Self-Organizing Recurrent Neural Networks
SORN is a class of neuro-inspired artificial network build based on plasticity mechanisms in biological brain and mimic neocortical circuits ability of learning and adaptation. SORN consists of pool of excitatory neurons and small population of inhibitory neurons which are controlled by 5 plasticity mechanisms found in neocortex, namely Spike Timing Dependent Plasticity (STDP), Intrinsic Plasticity (IP), Synaptic Scaling (SS),Synaptic Normalization(SN) and inhibitory Spike Timing Dependent Plasticity (iSTDP). Using mathematical tools, SORN network simplifies the underlying structural and functional connectivity mechanisms responsible for learning and memory in the brain
'sorn' is a Python package designed for Self Organizing Recurrent Neural Networks. It provides a research environment for computational neuroscientists to study the self-organization, adaption, learning,memory and behavior of brain circuits by reverse engineering neural plasticity mechanisms. Further to extend the potential applications of sorn
, a demostrative example of a neuro-robotics experiment using OpenAI gym is also documented.
SORN Reservoir
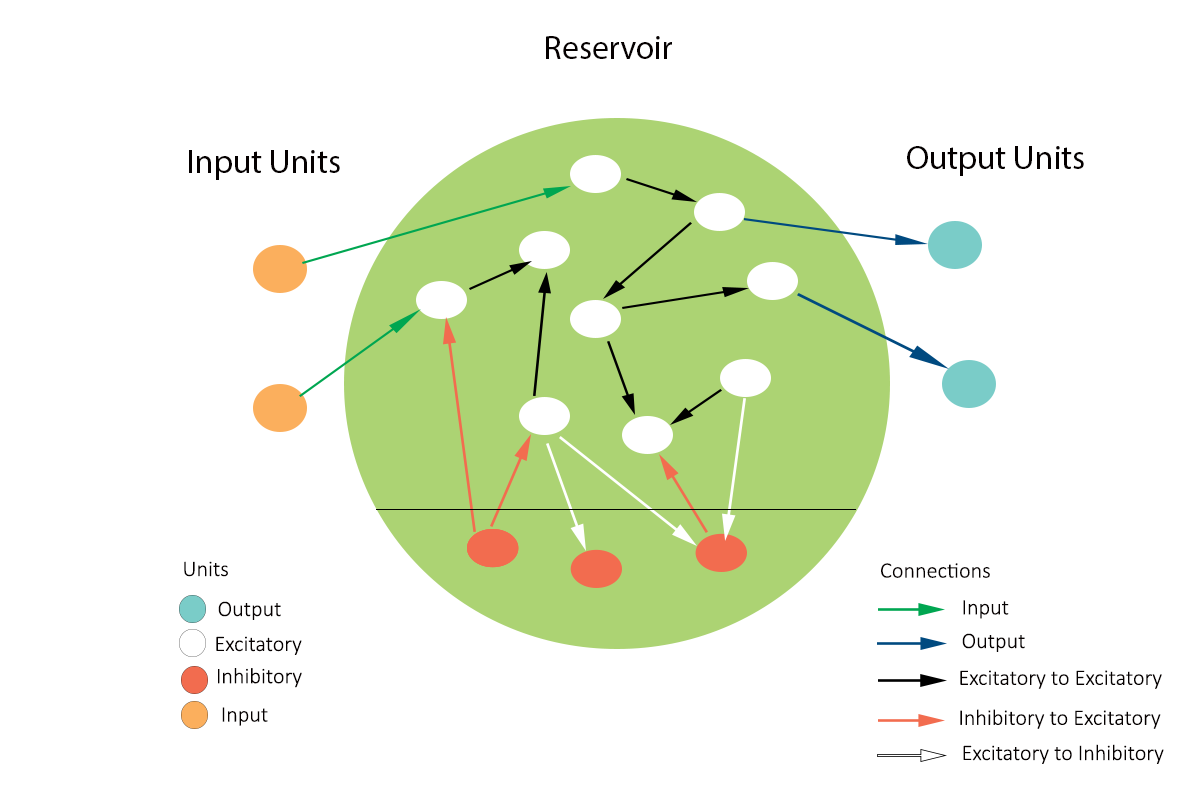
Installation
pip install sorn
or to install the latest version from the development branch
pip install git+https://github.com/Saran-nns/sorn
Dependencies
SORN supports Python 3.7+
ONLY. For older Python versions please use the official Python client.
To install all optional dependencies,
pip install 'sorn[all]'
Usage
Plasticity Phase
import sorn
from sorn import Simulator
import numpy as np
# Sample input
num_features = 10
time_steps = 200
inputs = np.random.rand(num_features,time_steps)
# Simulate the network with default hyperparameters under gaussian white noise
state_dict, sim_dict = Simulator.run(inputs=inputs, phase='plasticity',
state=None, noise=True,
timesteps=time_steps,
callbacks=["ExcitatoryActivation",
"WEE",
"EEConnectionCounts"])
Network Initialized
Number of connections in Wee 3909 , Wei 1574, Wie 8000
Shapes Wee (200, 200) Wei (40, 200) Wie (200, 40)
Training Phase
from sorn import Trainer
# NOTE: During training phase, input to `sorn` should have second (time) dimension set to 1. ie., input shape should be (input_features,1).
inputs = np.random.rand(num_features,1)
# SORN network is frozen during training phase
state_dict, sim_dict = Trainer.run(inputs= inputs, phase='training',
state=state_dict, noise=False,
timesteps=1,
ne=100, nu=num_features,
lambda_ee=10, eta_stdp=0.001,
callbacks=["InhibitoryActivation",
"WEI",
"EIConnectionCounts"] )
Network Output Descriptions
state_dict
- Dictionary of connection weights (Wee
, Wei
, Wie
) , Excitatory network activity (X
), Inhibitory network activities(Y
), Threshold values (Te
, Ti
)
sim_dict
- Dictionary of network states and parameters collected during the simulation/training: Provided, all available options of the argument callbacks
, then the sim_dict
should contain the following;
"ExcitatoryActivation" - Excitatory network activity of entire simulation period
"InhibitoryActivation" - Inhibitory network activity of entire simulation period
"RecurrentActivation" - Recurrent network activity of entire simulation period
"EEConnectionCounts" - Number of active connections in the Excitatory pool at each time step
"EIConnectionCounts" - Number of active connections from Inhibitory to Excitatory pool at each time step
"TE" - Threshold values of excitatory neurons at each time step
"TI" - Threshold values of inhibitory neurons at each time step
"WEE" - Synaptic efficacies between excitatory neurons
"WEI" - Connection weights from inhibitory to excitatory neurons
"WIE" - Connection weights from excitatory to inhibitory neurons
Documentation
For detailed documentation about development, analysis, plotting methods and a sample experiment with OpenAI Gym, please visit SORN Documentation
Citation
@article{Nambusubramaniyan2021,
doi = {10.21105/joss.03545},
url = {https://doi.org/10.21105/joss.03545},
year = {2021},
publisher = {The Open Journal},
volume = {6},
number = {65},
pages = {3545},
author = {Saranraj Nambusubramaniyan},
title = {`sorn`: A Python package for Self Organizing Recurrent Neural Network},
journal = {Journal of Open Source Software}
}
Contributions
I am welcoming contributions. If you wish to contribute, please create a branch with a pull request and the changes can be discussed there. If you find a bug in the code or errors in the documentation, please open a new issue in the Github repository and report the bug or the error. Please provide sufficient information for the bug to be reproduced.