
npm install compromise
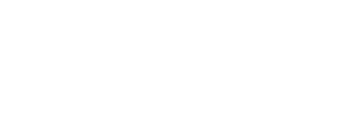
-
↬ᔐᖜ↬- and how we can't get the information back out?⇬
should not really be used.
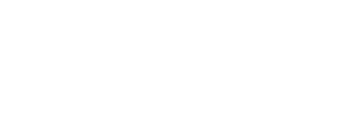
.match():
interpret and match text:
let doc = nlp(entireNovel)
doc.match('the #Adjective of times').text()
// "the blurst of times?"
if (doc.has('simon says #Verb') === false) {
return null
}
.verbs():
conjugate and negate verbs in any tense:
let doc = nlp('she sells seashells by the seashore.')
doc.verbs().toPastTense()
doc.text()
// 'she sold seashells by the seashore.'
.nouns():
play between plural, singular and possessive forms:
let doc = nlp('the purple dinosaur')
doc.nouns().toPlural()
doc.text()
// 'the purple dinosaurs'
.numbers():
interpret plain-text numbers
nlp.extend(require('compromise-numbers'))
let doc = nlp('ninety five thousand and fifty two')
doc.numbers().add(2)
doc.text()
// 'ninety five thousand and fifty four'
.topics():
names/places/orgs, tldr:
let doc = nlp(buddyHolly)
doc.people().if('mary').json()
// [{text:'Mary Tyler Moore'}]
let doc = nlp(freshPrince)
doc.places().first().text()
// 'West Phillidelphia'
doc = nlp('the opera about richard nixon visiting china')
doc.topics().json()
// [
// { text: 'richard nixon' },
// { text: 'china' }
// ]
.contractions():
handle implicit terms:
let doc = nlp("we're not gonna take it, no we ain't gonna take it.")
// match an implicit term
doc.has('going') // true
// transform
doc.contractions().expand()
dox.text()
// 'we are not going to take it, no we are not going to take it.'
Use it on the client-side:
<script src="https://unpkg.com/compromise"></script>
<script src="https://unpkg.com/compromise-numbers"></script>
<script>
nlp.extend(compromiseNumbers)
var doc = nlp('two bottles of beer')
doc.numbers().minus(1)
document.body.innerHTML = doc.text()
// 'one bottle of beer'
</script>
as an es-module:
import nlp from 'compromise'
var doc = nlp('London is calling')
doc.verbs().toNegative()
// 'London is not calling'
compromise is 180kb (minified):
it's pretty fast. It can run on keypress:
it works mainly by conjugating all forms of a basic word list.
The final lexicon is ~14,000 words:
you can read more about how it works, here. it's weird.
.extend():
decide how words get interpreted:
let myWords = {
kermit: 'FirstName',
fozzie: 'FirstName',
}
let doc = nlp(muppetText, myWords)
or make heavier changes with a compromise-plugin.
const nlp = require('compromise')
nlp.extend((Doc, world) => {
// add new tags
world.addTags({
Character: {
isA: 'Person',
notA: 'Adjective',
},
})
// add or change words in the lexicon
world.addWords({
kermit: 'Character',
gonzo: 'Character',
})
// add methods to run after the tagger
world.postProcess(doc => {
doc.match('light the lights').tag('#Verb . #Plural')
})
// add a whole new method
Doc.prototype.kermitVoice = function () {
this.sentences().prepend('well,')
this.match('i [(am|was)]').prepend('um,')
return this
}
})
Docs:
gentle introduction:
Documentation:
Talks:
- Language as an Interface - by Spencer Kelly
- Coding Chat Bots - by KahWee Teng
- On Typing and data - by Spencer Kelly
Articles:
- Geocoding Social Conversations with NLP and JavaScript - by Microsoft
- Microservice Recipe - by Eventn
- Adventure Game Sentence Parsing with Compromise
- Building Text-Based Games - by Matt Eland
- Fun with javascript in BigQuery - by Felipe Hoffa
- Natural Language Processing... in the Browser? - by Charles Landau
Some fun Applications:
- Automated Bechdel Test - by The Guardian
- Story generation framework - by Jose Phrocca
- Tumbler blog of lists - horse-ebooks-like lists - by Michael Paulukonis
- Video Editing from Transcription - by New Theory
- Browser extension Fact-checking - by Alexander Kidd
- Siri shortcut - by Michael Byrns
- Amazon skill - by Tajddin Maghni
- Tasking Slack-bot - by Kevin Suh [see more]
API:
Constructor
(these methods are on the nlp
object)
- .tokenize() - parse text without running POS-tagging
- .extend() - mix in a compromise-plugin
- .fromJSON() - load a compromise object from
.json()
result - .verbose() - log our decision-making for debugging
- .version() - current semver version of the library
- .world() - grab all current linguistic data
- .parseMatch() - pre-parse any match statements for faster lookups
Utils
- .all() - return the whole original document ('zoom out')
- .found [getter] - is this document empty?
