gsurma / Text_predictor
Programming Languages
Projects that are alternatives of or similar to Text predictor
Text Predictor
Character-level RNN (Recurrent Neural Net) LSTM (Long Short-Term Memory) implemented in Python 2.7/TensorFlow in order to predict a text based on a given dataset.
Check out corresponding Medium article:
Text Predictor - Generating Rap Lyrics with Recurrent Neural Networks (LSTMs)đ
Heavily influenced by: http://karpathy.github.io/2015/05/21/rnn-effectiveness/.
Idea
- Train RNN LSTM on a given dataset (.txt file).
- Predict text based on a trained model.
Datasets
kanye - Kanye West's discography (332 KB)
darwin - the complete works of Charles Darwin (20 MB)
reuters - a collection of Reuters headlines (95 MB)
war_and_peace - Leo Tolstoy's War and Peace novel (3 MB)
wikipedia - excerpt from English Wikipedia (48 MB)
hackernews - a collection of Hackernews headlines (90 KB)
sherlock - a collection of books with Sherlock Holmes (3 MB)
shakespeare - the complete works of William Shakespeare (4 MB)
tagore - short stories by Rabindranath Tagore (2.6 MB)
Feel free to add new datasets. Just create a folder in the ./data
directory and put an input.txt
file there. Output file along with the training plot will be automatically generated there.
Usage
- Clone the repo.
- Go to the project's root folder.
- Install required packages
pip install -r requirements.txt
. -
python text_predictor.py <dataset>
.
Results
Each dataset were trained with the same hyperparameters.
Hyperparameters
BATCH_SIZE = 32
SEQUENCE_LENGTH = 50
LEARNING_RATE = 0.01
DECAY_RATE = 0.97
HIDDEN_LAYER_SIZE = 256
CELLS_SIZE = 2
Sherlock
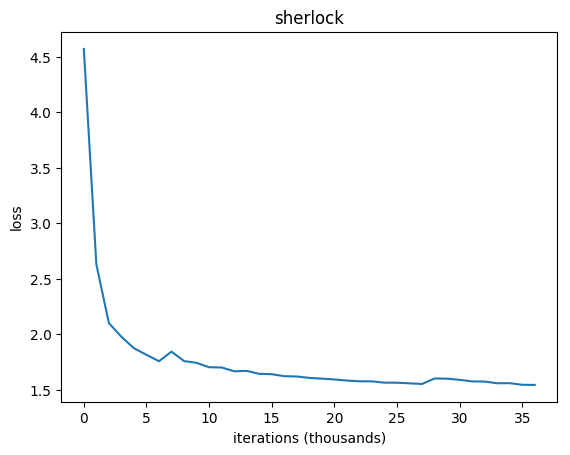
Iteration: 0
l ÃŠÂ°ÂŖI." r, iEgPylXyg
m .iÃŧTÃģ Ccy2M]zTÃĸ. sSRMÂŖt Ê5 âÃŽRlT QAlY4Kv"Ê)kPÂŖStr5/lQVu )Pe0/;s8leJ.ÂŖm40tÃŽJÃŽwB`0]ÂŊjyÃģA`BJi'omNxÂŊ2zG iH:gqri76b&g)ie18PMÂŖvA7pÃKÃĸNQ6
2 Ãģ?]wgÂŖJo4qCde,â.'G,h &wIUaDuÃŽxq`cqb!kf5yB
Iteration: 500
"Other. I
unwallfore of his had Sommopilor out he hase you thed I it.
Book into here, but I told at ht it something do was sack knet afminture-ly. We moke, do oR before drinessast farm. I
Iteration: 1000
some to see me tignaius
rely."
There that you'd them were from I
should not have any take an watchate save now out," said Hodden?"
"Th, a lott remarks. Showed."
"A joan?"
Iteration: 100000
Then mention.""Quite
I gather is stillar in silence was written on the whom I reward an
details grieves of his east back. The week shook this strength.
