akanimax / Fagan
Programming Languages
Projects that are alternatives of or similar to Fagan
fagan
A variant of the Self Attention GAN named: FAGAN (Full Attention GAN). The architecture of this gan contains the full attention layer as proposed in this project.
The project uses package named attn-gan-pytorch
created by me,
which is available at https://pypi.org/project/attn-gan-pytorch/ .
Celeba samples

samples generated during training of the proposed architecture on the celeba dataset.
Full attention layer
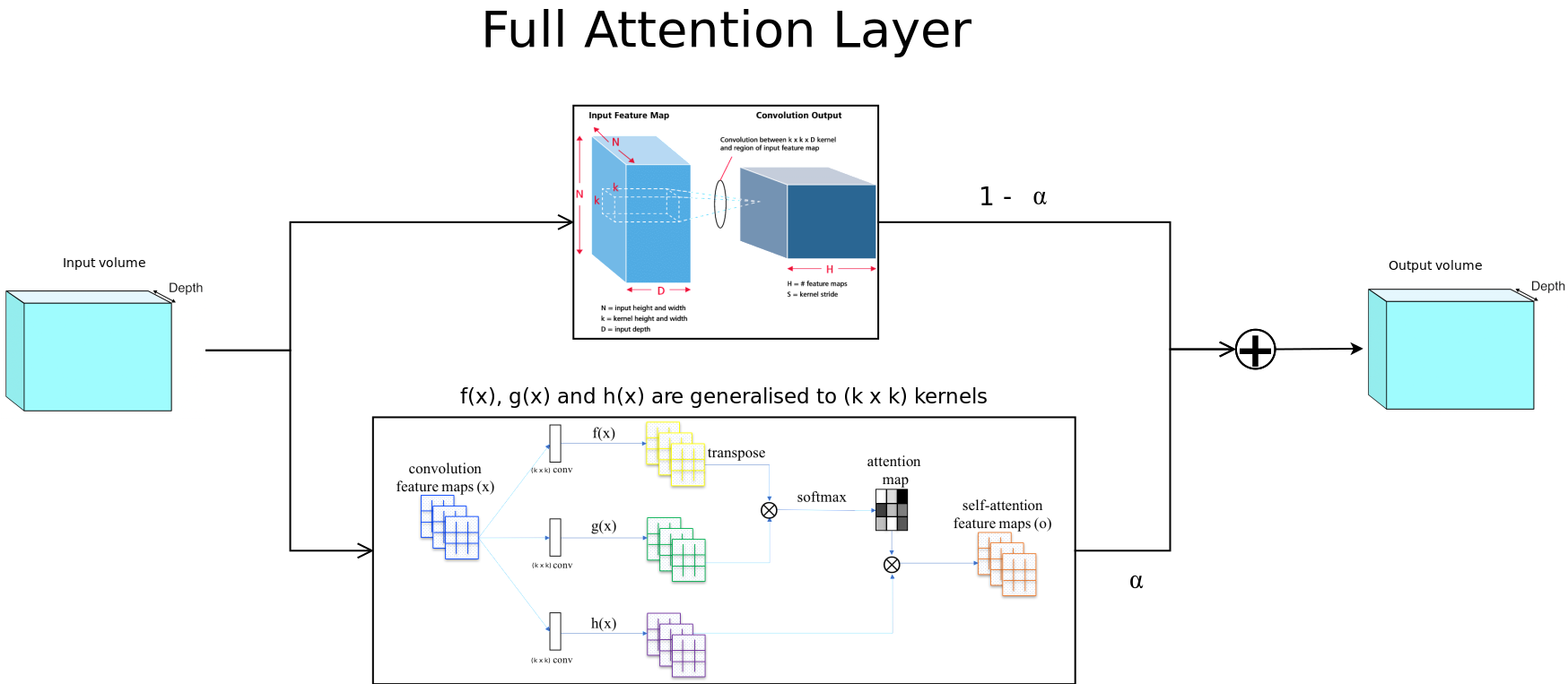
The above figure describes the architecture of the proposed full attention layer. As you can see, on the upper path we compute traditional convolution output and the lower path, we have an attention layer which generalises to (k x k) convolution filters instead of just (1 x 1) filters. The alpha shown in the residual calculation is a trainable parameter.
Now why is the lower path not self attention? The reason for it is that while computing the attention maps, the input is first locally aggregated by the (k x k) convolutions, and therefore is no longer just self attention since it uses a small spatially neighbouring area into computations. Given enough depth and filter size, we could cover the entire input image as a receptive field for a subsequent attention calculation, hence the name: Full Attention.