- .parent() - return the previous result
- .parents() - return all of the previous results
- .tagger() - (re-)run the part-of-speech tagger on this document
- .wordCount() - count the # of terms in the document
- .length [getter] - count the # of characters in the document (string length)
- .clone() - deep-copy the document, so that no references remain
- .cache({}) - freeze the current state of the document, for speed-purposes
- .uncache() - un-freezes the current state of the document, so it may be transformed
Accessors
- .first(n) - use only the first result(s)
- .last(n) - use only the last result(s)
- .slice(n,n) - grab a subset of the results
- .eq(n) - use only the nth result
- .terms() - split-up results by each individual term
- .firstTerms() - get the first word in each match
- .lastTerms() - get the end word in each match
- .sentences() - get the whole sentence for each match
- .termList() - return a flat list of all Term objects in match
- .groups('') - grab any named capture-groups from a match
Match
(all match methods use the match-syntax.)
- .match('') - return a new Doc, with this one as a parent
- .not('') - return all results except for this
- .matchOne('') - return only the first match
- .if('') - return each current phrase, only if it contains this match ('only')
- .ifNo('') - Filter-out any current phrases that have this match ('notIf')
- .has('') - Return a boolean if this match exists
- .lookBehind('') - search through earlier terms, in the sentence
- .lookAhead('') - search through following terms, in the sentence
- .before('') - return all terms before a match, in each phrase
- .after('') - return all terms after a match, in each phrase
- .lookup([]) - quick find for an array of string matches
Case
- .toLowerCase() - turn every letter of every term to lower-cse
- .toUpperCase() - turn every letter of every term to upper case
- .toTitleCase() - upper-case the first letter of each term
- .toCamelCase() - remove whitespace and title-case each term
Whitespace
- .pre('') - add this punctuation or whitespace before each match
- .post('') - add this punctuation or whitespace after each match
- .trim() - remove start and end whitespace
- .hyphenate() - connect words with hyphen, and remove whitespace
- .dehyphenate() - remove hyphens between words, and set whitespace
- .toQuotations() - add quotation marks around these matches
- .toParentheses() - add brackets around these matches
Tag
- .tag('') - Give all terms the given tag
- .tagSafe('') - Only apply tag to terms if it is consistent with current tags
- .unTag('') - Remove this term from the given terms
- .canBe('') - return only the terms that can be this tag
Loops
- .map(fn) - run each phrase through a function, and create a new document
- .forEach(fn) - run a function on each phrase, as an individual document
- .filter(fn) - return only the phrases that return true
- .find(fn) - return a document with only the first phrase that matches
- .some(fn) - return true or false if there is one matching phrase
- .random(fn) - sample a subset of the results
Insert
- .replace(match, replace) - search and replace match with new content
- .replaceWith(replace) - substitute-in new text
- .delete() - fully remove these terms from the document
- .append(str) - add these new terms to the end (insertAfter)
- .prepend(str) - add these new terms to the front (insertBefore)
- .concat() - add these new things to the end
Transform
- .sort('method') - re-arrange the order of the matches (in place)
- .reverse() - reverse the order of the matches, but not the words
- .normalize({}) - clean-up the text in various ways
- .unique() - remove any duplicate matches
- .split('') - return a Document with three parts for every match ('splitOn')
- .splitBefore('') - partition a phrase before each matching segment
- .splitAfter('') - partition a phrase after each matching segment
- .segment({}) - split a document into labeled sections
- .join('') - make all phrases into one phrase
Output
- .text('method') - return the document as text
- .json({}) - pull out desired metadata from the document
- .out('array|offset|terms') - some named output formats (deprecated)
- .debug() - pretty-print the current document and its tags
Selections
- .clauses() - split-up sentences into multi-term phrases
- .hyphenated() - all terms connected with a hyphen or dash like
'wash-out'
- .phoneNumbers() - things like
'(939) 555-0113'
- .hashTags() - things like
'#nlp'
- .emails() - things like
'[email protected]'
- .emoticons() - things like
:)
- .emojis() - things like
💋
- .atMentions() - things like
'@nlp_compromise'
- .urls() - things like
'compromise.cool'
- .adverbs() - things like
'quickly'
- .pronouns() - things like
'he'
- .conjunctions() - things like
'but'
- .prepositions() - things like
'of'
- .abbreviations() - things like
'Mrs.'