There was no mystery for y
Hackernews
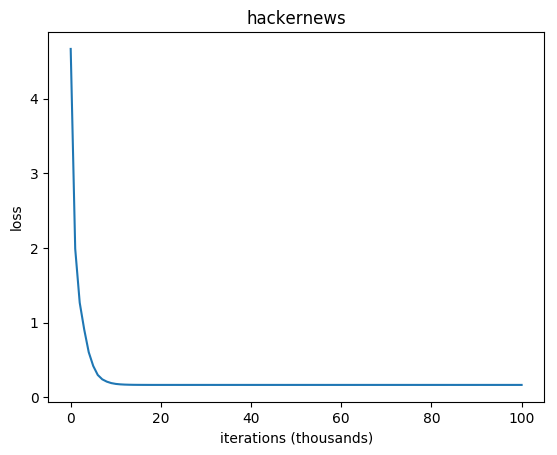
Iteration: 0
%âl~E4*1[â˛)j&â&T$bâ]u:âĻâ.2WPUlFLu*)EÂĩk`qbâŦ[QoE'aLesPâU4.q
o_Z2ZPGÊâMIn8beXSB=BâdNuyâĻuÂĩ20P8vLâ(#
-`H/âŦâŦ:âmÂĩ,g+WU5'^cA=Yât
z+.I,â6N7?f;7Z)nk
iâ ?YsW"iHJ77âŦTyây_eS5pnwN6â
%oVhkXr[xAlc*TxâS1âJ1LlHN'SuHEsiH
Iteration: 500
us codhy in N. DeveloLenic pare abouts scax
Microsign Sy Scodwars
Machons Startians: The is abandied
Payer Progroads Procinters
How 1.05)
Trase elacts Macasications Data Freit Paily trigha bourni
Iteration: 1000
MP
Tx-: IPGS
Primina
Weype
Begal Cd for for was curre hail deselliash your lapthim
Track.L
Tvist
Ubunts writing the like review
Swifch, Now internet will Net 10 TS some libission
Lass and dom
Iteration: 100000
More Than 10 Years Old Available for Free Lens
Teshmeration order Google Vision New NSA targets (2016)
Shot-sizzations of catV; Google - Way Domer Sacks Not Auto-accounts
Amit Gupta needs is moving
Shakespeare
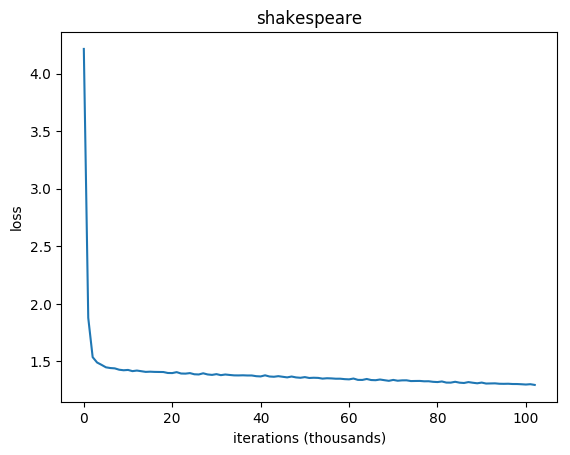
Iteration: 0
TfzVRzdYlDehaDHIhzEiZ&,3knZtHJD]kBOFCpWH.wkWCDVHAK;JcoOMpHJtVNvpcrRSZ,hccUNQ EyG -kpEuvR;MW[JWm;EWv]Au!]EIriywVeGYdljvLkoFMRdikQV:AyoSij.M.;R'lK
vdtnVkxtzL!'qtW$emHfStGUOoK;LJ h
LSyL ?P$KET Z?muR$reIB
Iteration: 500
ticlother them his steaks? whom father-ple plaise't!
HORATIO:
GLOILUS:
Le wime heast,
'Tind soul a bear if thy Gulithes? Preshing;
In beto that mad his says,
Bock Presrike this pray morrombage wenly
Iteration: 1000
HENI:
If which fout in must likest part sors and merr'd?
E sin even and mel full and gooder?
BRUTUS:
Heno Egison to a puenbiloot vieter.
DROMIO OF SYRACUSE:
That is
never standshruced meledder morng
Iteration: 100000
Be feast, tent?
