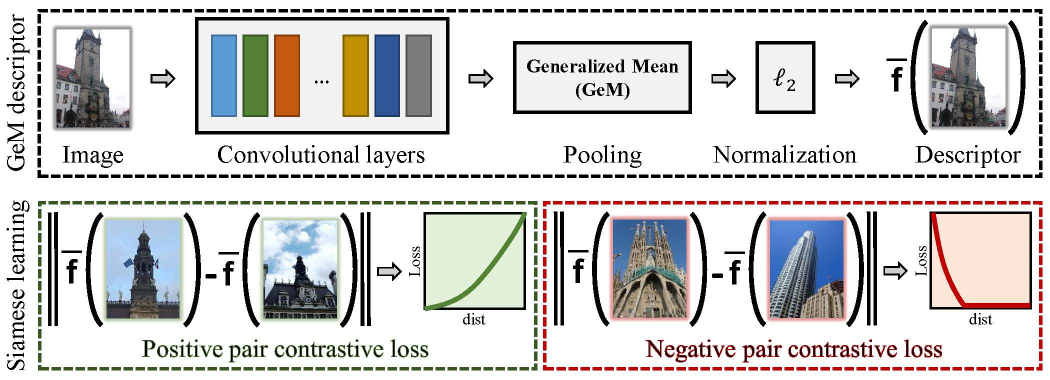
Prerequisites
In order to run this toolbox you will need:
- MATLAB (tested with MATLAB R2017a on Debian 8.1)
- MatConvNet MATLAB toolbox version 1.0-beta25
- All the rest (data + networks) is automatically downloaded with our scripts
Image retrieval
This code implements:
- Training (fine-tuning) CNN for image retrieval
- Learning supervised whitening for CNN image representations
- Testing CNN image retrieval on Oxford5k and Paris6k datasets
Run the following script in MATLAB:
>> run [MATCONVNET_ROOT]/matlab/vl_setupnn;
>> run [CNNIMAGERETRIEVAL_ROOT]/setup_cnnimageretrieval;
>> train_cnnimageretrieval;
>> test_cnnimageretrieval;
See [CNNIMAGERETRIEVAL_ROOT]/examples/train_cnnimageretrieval
and [CNNIMAGERETRIEVAL_ROOT]/examples/test_cnnimageretrieval
for additional details.
We provide the pretrained networks trained using the same parameters as in our ECCV 2016 and TPAMI 2018 papers. Performance comparison with the networks trained with our CNN Image Retrieval in PyTorch, on the original and the revisited Oxford and Paris benchmarks:
Model | Oxford | Paris | ROxf (M) | RPar (M) | ROxf (H) | RPar (H) |
---|---|---|---|---|---|---|
VGG16-GeM (MatConvNet) | 87.9 | 87.7 | 61.9 | 69.3 | 33.7 | 44.3 |
VGG16-GeM (PyTorch) | 87.2 | 87.8 | 60.5 | 69.3 | 32.4 | 44.3 |
ResNet101-GeM (MatConvNet) | 87.8 | 92.7 | 64.7 | 77.2 | 38.5 | 56.3 |
ResNet101-GeM (PyTorch) | 88.2 | 92.5 | 65.3 | 76.6 | 40.0 | 55.2 |
Note: Data and networks used for training and testing are automatically downloaded when using the example scripts.
Sketch-based image retrieval and shape matching
This code implements:
- Training (fine-tuning) CNN for sketch-based image retrieval and shape matching
- Testing CNN sketch-based image retrieval on Flickr15k dataset
Run the following script in MATLAB:
>> run [MATCONVNET_ROOT]/matlab/vl_setupnn;
>> run [CNNIMAGERETRIEVAL_ROOT]/setup_cnnimageretrieval;
>> train_cnnsketch2imageretrieval;
>> test_cnnsketch2imageretrieval;
See [CNNIMAGERETRIEVAL_ROOT]/examples/train_cnnsketch2imageretrieval
and [CNNIMAGERETRIEVAL_ROOT]/examples/test_cnnsketch2imageretrieval
for additional details.
We provide the pretrained networks trained using the same parameters as in our ECCV 2018 paper. The Flickr15k dataset used in the paper is slightly outdated compared to the latest one that is automatically downloaded when using this code (0.1 difference in mAP), so we report results here:
EdgeMAC components | ||||
---|---|---|---|---|
Fine-tuned | x | x | x | x |
Mirror | x | x | ||
Multi-scale | x | x | ||
mAP | 42.0 | 43.5 | 45.7 | 46.2 |
Note: Data and networks used for testing are automatically downloaded when using the example scripts.
Related publications
Image retrieval
@article{RTC18a,
title = {Fine-tuning {CNN} Image Retrieval with No Human Annotation},
author = {Radenovi{\'c}, F. and Tolias, G. and Chum, O.}
journal = {TPAMI},
year = {2018}
}
@inproceedings{RTC16,
title = {{CNN} Image Retrieval Learns from {BoW}: Unsupervised Fine-Tuning with Hard Examples},
author = {Radenovi{\'c}, F. and Tolias, G. and Chum, O.},
booktitle = {ECCV},
year = {2016}
}
Sketch-based image retrieval and shape matching
@article{RTC18b,
title = {Deep Shape Matching},
author = {Radenovi{\'c}, F. and Tolias, G. and Chum, O.}
journal = {ECCV},
year = {2018}
}
Revisited benchmarks for Oxford and Paris ('roxford5k' and 'rparis6k')
@inproceedings{RITAC18,
author = {Radenovi{\'c}, F. and Iscen, A. and Tolias, G. and Avrithis, Y. and Chum, O.},
title = {Revisiting Oxford and Paris: Large-Scale Image Retrieval Benchmarking},
booktitle = {CVPR},
year = {2018}
}