Celeba Experiment
Hinge-Gan loss experiment
The following diagram is the plot of the loss (Hinge-GAN) generated from the loss-logs obtained during training.
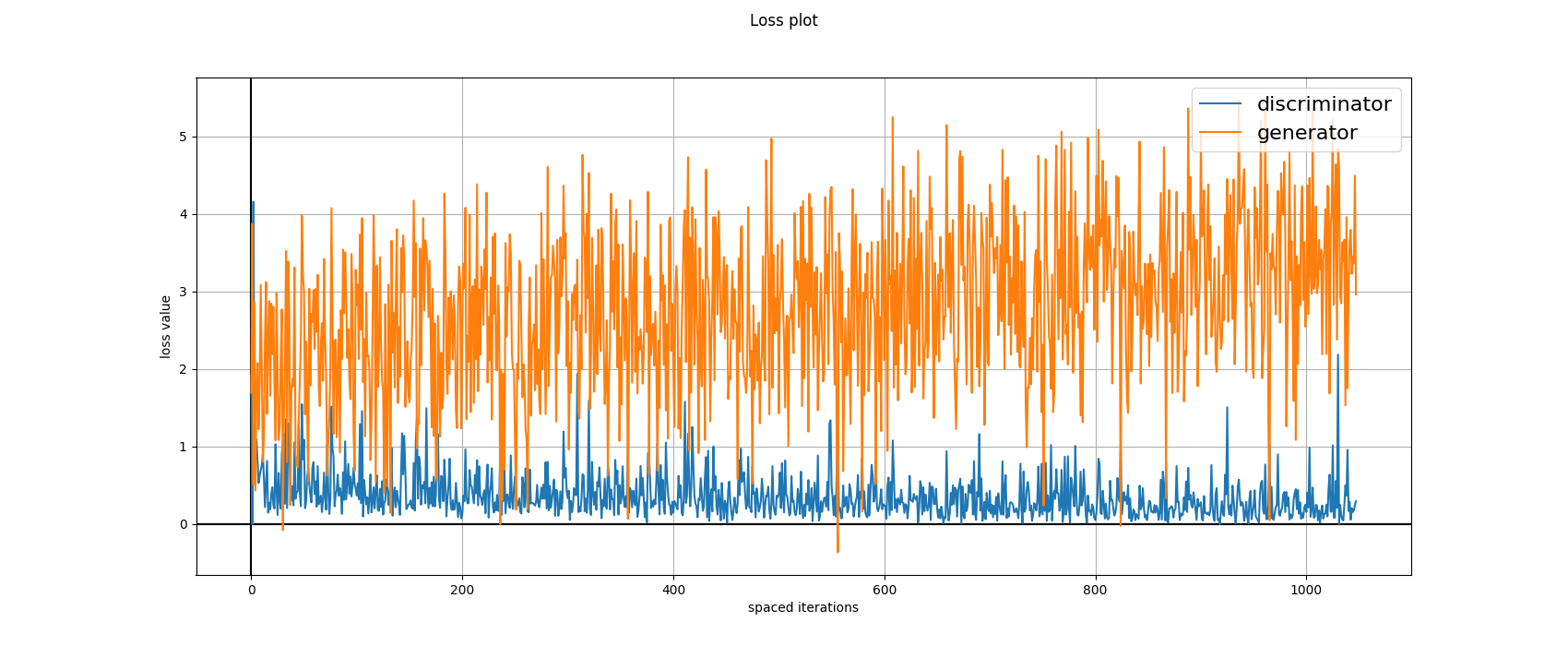
Relativistic Hinge-Gan loss experiment
The training of the relativistic version is percetually better (stabler).
Please refer to the following loss_plot for this experiment for more info.