LYSANDER:
And thou love so kiss, to dipate.
All Cornasiers of Atheniansiage are to my sake; but where in end.
APEMANTUS:
Did such a pays. Go, we'll proof.
BERTRAM:
I am reason'dst
War and Peace
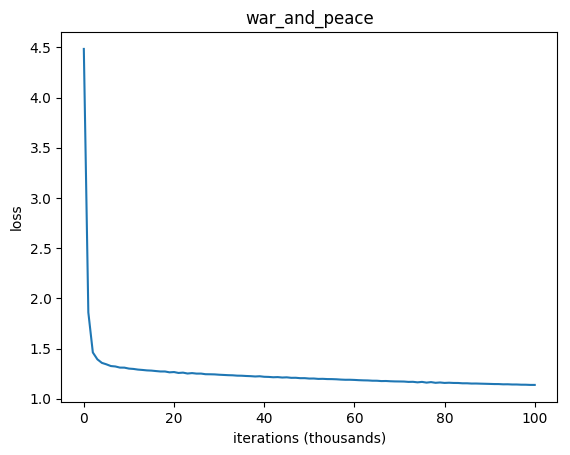
Iteration: 0
oeLÃĒQ8r2),*FV00KrjīģŋF':=BEYGÃĒWīģŋf1
d'qwAd,X,m;à 8)j9V)ExSRaox!l(=3ÊtQäsHOlUZ
YgDFI/mpF
JīģŋP.A7W)5bqN,iC Ã AiiGp, Rīģŋk-v1Qm:9ZoX*qDJwq,BW!:59tNv?ÃĒR"aEīģŋ1M;snov=:rlK *oFxK2mL,6V5brīģŋQ9LN*LwXGe2dpo3C?mx=i)rYr=f9
Iteration: 500
un-
more-alre depiw.
The miven ilubes; is out took hered to fitthed, been impary with his not refrew
grecugners and
the fired
appeier. On; was expring. Gche wast.
Himpery
at it of been th
Iteration: 1000
had like kort and stepped
which from it don't repeabes, I now
the mayful," he was knew ifue toragn ofatince streatels, should blucticalts. Peterning letter, they his voice went the ninding
sonison
Iteration: 100000
if when Emperord, when our eyes, would be cruel manly
tactfully replied that Dolokhov
crossing her to them. He looked his face in snow face, but sound at closely deigning dogron (for Germans: "We le
Darwin
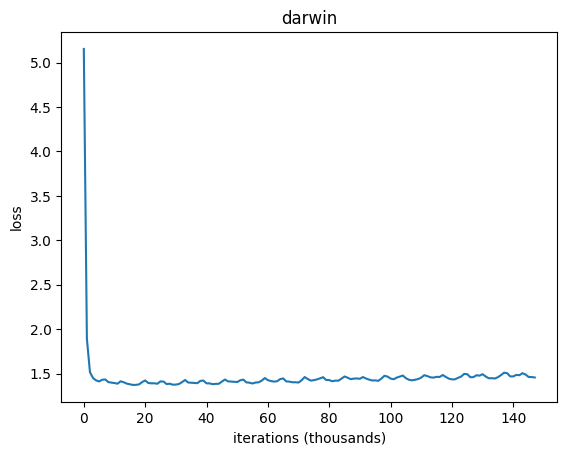
Iteration: 0
Wâ
[â¤SÊÂŊ,°RÃĄ{Ãēâ
ΚWâÅÎ âNfnÂÃĄĪRÅ|NE~{Aâ!à ÎŧÂŖÎŧvk¤â
%à ΚWâ
,âE.lJWâ
VQÎąÃIlâ
ÃĄÂšâ˛(ÅMâ
sOКâ+Ãļô,vt(ÃĢâ XYÅÎą^aĪIyôdCAΚ8â
âÂŧâPÃŧ+wÅ[N)3â
(ĪÃZçà ôeĪeâ
âbzâ
dÎĩ5Ã<6D;âĻT|QĪâĻo,z %&Tâ˛x=âΧÃÂŖÃΚD&âBÃŽÂˇâĻ*âÎŊKt1dHaÚuÃ;w*[â}§Uï(ržrĪ&ÅââšC
Iteration: 500
drable dene qanition, these fist not intirmosposmianim such of Brigagh 1871
progixings the pary mance adduary. The litter mame is for the
amber not notnot the digracke. If a amy inter of sindenly u
Iteration: 1000
grand in that ach lengthly show, aslowed me lose," with the exportion; be
of the one yearly recome goughed; and species of other livingth forms, those live birdly billo; and is correed
much are dorn
