jessemelpolio / Non Stationary_texture_syn
Programming Languages
Labels
Projects that are alternatives of or similar to Non Stationary texture syn
Non-stationary texture synthesis using adversarial expansions
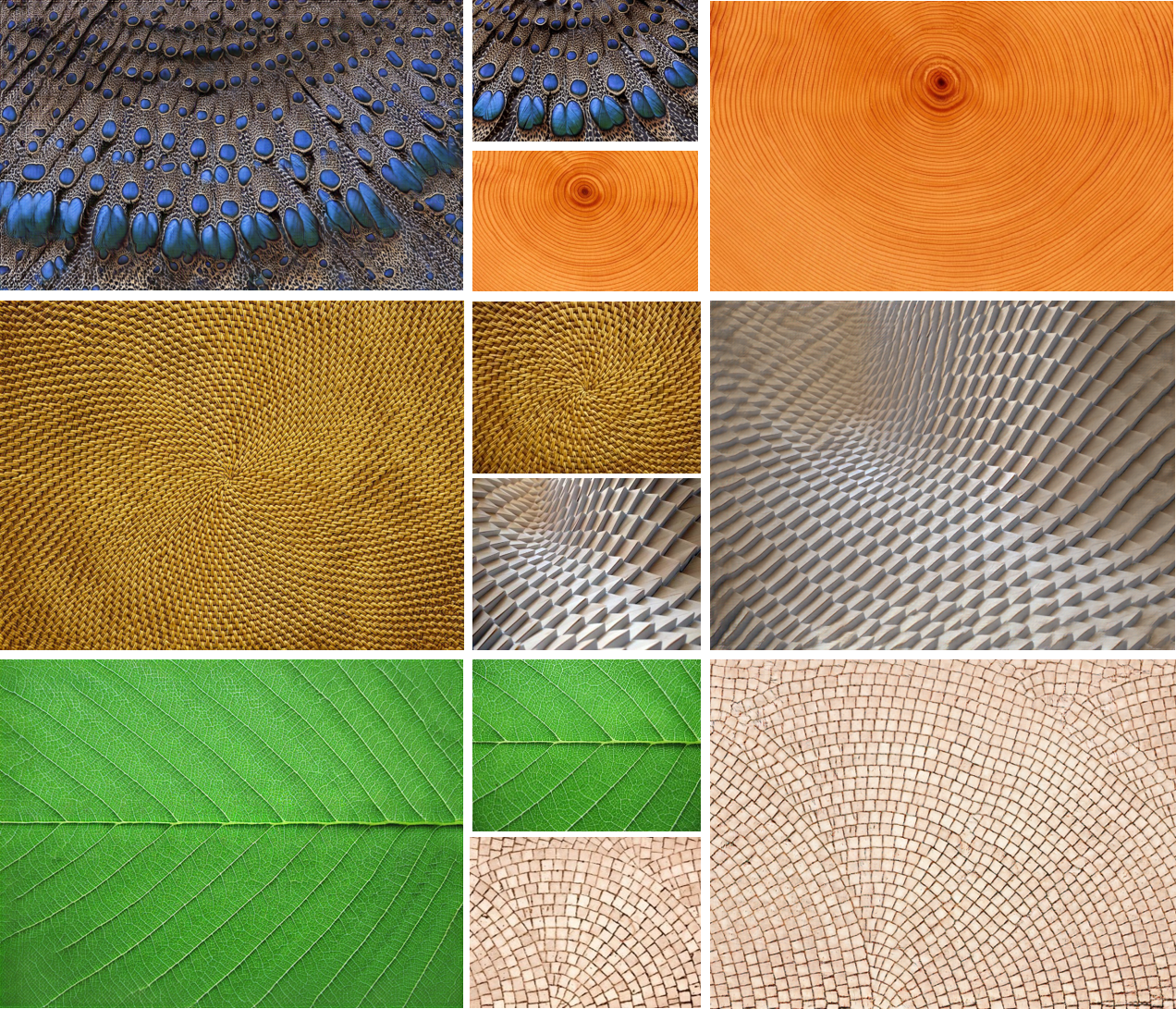
This is the official code of paper Non-stationary texture synthesis using adversarial expansions.
This code was mainly adapted by Zhen Zhu on the basis of the repository CycleGAN.
