google-research / Seed_rl
Programming Languages
Projects that are alternatives of or similar to Seed rl
SEED
This repository contains an implementation of distributed reinforcement learning agent where both training and inference are performed on the learner.
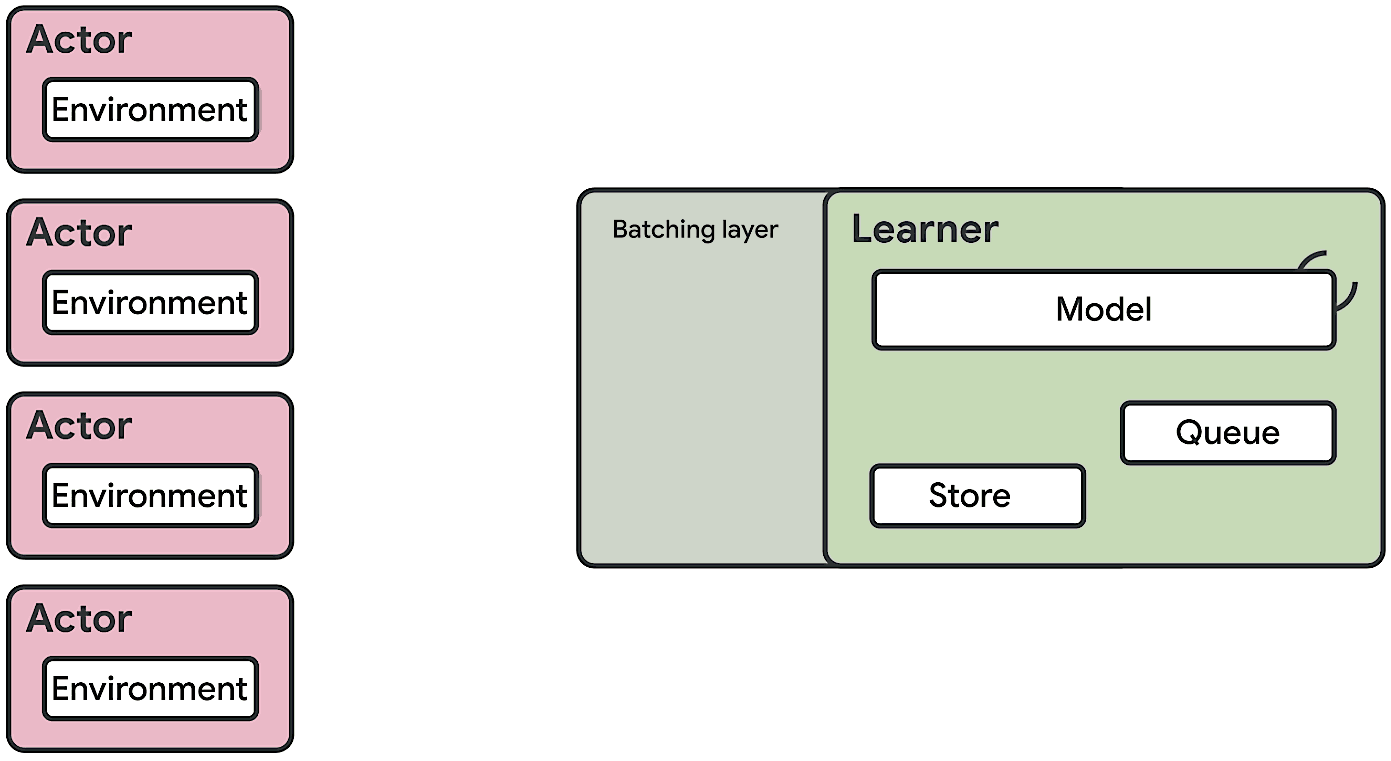
Four agents are implemented:
-
IMPALA: Scalable Distributed Deep-RL with Importance Weighted Actor-Learner Architectures
-
R2D2 (Recurrent Experience Replay in Distributed Reinforcement Learning)
-
Configurable On-Policy Agent implementing the following algorithms:
The code is already interfaced with the following environments:
However, any reinforcement learning environment using the gym API can be used.
For a detailed description of the architecture please read our paper. Please cite the paper if you use the code from this repository in your work.