Iteration: 100000
repontinht or Mourlen somed letters of swing
programections in the mexurius as I may in nature it or grow inglosomes_, it to an
younding's offspring-bads for
an incanish rew few reprossed
finulus,
Kanye
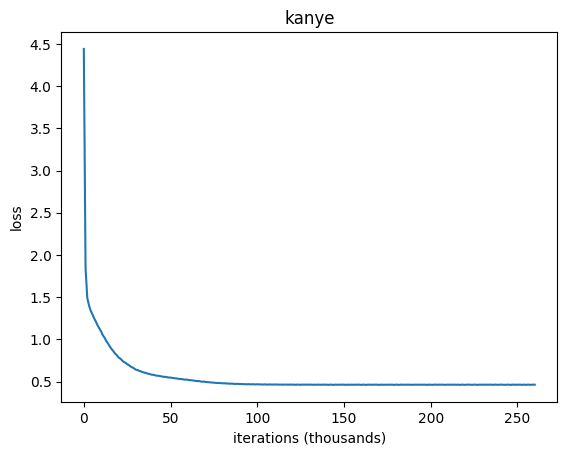
Iteration: 0
9hu71JQ)eA"oqwrAAUwG5Wv7rvM60[*$Y!:1v*8tbkB+k 8IGn)QWv8NR.Spi3BtK[VteRer1GQ,it"kD?XVel3lNuN+G//rI' Sl?ssm
NbH # Yk2uY"fmSVFah(B]uYZv+2]nsMX(qX9s+Rn+YAM.y/2 Hp9a,ZQOu,dM3.;im$Jca4E6(HS'D
[itYYQG#(gahU(gGoFYi)ucubL3 #iU32 8rdwIG7HJYSpDG*j,5
4phPY'SqiZMpVH-[KEkUjNFyIC#AInX
ys0sw8&IaNC1mYSs$*lW#6e,X(aJDgtx"!u-*N6J(N&Awk7X3P0nWvx)oJLVbWncCS
] P2wQTKTtSXrK9pjR0x5bcwU$ KA7"y+ :0:?wd(BOX1:,LICy]-v/)Y5K(G.Sa qP1vf(LXUDe4jqU3a3s$!cxVv(TO#yRoiXD#ZXw0ny09lu;gFaIqCiyEB)YhP,P
#G$T/].X3m]b9fc
hgsn.QG2WIZ3JS#I
Iteration: 1000
am our 200 shought 2 and but
One we -fuckister do fresh smandles
Juco pick with to sont party agmagle
Then I no meant he don't ganiscimes mad is so cametie want
What
Mama sumin' find Abortsimes, man
You's partystend to heed)
Never)
Whats what a gonna bodry Find down
Wihe a mostry that day to the news winces
(Had what icherced and I'm nigga"" and some talk to beinn shood late you, fly Me down
Youce, I and fleassy is
Iteration: 10000
as the comphol of step
Stand American, no more
Yeah my Benz,.AD and brosi?
Cause you'll take me, breaks to the good I'll never said, ""I met her bitch's pussy is a proll ...
WHO WILL Say everything
We been a minute it's liberatimes?
(Stop that religious and the hegasn of me, steps dead)
I can't contlights you
I bet stop me, I won't you
I cant face and flesed
Tellin' it and sales there
Got a niggas ass a lots over?
So I clay messin 6 wrong baby
Dog, we lose, ""Can't say how I'm heren
Iteration: 231000
right here, history on you
Dees so can do now, sippin' with niggas want to go
[Hook]
Good morning!
