rui1996 / Deraindrop
Programming Languages
Labels
Projects that are alternatives of or similar to Deraindrop
Attentive Generative Adversarial Network for Raindrop Removal from A Single Image (CVPR'2018)
Rui Qian, Robby T.Tan, Wenhan Yang, Jiajun Su and Jiaying Liu
[Paper Link] [Project Page] [Slides](TBA)[Video](TBA) (CVPR'18 Spotlight)
Abstract
Raindrops adhered to a glass window or camera lens can severely hamper the visibility of a background scene and degrade an image considerably. In this paper, we address the problem by visually removing raindrops, and thus transforming a raindrop degraded image into a clean one. The problem is intractable, since first the regions occluded by raindrops are not given. Second, the information about the background scene of the occluded regions is completely lost for most part. To resolve the problem, we apply an attentive generative network using adversarial training. Our main idea is to inject visual attention into both the generative and discriminative networks. During the training, our visual attention learns about raindrop regions and their surroundings. Hence, by injecting this information, the generative network will pay more attention to the raindrop regions and the surrounding structures, and the discriminative network will be able to assess the local consistency of the restored regions. Apart from raindrop removal, this injection of visual attention to both generative and discriminative networks is another contribution of this paper. Our experiments show the effectiveness of our approach, which outperforms the state of the art methods quantitatively and qualitatively.
If you find the resource useful, please cite the following :- )
@InProceedings{Qian_2018_CVPR,
author = {Qian, Rui and Tan, Robby T. and Yang, Wenhan and Su, Jiajun and Liu, Jiaying},
title = {Attentive Generative Adversarial Network for Raindrop Removal From a Single Image},
booktitle = {The IEEE Conference on Computer Vision and Pattern Recognition (CVPR)},
month = {June},
year = {2018}
}
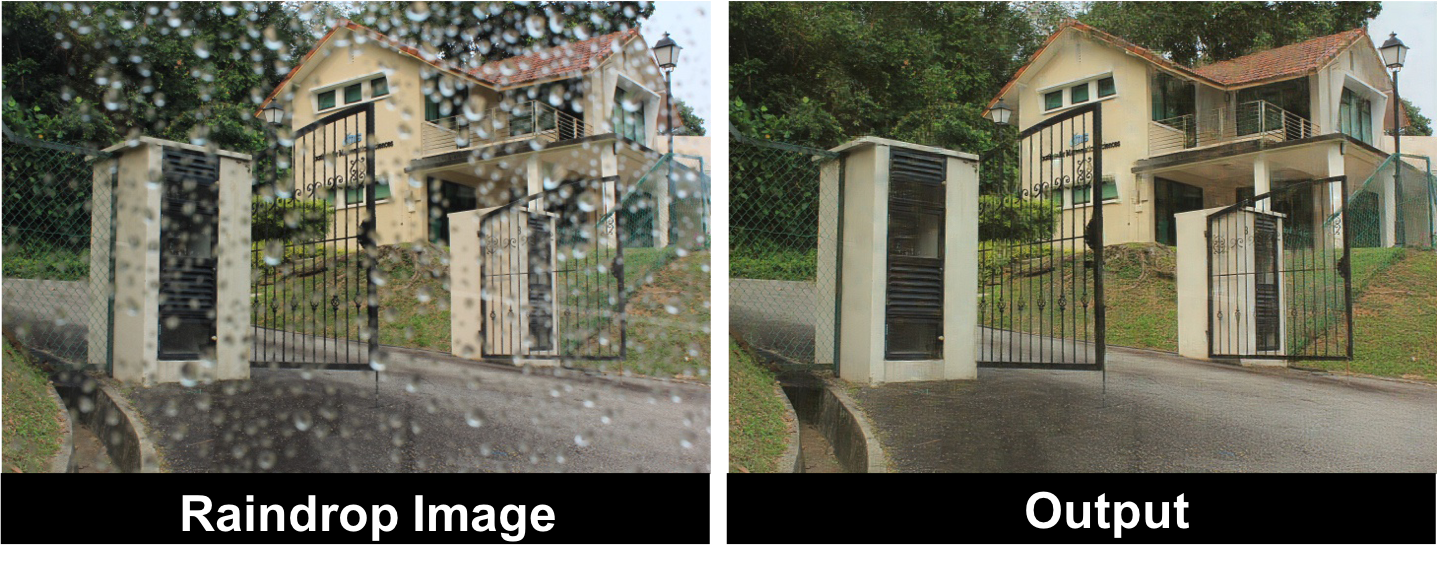
Prerequisites:
- Linux
- Python 2.7
- NVIDIA GPU + CUDA CuDNN (CUDA 8.0)
Installation:
- Clone this repo
- Install PyTorch and dependencies from http://pytorch.org
(Note: the code is suitable for PyTorch 0.3.1)
Demo
The demo pictures are put under the directory ./demo/input/
and ./demo/output/
is a sample of the ouput of the model. If you want to generate your own, use the following code:
CUDA_VISIBLE_DEVICES=gpu_id python predict.py --mode demo --input_dir ./demo/input/ --output_dir ./demo/output/
Dataset
The whole dataset can be find here:
https://drive.google.com/open?id=1e7R76s6vwUJxILOcAsthgDLPSnOrQ49K
####Training Set:
861 image pairs for training.
####Testing Set A:
For quantitative evaluation where the alignment of image pairs is good. A subset of testing set B.
####Testing Set B:
239 image pairs for testing.
Testing
For quantitative evaluation:
Put the test_a dataset under the DeRaindrop directory, and run:
CUDA_VISIBLE_DEVICES=gpu_id python predict.py --mode test --input_dir ./test_a/data/ --gt_dir ./test_a/gt/
Note: Due to the mask of some information such as vehicle license plate number, the PSNR
drop a little (0.06) compared to the result in the paper.
For qualitative evaluation:
Just similar to the demo. Just change the input directory and output directory as you like.
Contact
If you have questions, you can contact [email protected]
.