- .people() - names like 'John F. Kennedy'
- .places() - like 'Paris, France'
- .organizations() - like 'Google, Inc'
- .topics() -
people()
+places()
+organizations()
Subsets
- .contractions() - things like "didn't"
- .contractions().expand() - things like "didn't"
- .contract() -
"she would"
->"she'd"
- .parentheses() - return anything inside (parentheses)
- .possessives() - things like
"Spencer's"
- .quotations() - return any terms inside quotation marks
- .acronyms() - things like
'FBI'
- .lists() - things like
'eats, shoots, and leaves'
- .lists().items() - return the partitioned things in the list
- .lists().add() - put a new item in the list
- .nouns() - return any subsequent terms tagged as a Noun
- .nouns().json() - overloaded output with noun metadata
- .nouns().adjectives() - get any adjectives describing this noun
- .nouns().toPlural() -
'football captain' → 'football captains'
- .nouns().toSingular() -
'turnovers' → 'turnover'
- .nouns().isPlural() - return only plural nouns
- .nouns().isSingular() - return only singular nouns
- .nouns().hasPlural() - return only nouns that can be inflected as plural
- .nouns().toPossessive() - add a
's
to the end, in a safe manner.
- .verbs() - return any subsequent terms tagged as a Verb
- .verbs().json() - overloaded output with verb metadata
- .verbs().conjugate() - return all forms of these verbs
- .verbs().toPastTense() -
'will go' → 'went'
- .verbs().toPresentTense() -
'walked' → 'walks'
- .verbs().toFutureTense() -
'walked' → 'will walk'
- .verbs().toInfinitive() -
'walks' → 'walk'
- .verbs().toGerund() -
'walks' → 'walking'
- .verbs().toParticiple() -
'drive' → 'driven'
- otherwise simple-past ('walked') - .verbs().toNegative() -
'went' → 'did not go'
- .verbs().toPositive() -
"didn't study" → 'studied'
- .verbs().isNegative() - return verbs with 'not'
- .verbs().isPositive() - only verbs without 'not'
- .verbs().isPlural() - return plural verbs like 'we walk'
- .verbs().isSingular() - return singular verbs like 'spencer walks'
- .verbs().adverbs() - return the adverbs describing this verb.
- .verbs().isImperative() - only instruction verbs like 'eat it!'
