JWarmenhoven / Dbda Python
Projects that are alternatives of or similar to Dbda Python
Doing Bayesian Data Analysis - Python/PyMC3
This repository contains Python/PyMC3 code for a selection of models and figures from the book 'Doing Bayesian Data Analysis: A Tutorial with R, JAGS, and Stan', Second Edition, by John Kruschke (2015). The datasets used in this repository have been retrieved from the book's website. Note that, in its current form, this repository is not a standalone tutorial and that you probably should have a copy of the book to follow along. Suggestions for improvement and help with unsolved issues are welcome!
Note that the code is in Jupyter Notebook format and requires modification to use with other datasets.
Some of the general concepts from the book are discussed in papers by Kruschke & Liddell. See references below.
2018-08-16:
Updating the notebooks with PyMC3 v3.5 and general code clean-up. Inserting plots of the PyMC models in plate notation (v3.5 feature). Fixing some deprecation warnings.
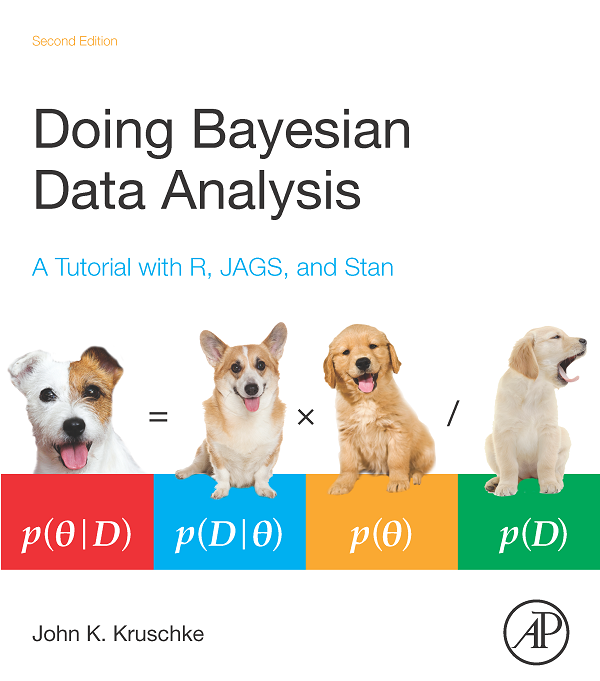
Chapter 9 - Hierarchical Models
Chapter 10 - Model Comparison and Hierarchical Modelling
Chapter 12 - Bayesian Approaches to Testing a Point ("Null") Hypothesis
Chapter 16 - Metric-Predicted Variable on One or Two Groups
Chapter 17 - Metric-Predicted Variable with One Metric Predictor
Chapter 18 - Metric Predicted Variable with Multiple Metric Predictors
Chapter 19 - Metric Predicted Variable with One Nominal Predictor
Chapter 20 - Metric Predicted Variable with Multiple Nominal Predictor
Chapter 21 - Dichotomous Predicted Variable
Chapter 22 - Nominal Predicted Variable
Chapter 23 - Ordinal Predicted Variable
Chapter 24 - Count Predicted Variable
Extra:
Bayesian Linear Regression example (Bishop, 2006)
Example on modelling Ordinal Data (Liddell & Kruschke, 2018)
Libraries used:
- pymc3
- theano
- pandas
- numpy
- scipy
- matplotlib
- seaborn
References:
Bishop, C.M. (2006), Pattern Recognition and Machine Learning, Springer Science+Business Media, New York. https://www.microsoft.com/en-us/research/people/cmbishop/
Kruschke, J.K. (2015), Doing Bayesian Data Analysis: A Tutorial with R, JAGS, and Stan, Second Edition, Academic Press / Elsevier, https://sites.google.com/site/doingbayesiandataanalysis/
Kruschke, J.K. & Liddell, T.M. (2017), The Bayesian New Statistics: Hypothesis testing, estimation, meta-analysis, and power analysis from a Bayesian perspective, Psychonomic Bulletin & Review, http://dx.doi.org/10.3758/s13423-016-1221-4
Kruschke, J.K. & Liddell, T.M. (2017), Bayesian data analysis for newcomers, Psychonomic Bulletin & Review, http://dx.doi.org/10.3758/s13423-017-1272-1
Liddell, T., & Kruschke, J. K. (2018, April 5). Analyzing ordinal data with metric models: What could possibly go wrong? Retrieved from http://osf.io/3tkz4
Salvatier J, Wiecki TV, Fonnesbeck C. (2016), Probabilistic programming in Python using PyMC3, PeerJ Computer Science 2:e55, https://doi.org/10.7717/peerj-cs.55
PyMC3 - http://pymc-devs.github.io/pymc3/
Note:
The repository below contains python code for the first edition of the book. The code in that repository is a much more direct implementation of the R/JAGS code from the book than you will find here.
https://github.com/aloctavodia/Doing_bayesian_data_analysis