Bibtex
@article{espeholt2019seed,
title={SEED RL: Scalable and Efficient Deep-RL with Accelerated Central Inference},
author={Lasse Espeholt and Rapha{\"e}l Marinier and Piotr Stanczyk and Ke Wang and Marcin Michalski},
year={2019},
eprint={1910.06591},
archivePrefix={arXiv},
primaryClass={cs.LG}
}
Pull Requests
At this time, we do not accept pull requests. We are happy to link to forks that add interesting functionality.
Prerequisites
There are a few steps you need to take before playing with SEED. Instructions below assume you run the Ubuntu distribution.
-
Install docker by following instructions at https://docs.docker.com/install/linux/docker-ce/ubuntu/. You need 19.03 version or later due to required GPU support.
-
Make sure docker works as non-root user by following instructions at https://docs.docker.com/install/linux/linux-postinstall, section Manage Docker as a non-root user.
-
Install git:
apt-get install git
- Clone SEED git repository:
git clone https://github.com/google-research/seed_rl.git
cd seed_rl
Local Machine Training on a Single Level
To easily start with SEED we provide a way of running it on a local
machine. You just need to run one of the following commands (adjusting
number of actors
and number of envs. per actor
Env. batch size` to your machine):
./run_local.sh [Game] [Agent] [number of actors] [number of envs. per actor]
./run_local.sh atari r2d2 4 4
./run_local.sh football vtrace 4 1
./run_local.sh dmlab vtrace 4 4
./run_local.sh mujoco ppo 4 32 --gin_config=/seed_rl/mujoco/gin/ppo.gin
It will build a Docker image using SEED source code and start the training inside the Docker image. Note that hyper parameters are not tuned in the runs above. Tensorboard is started as part of the training. It can be viewed under http://localhost:6006 by default.
We also provide a sample script for running training with tuned parameters for
HalfCheetah-v2. This setup runs training with 8x32=256 parallel environments to
make training faster. The sample complexity can be improved at the cost
of slower training by running fewer environments and increasing the
unroll_length
parameter.
./mujoco/local_baseline_HalfCheetah-v2.sh
Distributed Training using AI Platform
Note that training with AI Platform results in charges for using compute resources.
The first step is to configure GCP and a Cloud project you will use for training:
- Install Cloud SDK following instructions at https://cloud.google.com/sdk/install and setup up your GCP project.
- Make sure that billing is enabled for your project.
- Enable the AI Platform ("Cloud Machine Learning Engine") and Compute Engine APIs.
- Grant access to the AI Platform service accounts as described at https://cloud.google.com/ml-engine/docs/working-with-cloud-storage.
- Cloud-authenticate in your shell, so that SEED scripts can use your project:
gcloud auth login
gcloud config set project [YOUR_PROJECT]
Then you just need to execute one of the provided scenarios:
gcp/train_[scenario_name].sh
This will build the Docker image, push it to the repository which AI Platform can access and start the training process on the Cloud. Follow output of the command for progress. You can also view the running training jobs at https://console.cloud.google.com/ml/jobs
DeepMind Lab Level Cache
By default majority of DeepMind Lab's CPU usage is generated by creating new
scenarios. This cost can be eliminated by enabling level cache. To enable it,
set the level_cache_dir
flag in the dmlab/config.py
.
As there are many unique episodes it is a good idea to share the same cache
across multiple experiments.
For AI Platform you can add
--level_cache_dir=gs://${BUCKET_NAME}/dmlab_cache
to the list of parameters passed in gcp/submit.sh
to the experiment.
Baseline data on ATARI-57
We provide baseline training data for SEED's R2D2 trained on ATARI games in the form of training curves (checkpoints and Tensorboard event files coming soon). We provide data for 4 independent seeds run up to 40e9 environment frames.
The hyperparameters and evaluation procedure are the same as in section A.3.1 in the paper.
Training curves
Training curves are available on this page.
Checkpoints and Tensorboard event files
Checkpoints and tensorboard event files can be downloaded individually here or as a single (70GBs) zip file.
Additional links
SEED was used as a core infrastructure piece for the What Matters In On-Policy Reinforcement Learning? A Large-Scale Empirical Study paper. A colab that reproduces plots from the paper can be found here.