Huangying-Zhan / Df Vo
Programming Languages
Labels
Projects that are alternatives of or similar to Df Vo
Introduction
This repo implements the system described in the ICRA-2020 paper and the extended report:
Visual Odometry Revisited: What Should Be Learnt?
DF-VO: What Should Be Learnt for Visual Odometry?
Huangying Zhan, Chamara Saroj Weerasekera, Jiawang Bian, Ravi Garg, Ian Reid
The demo video can be found here.
@INPROCEEDINGS{zhan2019dfvo,
author={H. {Zhan} and C. S. {Weerasekera} and J. -W. {Bian} and I. {Reid}},
booktitle={2020 IEEE International Conference on Robotics and Automation (ICRA)},
title={Visual Odometry Revisited: What Should Be Learnt?},
year={2020},
volume={},
number={},
pages={4203-4210},
doi={10.1109/ICRA40945.2020.9197374}}
@misc{zhan2021dfvo,
title={DF-VO: What Should Be Learnt for Visual Odometry?},
author={Huangying Zhan and Chamara Saroj Weerasekera and Jia-Wang Bian and Ravi Garg and Ian Reid},
year={2021},
eprint={2103.00933},
archivePrefix={arXiv},
primaryClass={cs.CV}
}
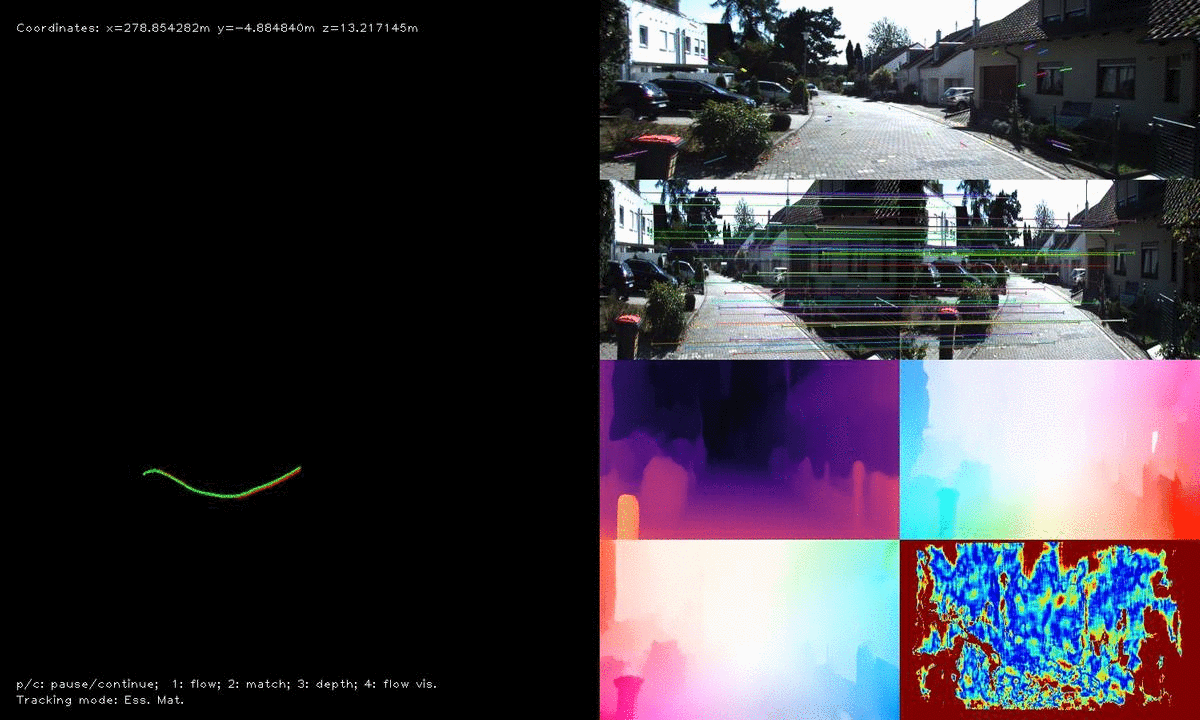
This repo includes
- the frame-to-frame tracking system DF-VO;
- evaluation scripts for visual odometry;
- trained models and VO results
Contents
Part 1. Requirements
This code was tested with Python 3.6, CUDA 9.0, Ubuntu 16.04, and PyTorch-1.1.