Plugins:
These are some helpful extensions:
Adjectives
npm install compromise-adjectives
- .adjectives() - like
quick
- .adjectives().json() - overloaded output with adjective metadata
- .adjectives().conjugate() - return all conjugated forms of this adjective
- .adjectives().toSuperlative() - convert
quick
toquickest
- .adjectives().toComparative() - convert
quick
toquicker
- .adjectives().toAdverb() - convert
quick
toquickly
- .adjectives().toVerb() - convert
quick
toquicken
- .adjectives().toNoun() - convert
quick
toquickness
Dates
npm install compromise-dates
- .dates() - find dates like
June 8th
or03/03/18
- .dates().get() - simple start/end json result
- .dates().json() - overloaded output with date metadata
- .dates().format('') - convert the dates to specific formats
- .dates().toShortForm() - convert 'Wednesday' to 'Wed', etc
- .dates().toLongForm() - convert 'Feb' to 'February', etc
- .durations() -
2 weeks
or5mins
- .durations().get() - return simple json for duration
- .durations().json() - overloaded output with duration metadata
- .times() -
4:30pm
orhalf past five
- .durations().get() - return simple json for times
- .times().json() - overloaded output with time metadata
Numbers
npm install compromise-numbers
- .numbers() - grab all written and numeric values
- .numbers().get() - retrieve the parsed number(s)
- .numbers().json() - overloaded output with number metadata
- .numbers().units() - grab 'kilos' from
25 kilos'
- .numbers().fractions() - things like
1/3rd
- .numbers().toText() - convert number to
five
orfifth
- .numbers().toNumber() - convert number to
5
or5th
- .numbers().toOrdinal() - convert number to
fifth
or5th
- .numbers().toCardinal() - convert number to
five
or5
- .numbers().set(n) - set number to n
- .numbers().add(n) - increase number by n
- .numbers().subtract(n) - decrease number by n
- .numbers().increment() - increase number by 1
- .numbers().decrement() - decrease number by 1
- .numbers().isEqual(n) - return numbers with this value
- .numbers().greaterThan(min) - return numbers bigger than n
- .numbers().lessThan(max) - return numbers smaller than n
- .numbers().between(min, max) - return numbers between min and max
- .numbers().isOrdinal() - return only ordinal numbers
- .numbers().isCardinal() - return only cardinal numbers
- .numbers().toLocaleString() - add commas, or nicer formatting for numbers
- .money() - things like
'$2.50'
- .money().get() - retrieve the parsed amount(s) of money
- .money().json() - currency + number info
- .money().currency() - which currency the money is in
- .fractions() - like '2/3rds' or 'one out of five'
- .fractions().get() - simple numerator, denomenator data
- .fractions().json() - json method overloaded with fractions data
- .fractions().toDecimal() - '2/3' -> '0.66'
- .fractions().normalize() - 'four out of 10' -> '4/10'
- .fractions().toText() - '4/10' -> 'four tenths'
- .fractions().toPercentage() - '4/10' -> '40%'
- .percentages() - like '2.5%'
- .fractions().get() - return the percentage number / 100
- .fractions().json() - json overloaded with percentage information
- .fractions().toFraction() - '80%' -> '8/10'
Export
npm install compromise-export
- .export() - store a parsed document for later use
- nlp.load() - re-generate a Doc object from .export() results
Html
npm install compromise-html
- .html({}) - generate sanitized html from the document
Hash
npm install compromise-hash
- .hash() - generate an md5 hash from the document+tags
- .isEqual(doc) - compare the hash of two documents for semantic-equality
Keypress
npm install compromise-keypress
- nlp.keypress('') - generate an md5 hash from the document+tags
- nlp.clear('') - clean-up any cached sentences from memory
Ngrams
npm install compromise-ngrams
- .ngrams({}) - list all repeating sub-phrases, by word-count
- .unigrams() - n-grams with one word
- .bigrams() - n-grams with two words
- .trigrams() - n-grams with three words
- .startgrams() - n-grams including the first term of a phrase
- .endgrams() - n-grams including the last term of a phrase
- .edgegrams() - n-grams including the first or last term of a phrase
Paragraphs
npm install compromise-paragraphs
this plugin creates a wrapper around the default sentence objects.
