lrjconan / Gran
Labels
Projects that are alternatives of or similar to Gran
GRAN
This is the official PyTorch implementation of Efficient Graph Generation with Graph Recurrent Attention Networks as described in the following NeurIPS 2019 paper:
@inproceedings{liao2019gran,
title={Efficient Graph Generation with Graph Recurrent Attention Networks},
author={Liao, Renjie and Li, Yujia and Song, Yang and Wang, Shenlong and Nash, Charlie and Hamilton, William L. and Duvenaud, David and Urtasun, Raquel and Zemel, Richard},
booktitle={NeurIPS},
year={2019}
}
Visualization
Generation of GRAN per step:
Overall generation process:
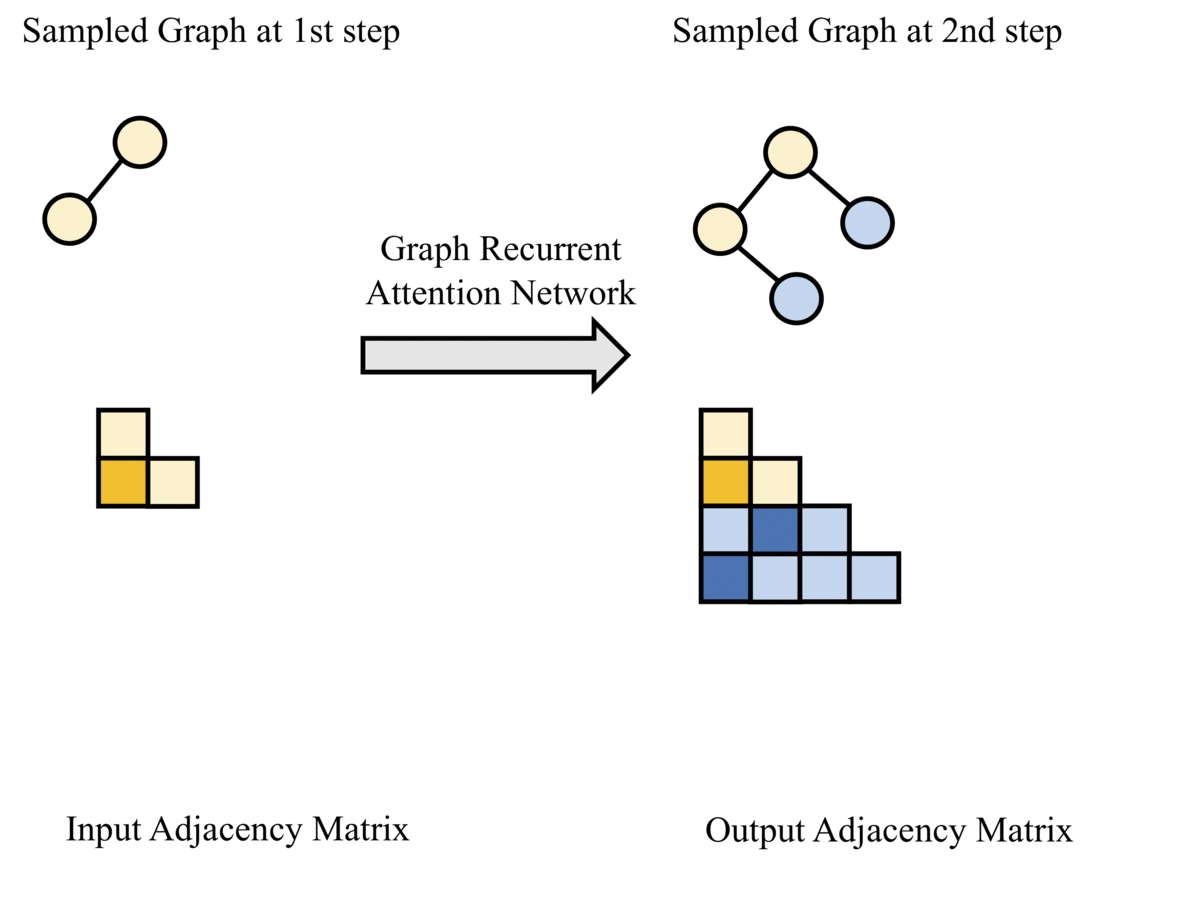
Dependencies
Python 3, PyTorch(1.2.0)
Other dependencies can be installed via
pip install -r requirements.txt
Run Demos
Train
-
To run the training of experiment
X
whereX
is one of {gran_grid
,gran_DD
,gran_DB
,gran_lobster
}:python run_exp.py -c config/X.yaml
Note:
- Please check the folder
config
for a full list of configuration yaml files. - Most hyperparameters in the configuration yaml file are self-explanatory.
Test
-
After training, you can specify the
test_model
field of the configuration yaml file with the path of your best model snapshot, e.g.,test_model: exp/gran_grid/xxx/model_snapshot_best.pth
-
To run the test of experiments
X
:python run_exp.py -c config/X.yaml -t
Note:
- Please check the evaluation to set up.
Trained Models
-
You could use our trained model for comparisons. Please make sure you are using the same split of the dataset. Running the following script will download the trained model:
./download_model.sh
Sampled Graphs from GRAN
Cite
Please cite our paper if you use this code in your research work.
Questions/Bugs
Please submit a Github issue or contact [email protected] if you have any questions or find any bugs.