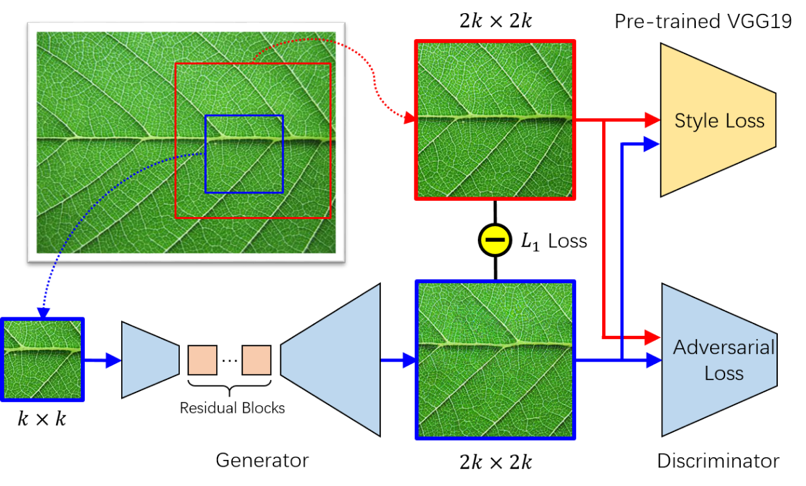
If you use this code for your research, please cite:
Non-stationary texture synthesis using adversarial expansions
Yang Zhou*, Zhen Zhu*, Xiang Bai, Dani Lischinski, Daniel Cohen-Or, Hui Huang
In SIGGRAPH 2018. (* equal contributions)
Requirements
This code is tested under Ubuntu 14.04 and 16.04. The total project can be well functioned under the following environment:
- python-2.7
- pytorch-0.3.0 with cuda correctly specified
- cuda-8.0
- other packages under python-2.7
Preparations
Please run download_pretrained_models.sh
first to make a new folder Models
and then download the VGG19 model pre-trained on ImageNet to this folder. The pre-trained VGG19 model is used to calculate style loss.
Data
There is no restriction for the format of the source texture images. The structure of the data folder is recommanded as the provided sub-folders inside datasets
folder. To be more specific, datasets/half
is what we use in paper production.
The dataset structure is recommended as:
+--half
|
| +--sunflower
|
| +--train
|
| +--sunflower.jpg
|
| +--test
|
| +--sunflower.jpg
|
| +--brick
|
| +--train
|
| +--brick.jpg
|
| +--test
|
| +--brick.jpg
|
...
Architecture of the repository
Inside the main folder, train.py
is used to train a model as described in our paper. test.py
is used to test with the original image(result is 2x the size of the input). test_recurrent.py
is used for extreme expansions. cnn-vis.py
is used to visualize the internal layers of our generator. The residual blocks visualization shown in our paper are generated through cnn-vis.py
.
In folder data
, file custom_dataset_data_loader
specified five dataset mode: aligned
, unaligned
, single
and half_crop
. Generally, we use single
for testing and half_crop
for training.
In folder models
, two files are of great importance: models.py
and networks.py
, please carefully check it before using it. half_gan_style.py
is the major model we use in our paper. Some utilities are implemented in vgg.py
.
In folder options
, all hyperparameters are defined here. Go to this folder to see the meaning of every hyperparameter.
Folder scripts
contains scripts used for training and testing. To train or test a model, use commands like sh scripts/train_half_style.sh
. Go into these files to see how to specify some hyper parameters.
Folder util
contains some scripts to generate perlin noise (perlin2d.py), generate random tile (random_tile.py), which are useful to replicate our paper's results. Some other useful scripts are also included.
Train, test and visualize
Folder scripts
contain scripts used for training and testing. To train or test a model, use commands like sh scripts/train_half_style.sh
. Go into these files to see how to specify some hyper parameters. To visualize the internal layers inside network, especially the residual blocks, you can use script visualize_layers.sh
, as shown in our paper.
Cite
If you use this code for your research, please cite our paper:
@article{TexSyn18,
title = {Non-stationary Texture Synthesis by Adversarial Expansion},
author = {Yang Zhou and Zhen Zhu and Xiang Bai and Dani Lischinski and Daniel Cohen-Or and Hui Huang},
journal = {ACM Transactions on Graphics (Proc. SIGGRAPH)},
volume = {37},
number = {4},
pages = {},
year = {2018},
}
Acknowledgements
The code is based on project CycleGAN. We sincerely thank for their great work.