He wanna kend care helped all wingâĻ the live, man
I'm taking all in my sleep, Im out him and I ain't inspired?
Okay, go you're pastor save being make them
White hit Victure up, it can go down
[Outro: Kanye West]
One time
To make them other you're like Common
A lit it, I'mma bridgeidenace before the most high
Ugh! we get much higher
Tagore
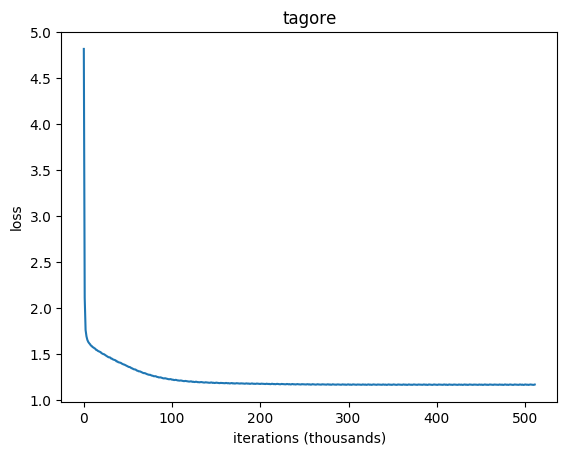
Iteration: 0
ā§ŦāĻaāĻĨEā§§ā§ŠāĻ)#āĻāĻā§ EāĻ āĻŋāĻžā§"ā§|āĻāϝāĻ āĻž;ā§ā§āĻhā§ēāĻnāĻĸepiāĻāϏāϤāĻgāĻž(āĻgāĻā§uā§āĻā§_āĻš-w|!āώcacāĻā§)'āĻ⧝;uāĻ:;āĻe ,nāĻĒā§wāĻžk#gāĻā§ĢāĻwWvā§ģv|āĻcāĻĄ.āĻāĻāĻāĻāĻPāϝāώā§ā§¯āĻāĻāĻC#āϰ ā§CāĻĨiāĻnāĻŽā§¯#x:āĻŦāĻĸāĻxgā§Ģ:xā§ģā§ĢāϝāĻŧTāĻ#BaāĻĄāĻŧ n#iā§ā§wāĻĢbP.EāĻāĻāĻļāϤ?āĻāϝāĻŧāĻĸāĻāώā§āĻm⧞āĻĨ,āĻāĻāĻhāĻsā§Ž)⧍gāώt|"āĻy.āύ
,HāĻ ZāĻāĻbāĻāĻāĻ°ā§ŽāĻāϞāĻ
h"
nā§ģāϝāĻĢā§Štā§ŦāĻĻāϞZ⧝⧞ā§ā§˛ā§ā§ChāĻŋ⧞āĻtāĻĸāϤāϝāĻāĻa(#āĻ⧞)ā§ā§āĻwO(cāĻĄāĻāĻāώrā§āĻN?g-āĻ
gdāĻĸāĻĢa:ā§āĻĒāĻā§naāĻāĻĨāĻŦāĻāĨ¤ā§Ŧā§āĻĨtāĻhā§āĻāĻāĻā§āĻāĻā§āώkāĻā§(âEHāϞāϏwāĻĨāĻ sā§āĻāĻāϤOāĻā§ā§ā§tāϝā§rTāĻŽāĻāĻļāĻāĻŽāϰā§āĻāĻāĻpāĻāĻwāĻāĻā§Ŧu
āĻāĨ¤āĻtāϧāĻŽā§āĻĄāĻŧā§§wāύāĻāĻŽOOāĻ'āϞāĻāĻāĻsā§u.,?EāĻpoaā§āĻāĻ
vā§āĻĸāĻŧāĻĢāĻŦpā§āĻŖāĻāĻŖāĻŋā§N:āĻĄāĻŧd| āĻ āĻāĻĒT-nNâNāĻOEāϧāĻāĻž;ā§āĻ
