RockyXu66 / Faster_rcnn_for_open_images_dataset_keras
Labels
Projects that are alternatives of or similar to Faster rcnn for open images dataset keras
Faster R-CNN for Open Images Dataset by Keras
Introduction
The original code of Keras version of Faster R-CNN I used was written by yhenon (resource link: GitHub .) He used the PASCAL VOC 2007, 2012, and MS COCO datasets. For me, I just extracted three classes, “Person”, “Car” and “Mobile phone”, from Google’s Open Images Dataset V4. I applied configs different from his work to fit my dataset and I removed unuseful code. Btw, to run this on Google Colab (for free GPU computing up to 12hrs), I compressed all the code into three .ipynb notebooks. Sorry for the messy structure.
I wrote my exploring and experiment results for Faster R-CNN in this article in Medium. If you are in China, you cannot directly access Medium. So I make a copy in here.
Project Structure
Object_Detection_DataPreprocessing.ipynb
is the file to extract subdata from Open Images Dataset V4 which includes downloading the images and creating the annotation files for our training. I run this part by my own computer because of no need for GPU computation. frcnn_train_vgg.ipynb
is the file to train the model. The configuration and model saved path are inside this file. frcnn_test_vgg.ipynb
is the file to test the model with test images and calculate the mAP (mean average precision) for the model. If you want to run the code on Colab, you need to give authority to Colab for connecting your Google Drive. Then, you need to upload your annotation file and training images to the Google Drive and change my path to your right path in the notebook.
Result for some test images
Have fun
As the images shown below, they are three porcoelainous monks made by China. I just named them according to their face look (not sure about the sleepy one). They are definitely not included in the Open Images Dataset V4. Except for the three classes I used in Open Images Dataset V4, I create my own six-classes dataset ('Apple Pen', 'Lipbalm', 'Scissor', 'Sleepy Monk', 'Upset Monk' and 'Happy Monk') for fun and train another detector to find out these objects. If you want to know how to do this, just see this article for more details.
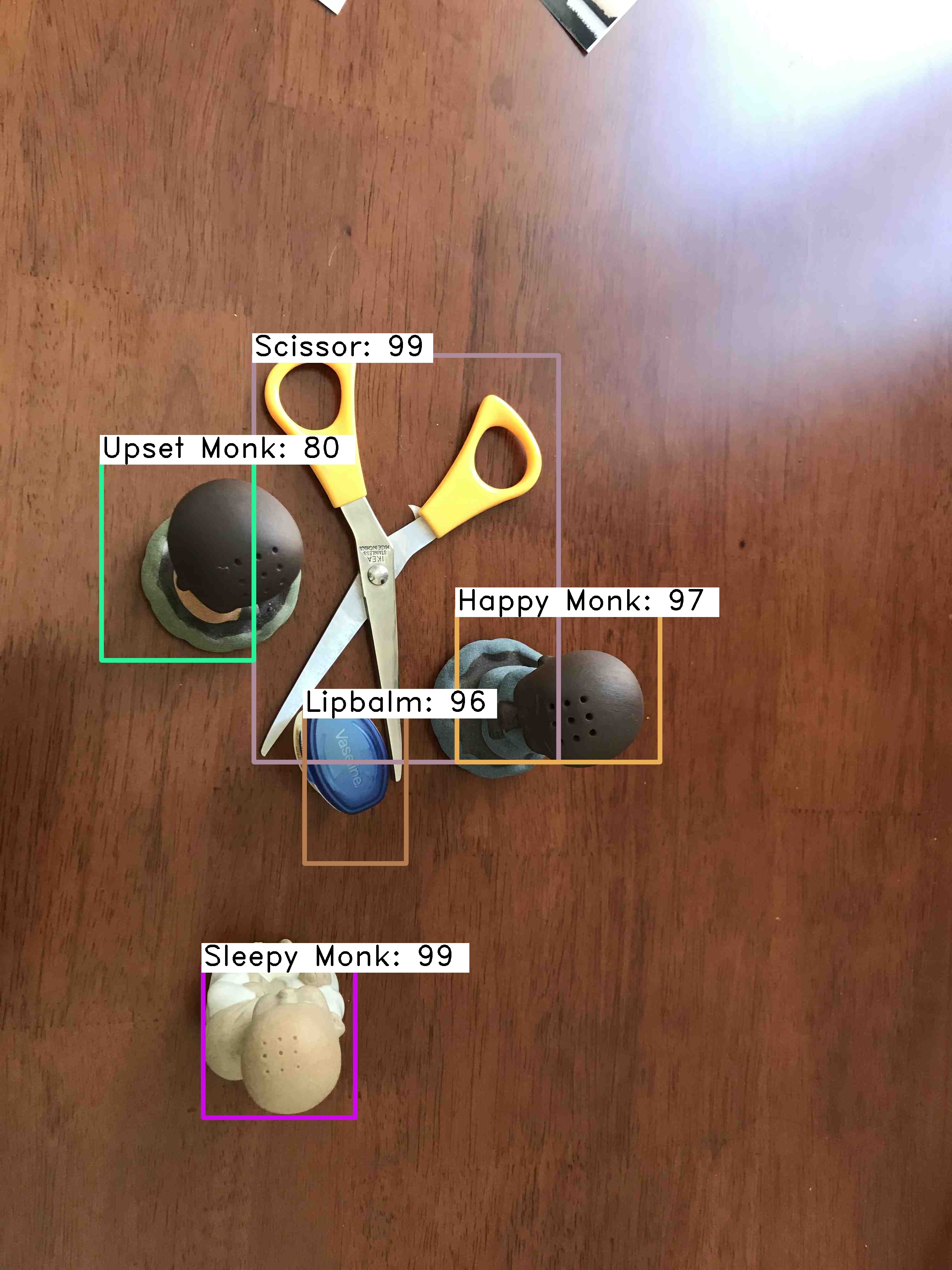