- .paragraphs() - return groups of sentences
- .paragraphs().json() - output metadata for each paragraph
- .paragraphs().sentences() - go back to a regular Doc object
- .paragraphs().terms() - return all individual terms
- .paragraphs().eq() - get the nth paragraph
- .paragraphs().first() - get the first n paragraphs
- .paragraphs().last() - get the last n paragraphs
- .paragraphs().match() -
- .paragraphs().not() -
- .paragraphs().if() -
- .paragraphs().ifNo() -
- .paragraphs().has() -
- .paragraphs().forEach() -
- .paragraphs().map() -
- .paragraphs().filter() -
Sentences
npm install compromise-sentences
- .sentences() - return a sentence class with additional methods
- .sentences().json() - overloaded output with sentence metadata
- .sentences().subjects() - return the main noun of each sentence
- .sentences().toPastTense() -
he walks
->he walked
- .sentences().toPresentTense() -
he walked
->he walks
- .sentences().toFutureTense() --
he walks
->he will walk
- .sentences().toNegative() - -
he walks
->he didn't walk
- .sentences().toPositive() -
he doesn't walk
->he walks
- .sentences().isPassive() - return only sentences with a passive-voice
- .sentences().isQuestion() - return questions with a
?
- .sentences().isExclamation() - return sentences with a
!
- .sentences().isStatement() - return sentences without
?
or!
- .sentences().prepend() - smarter prepend that repairs whitespace + titlecasing
- .sentences().append() - smarter append that repairs sentence punctuation
- .sentences().toExclamation() - end sentence with a
!
- .sentences().toQuestion() - end sentence with a
?
- .sentences().toStatement() - end sentence with a
.
Strict-match
npm install compromise-strict
- .strictMatch() - perform a compromise match using a formal parser
Syllables
npm install compromise-syllables
- .syllables() - split each term by its typical pronounciation
Penn-tags
npm install compromise-penn-tags
- .pennTags() - return POS tags from the Penn Tagset
Typescript
we're committed to typescript/deno support, both in main and in the official-plugins:
import nlp from 'compromise'
import ngrams from 'compromise-ngrams'
import numbers from 'compromise-numbers'
const nlpEx = nlp.extend(ngrams).extend(numbers)
nlpEx('This is type safe!').ngrams({ min: 1 })
nlpEx('This is type safe!').numbers()
Partial-builds
or if you don't care about POS-tagging, you can use the tokenize-only build: (90kb!)
<script src="https://unpkg.com/compromise/builds/compromise-tokenize.js"></script>
<script>
var doc = nlp('No, my son is also named Bort.')
//you can see the text has no tags
console.log(doc.has('#Noun')) //false
//the rest of the api still works
console.log(doc.has('my .* is .? named /^b[oa]rt/')) //true
</script>
Limitations:
-
slash-support: We currently split slashes up as different words, like we do for hyphens. so things like this don't work:
nlp('the koala eats/shoots/leaves').has('koala leaves') //false
-
inter-sentence match: By default, sentences are the top-level abstraction. Inter-sentence, or multi-sentence matches aren't supported without a plugin:
nlp("that's it. Back to Winnipeg!").has('it back')//false
-
nested match syntax: the
dangerbeauty of regex is that you can recurse indefinitely. Our match syntax is much weaker. Things like this are not (yet) possible:doc.match('(modern (major|minor))? general')
complex matches must be achieved with successive .match() statements. -
dependency parsing: Proper sentence transformation requires understanding the syntax tree of a sentence, which we don't currently do. We should! Help wanted with this.
FAQ
☂️ Isn't javascript too...
💃 Can it run on my arduino-watch?
-
Only if it's water-proof!
Read quick start for running compromise in workers, mobile apps, and all sorts of funny environments.
🌎 Compromise in other Languages?
✨ Partial builds?
-
we do offer a compromise-tokenize build, which has the POS-tagger pulled-out.
but otherwise, compromise isn't easily tree-shaken.
the tagging methods are competitive, and greedy, so it's not recommended to pull things out.
Note that without a full POS-tagging, the contraction-parser won't work perfectly. ((spencer's cool) vs. (spencer's house))
It's recommended to run the library fully.
See Also:
-
en-pos - very clever javascript pos-tagger by Alex Corvi
-
naturalNode - fancier statistical nlp in javascript
-
compendium-js - POS and sentiment analysis in javascript
-
nodeBox linguistics - conjugation, inflection in javascript
-
reText - very impressive text utilities in javascript
-
superScript - conversation engine in js
-
jsPos - javascript build of the time-tested Brill-tagger
-
spaCy - speedy, multilingual tagger in C/python
-
Prose - quick tagger in Go by Joseph Kato
MIT