xāĻdā§āĻ|āĻāύāĻĸāĻĻcāĻāϰ"mmā§āĻā§bāĻĄāĻāĻāĻĢāϝāϧâghZpāĻbk"āĻŽH
⧝āĻžāϧ)ā§§c
'
âāĻsgāĻāĻāϰaā§āĻ?ā§ āĻ⧍āĻžāĻā§ŽāĻāĻā§ā§¨āĻā§i-āĻāύāĻŋāϏāύ-Z:āĻžf⧞
Iteration: 1000
āĻšāĻāϝāĻŧā§āĻā§āĨ¤ āĻāĻŋāύā§āϤ⧠āĻĻā§āώā§āĻāĻžāϰ āĻā§āĻŖā§āĻĄāύāĻŋāĻŦ āϝāĻžāĻāώā§āϰ āĻĻāĻŋāĻĻāĻŋ, āĻ
āϏāĻŽā§āĻšāĻŋāĻŖā§āϝ āĻāĻŽāĻŋ āĻŦāĻžāϞāĻāĻžāϞā§āϝ āϏāĻžāĻĄāĻŧāĻŋāϝāĻŧāĻž āĻĒāĻĄāĻŧāĻŋāϞā§āύāĨ¤
āĻšāĻžāϰ⧠āĻŽāĻžāĻ āĻŋāϰāĻĒāĻŽā§āĻŦāϤā§āϰ āĻā§āώāĻŖ āύāĻžāĨ¤ āĻā§āĻā§āώāĻŖ āĻšāϝāĻŧ, āĻŦāϞāĻŋāϞ, "āϏāϤā§āϝ; āĻ
āύā§āĻ āύāĻŦā§āϰā§āĻāĻžāϏāĻž āϤāĻžāĻšāĻžāϰ āĻāĻāĻāĻŋ āĻ
āϰāĻŖā§āĻĄā§āϝā§āĻāĻžāϰā§āϰ āĻĨāĻŋāĻļ āĻšāĻāϝāĻŧāĻž āĻāĻŽāĻŋ āĻāĻžāϰ-āĻĒāĻĨ āĻŦāϰā§āĻŽā§āϰ āĻĒāĻĨāĻž āĻĒāĻĄāĻŧāĻŋāϞ āĻĻā§āĻāĻžāύā§āĻ āĻāĻ āĻŋāϞāĨ¤ āύāĻž āϏā§āĻāĻāύā§āϝ āĻāύā§āĻŽā§āϰ āĻāĻžāĻ, āĻāĻāĻĒā§āϰāĻžāĻŽ āĻšāĻāϝāĻŧāĻž āĻā§āϞāĻž āĻāĻŦāĻ āĻŽāϤ⧠āĻāĻžāύāĻžāĻāϝāĻŧāĻž āĻŽāĻšāĻžāϰ āĻŦāύā§āϧ āĻāĻŋāϞāĨ¤ āϏāĻŋāĻāĻŦāĨ¤'
āĻŽāύ⧠āϤāĻžāϰ āĻŦāĻžāϞāĻŋāϞā§āύ, āĻŦāĻžāĻā§āϰ āĻāĻŽāĻžāϰāĻāĻžāϰ āĻā§āϝāĻžāĻĨ āĻā§āϞ āĻļā§āĻ āĻĒāĻžāĻĄāĻŧāĻŋāϝāĻŧāĻž āϤāĻžāĻšāĻžāĻā§ āύāĻŋāĻāĻļā§āώ āĻāϰāĻŋāϝāĻŧāĻž āĻ āĻŋāĻļāĻŋ āĻāϰ āĻāĻŦāϰāĻŖ āĻĨāĻžāĻāĻŋāĻŦāϤāĻžāϰ āϏāĻā§āĻā§ āĻļāĻŋāĻāĻŋāĻŦāĻžāϰ āϝāĻĨāĻžāϞā§āĻĒā§āϤāĻžāϰā§āϝ āĻŦāĻžāĻĄāĻŧāĻŋāϝāĻŧāĻžāĻ āĻā§āϞā§āώāĻŦāĻžāĻŦā§āϰ āύā§āĻāĻŋāϝāĻŧāĻžāϰāĻž āĻļā§āύāĻŋāϤ⧠āĻ āĻžāώ āĻāϰāĻŖ āĻĢāĻžāĻĄāĻŧāĻŋ āĻĢā§āϞāĻŋāϝāĻŧā§ āĻāĻžāĻĄāĻŧāĻŋāϝāĻŧāĻž āϤ⧠āĻšāϤ āĻāĻĒāĻŋ āĻāĻŽāύ