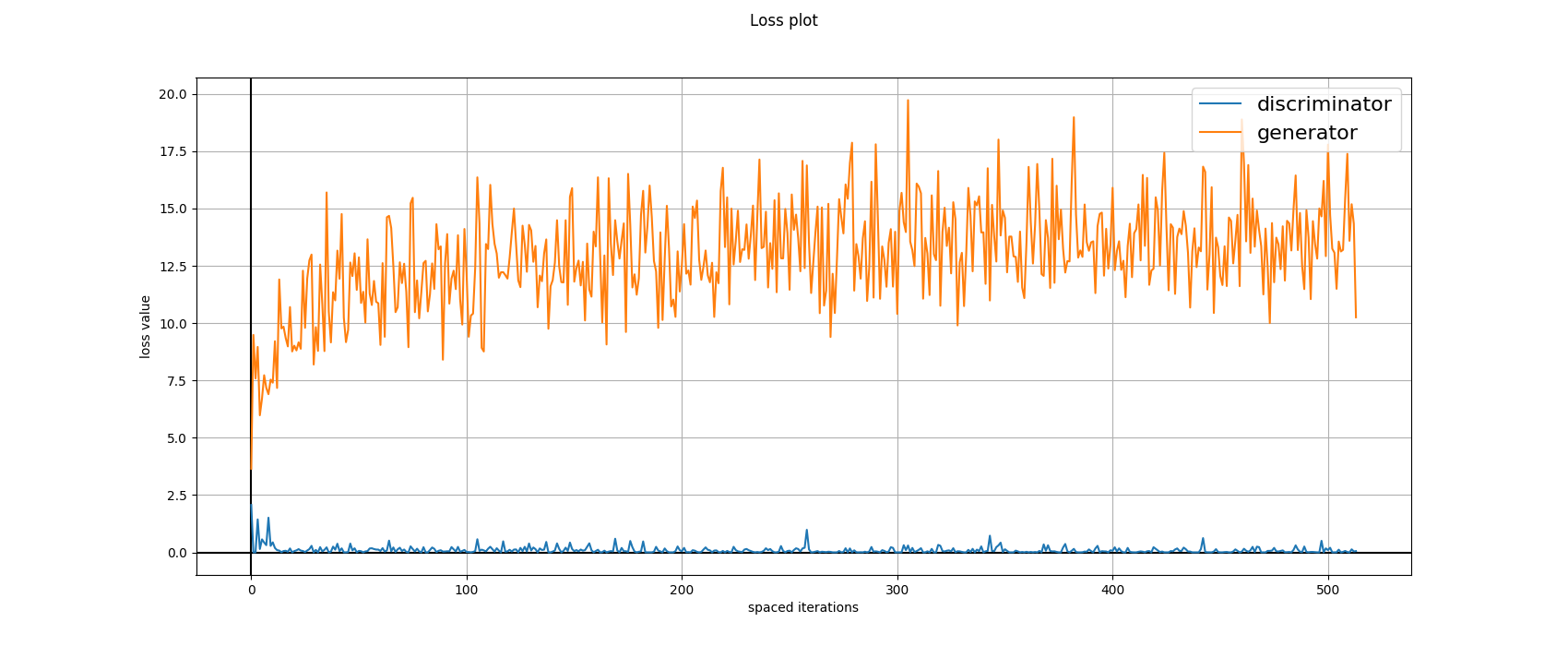
Running the Code
Running the training is actually very simple.
Just install the attn_gan_pytorch
package using the following command
$ workon [your virtual environment]
$ pip install attn-gan-pytorch
And then run the training by running the train.py
script. Refer to
the following parameters for tweaking for your own use:
optional arguments:
-h, --help show this help message and exit
--generator_config GENERATOR_CONFIG
default configuration for generator network
--discriminator_config DISCRIMINATOR_CONFIG
default configuration for discriminator network
--generator_file GENERATOR_FILE
pretrained weights file for generator
--discriminator_file DISCRIMINATOR_FILE
pretrained_weights file for discriminator
--images_dir IMAGES_DIR
path for the images directory
--sample_dir SAMPLE_DIR
path for the generated samples directory
--model_dir MODEL_DIR
path for saved models directory
--latent_size LATENT_SIZE
latent size for the generator
--batch_size BATCH_SIZE
batch_size for training
--start START starting epoch number
--num_epochs NUM_EPOCHS
number of epochs for training
--feedback_factor FEEDBACK_FACTOR
number of logs to generate per epoch
--checkpoint_factor CHECKPOINT_FACTOR
save model per n epochs
--g_lr G_LR learning rate for generator
--d_lr D_LR learning rate for discriminator
--data_percentage DATA_PERCENTAGE
percentage of data to use
--num_workers NUM_WORKERS
number of parallel workers for reading files
Trained weights for generating cool faces :)
refer to the models/fagan_1/
directory to find the saved weights for
this model in pytorch format. For spawning the architectures,
refer to the configs/
folder for loading the generator
and discriminator
configurations.
Other links
medium blog -> https://medium.com/@animeshsk3/fagan-full-attention-gan-2a29227dc014
attn_gan_pytorch package -> https://pypi.org/project/attn-gan-pytorch/
Thanks
Please feel free to open PRs here if
you train on other datasets using this package.
Best regards,
@akanimax :)