We suggest use Anaconda for installing the prerequisites.
cd envs
conda env create -f requirement.yml -p {ANACONDA_DIR/envs/dfvo} # install prerequisites
conda activate dfvo # activate the environment [dfvo]
Part 2. Download dataset and models
The main dataset used in this project is KITTI Driving Dataset. After downloaing the dataset, create a softlink in the current repo.
ln -s KITTI_ODOMETRY/sequences dataset/kitti_odom/odom_data
For our trained models, please visit here to download the models and save the models into the directory model_zoo/
.
Part 3. DF-VO
We have created some examples for running the algorithm.
# Example 1: run default kitti setup
python apis/run.py -d options/examples/default_configuration.yml
# Example 2: Run custom kitti setup
python apis/run.py \
-d options/examples/default_configuration.yml \
-c options/examples/kitti_stereo_train_icra.yml \
--no_confirm
# More examples and our experiments can be found in scripts/experiment.sh
The result (trajectory pose file) is saved in result_dir
defined in the configuration file.
Please check the options/examples/default_configuration.yml
or Configuration Documentation for reference.
Part 4. Result evaluation
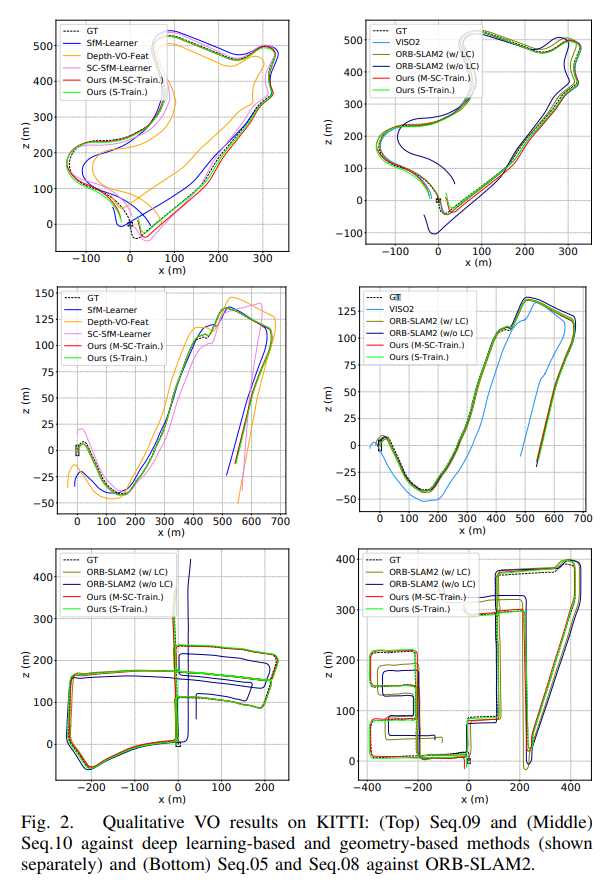
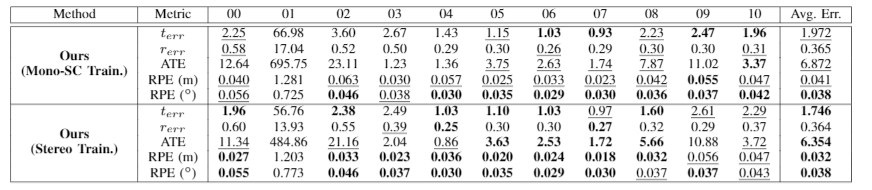
The original results, including related works, can be found here.
KITTI
KITTI Odometry benchmark contains 22 stereo sequences, in which 11 sequences are provided with ground truth. The 11 sequences are used for evaluating visual odometry.
python tools/evaluation/odometry/eval_odom.py --result result/tmp/0 --align 6dof
For more information about the evaluation toolkit, please check the toolbox page or the wiki page.
Part 5. Run your own dataset
We also provide a guideline to run DF-VO on your own dataset. Please check Run own dataset for more details.
License
For academic usage, the code is released under the permissive MIT license. Our intension of sharing the project is for research/personal purpose. For any commercial purpose, please contact the authors.
Acknowledgement
Some of the codes were borrowed from the excellent works of monodepth2, LiteFlowNet and pytorch-liteflownet. The borrowed files are licensed under their original license respectively.