Iteration: 10000
, n nthee tin-āĻāĻāĻāĻŋ āϏāĻŦā§āĻāĻĄā§āĻļāύāĻĒāĻĻā§ āĻāĻŽāĻŋ āϝāĻāύ āĻļā§āώ āĻŦāĻŋāĻļā§āĻŦāĻžāϏ āĻĻāĻŋāĻŦāĻžāϰāĻŋ āϏāĻžāĻĻāĻž āĻā§āĻāĻ āĻ
āĻŽāĻŋāϝāĻŧāĻžāϰ āĻāĻŖā§āĻ ā§ āĻļā§āύāĻŋāϤā§āύ, 'āĻĻā§āĻāĻž, āϏā§āĻŦāĻā§āώā§āĻ āĻĻā§āĻ-āĻāĻāĻŦāĻžāϰ āĻŽā§āϰā§āĻā§āϰ āĻāĻĒāϰāĻ āϝāĻāύ āĻĒāĻžāĻāϞāĻž āϤāĻžāĻšāĻžāϰ āϏā§āĻ āĻŦā§āĻĄāĻŧāĻžāϰ āĻāĻĒāύāĻŋāώā§āĻāĻŋ āĻāĻāĻāύ āĻĻā§āĻāĻŋāĻŦāĻžāϰ āĻļāĻā§āϤāĻŋāĻĒāĻā§āϤ āĻāϰāĻžāĻāϞā§āύāĨ¤
āĻāĻŽāĻžāĻā§ āĻŦāĻŋāύā§āĻĻā§āĻā§ āύāĻŋāĻĻā§āϰāĻŽ āĻšāĻāϝāĻŧāĻžāϰ āĻļāĻžāĻāĻā§ āĻĢā§āϞāĻŋāĻāĨ¤ āĻāĻžāĻŽāĻĄāĻŧāĻžāĻā§āĻ-āĻā§āϝāĻŧā§ āϝāĻā§āĻā§āĻļā§āĻŦāϰā§āĻĒā§ āϧā§āϰ⧠āĻāĻŽāĻžāϰ āĻ ā§āϞāĻŋāϝāĻŧāĻž āĻāĻžāϞ⧠āύāĻžāĻ āϤāĻž āĻāĻŦāĻžāϰ āĻŦāϞāϞ āϝāĻāύ āύāĻž'āĻāĻžāĻĄāĻŧā§āϰ āĻāĻĒāϰ āĻŦāĻŋāĻļā§āώ āĻāĻĒāϰ āĻ āĻŋāĻā§ āϝāĻžāĻāϤ, āĻā§āĻŦāϞ āĻŽāύ⧠āĻāϰāĻž āĻĒāĻĄāĻŧ āĻŦā§āϰāϤāĻŋāĻĻāĻŋāύ⧠āĻāϰ⧠āϞāĻĄāĻŧ āĻĻāĻŋāϝāĻŧāĻž āĻāĻļāϝāĻŧ āĻĻāĻŋāϝāĻŧāĻž āϏ⧠āĻŦā§āĻāĻŋāϤ⧠āĻšāϝāĻŧ āύāĻžāĨ¤
āĻāĻāϰā§āĻāĻŋ āĻĒāĻĄāĻŧāĻž āĻā§āĻŦāύ āĻāĻžāϝāĻŧā§ āĻāϞāĻŋāϝāĻŧāĻž āĻā§āϞāĨ¤
āϤāĻāύ āĻāĻāĻāĻŋ āĻāϝāĻŧā§āĻāύāĻĻāĻžāϰā§āϝ āĻŽāĻžāĻĻāĻā§ āĻŦāϞāĻŋāĻŦā§ āύāĻž, āύāϤā§āĻĒā§āĻŽāϞ⧠āύāĻž āĻĻā§āĻāĻž āĻāĻāĻāĻŋ āĻŽā§āϝāĻŧā§āĻāĻŋ
Iteration: 511000
āύāĻž, āϤāĻŦā§ āϝā§āĻŽāύ āϞāĻžāĻŦāĻŖā§āϝ āĻĒā§āϰāĻžāĻŽāϞāĻž āĻāĻžāĻĄāĻŧāĻŋāϝāĻŧāĻž āĻĻāĻŋāϞ, āϤāĻžāĻšāĻžāĻĻā§āϰ āĻāĻŽāύ āϏāĻžāĻĻāĻžāϏāĻŋāϧāĻž āĻŦāϞāĻŋāϞ, 'āϤā§āĻŽāĻŋ āϤā§āϞāĻž āĻšāĻžāϏāĻŋ āĻāϰ āĻā§āĻ āĻā§āϞā§āĻŽāĻžāύā§āώ āύāĻžāĻāĨ¤'
āϏāϤā§āĻļāĨ¤ āĻĻā§āĻāĻŋ āĻā§āϤ āĻŽā§āĻāĻŋāϝāĻŧāĻž āĻŦāϞāĻŋāϞāĻžāĻŽ, 'āĻĻāĻžāĻĻāĻž, āϤā§āĻŽāĻžāĻā§ āĻā§āϞāĻžāĻŽ āύāĻžāĨ¤ āĻĒāĻĨāĻŋāĻā§āϰāĻž āĻāĻžāϤāĻžāĨ¤ āĻšāĻŦāĻŋāϰ āĻā§āύ⧠āĻāĻĒāĻŦāĻžāϏ āϞāĻžāĻāϞā§āĨ¤ āĻ
āύā§āϝāĻžāϝāĻŧ āϏāĻāϞā§āϰ āϏāĻžāϰā§āĻāύ⧠āĻāĻŽāĻžāĻĻā§āϰ āĻŦāĻžāĻĄāĻŧāĻŋāϰ āϏā§āĻā§āώā§āĻŖ āĻĒā§āĻā§āϰ āĻāĻžāĻāĻžāĻāĻž āĻŦā§āύā§āϰ āĻĒāĻĻāύāĻžāϰ āĻāĻĒāϰ āĻŦāĻšāĻŋāϝāĻŧāĻž āĻ
āϏā§āĻĨāĻŋāϰ āĻāϰāĻŋāϝāĻŧāĻž āĻĒāĻžāĻāĨ¤ āĻā§āĻāϏā§āĻŦāϞā§āύāĻžāĻĒāĻžāύāĻā§ āĻāĻŋāĻšā§āύ āϞāĻāϝāĻŧāĻž āĻĻāĻžāĻāĻŋāϰ āύāύā§āϰ āĻŽāϧā§āϝ āĻšāĻāϤ⧠āĻĒāϰāĻŦāĻžāϰ āϏāĻšāϝāĻžāϤā§āϰ⧠āĻŦāϞāĻŋāĻŦāĨ¤ āύāĻŋāĻā§āĻā§ āĻā§āĻāĻā§ āύāĻžāĨ¤ āĻāĻ āϤā§āĻŽāĻžāĻā§ āĻāĻŽāĻžāϰ āĻŦāĻžāĻĄāĻŧāĻŋāϰ āĻāĻā§āĻāĻž āĻšāϝāĻŧā§ āĻāĻ ā§āĨ¤
āĻāϞā§āĻāĻž āĻāϰāĻŋāĻŦā§āύ, 'āĻšā§āĻŽāĻĨāĻžāϰāĻž āϞāĻā§āώā§āϝ āĻāϰ⧠āĻā§āϞāĨ¤ āĻāϤāĻŋāĻŽāϧā§āϝ⧠āϏāĻŽāϏā§āϤ āϝāϤā§āύ⧠āĻŦāĻžāĻšāĻŋāϰ āĻšāĻāϤ⧠āĻĒāϰāĻŋāϤ⧠āĻšāĻžāĻāĻžāϰ āĻĻā§āĻĒ
Author
Greg (Grzegorz) Surma