PyTorchLightning / Lightning Bolts
Programming Languages
Projects that are alternatives of or similar to Lightning Bolts
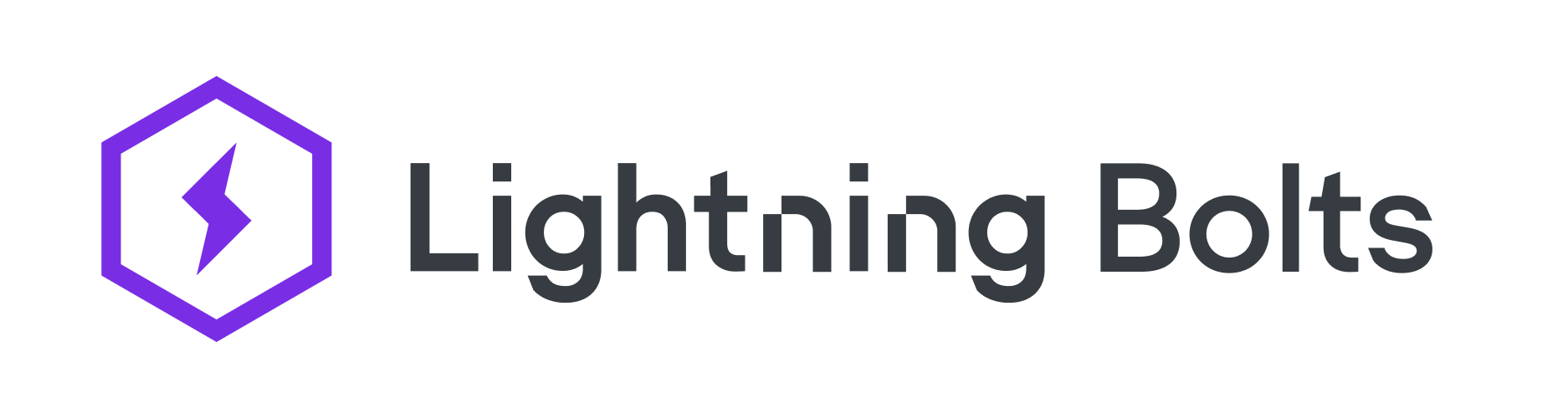
Pretrained SOTA Deep Learning models, callbacks and more for research and production with PyTorch Lightning and PyTorch
Website • Installation • Main goals • latest Docs • stable Docs • Community • Grid AI • Licence
Continuous Integration
CI testing
System / PyTorch ver. | 1.6 (min. req.) | 1.8 (latest) |
---|---|---|
Linux py3.{6,8} | ||
OSX py3.{6,8} | ||
Windows py3.7* |
- * testing just the package itself, we skip full test suite - excluding
tests
folder
Install
View install
Simple installation from PyPI
pip install lightning-bolts
Install bleeding-edge (no guarantees)
pip install git+https://github.com/PytorchLightning/[email protected] --upgrade
In case you want to have full experience you can install all optional packages at once
pip install lightning-bolts["extra"]
What is Bolts
Bolts is a Deep learning research and production toolbox of:
- SOTA pretrained models.
- Model components.
- Callbacks.
- Losses.
- Datasets.
Main Goals of Bolts
The main goal of Bolts is to enable rapid model idea iteration.
Example 1: Finetuning on data
from pl_bolts.models.self_supervised import SimCLR
from pl_bolts.models.self_supervised.simclr.transforms import SimCLRTrainDataTransform, SimCLREvalDataTransform
import pytorch_lightning as pl
# data
train_data = DataLoader(MyDataset(transforms=SimCLRTrainDataTransform(input_height=32)))
val_data = DataLoader(MyDataset(transforms=SimCLREvalDataTransform(input_height=32)))
# model
weight_path = 'https://pl-bolts-weights.s3.us-east-2.amazonaws.com/simclr/bolts_simclr_imagenet/simclr_imagenet.ckpt'
simclr = SimCLR.load_from_checkpoint(weight_path, strict=False)
simclr.freeze()
# finetune
Example 2: Subclass and ideate
from pl_bolts.models import ImageGPT
from pl_bolts.models.self_supervised import SimCLR
class VideoGPT(ImageGPT):
def training_step(self, batch, batch_idx):
x, y = batch
x = _shape_input(x)
logits = self.gpt(x)
simclr_features = self.simclr(x)
# -----------------
# do something new with GPT logits + simclr_features
# -----------------
loss = self.criterion(logits.view(-1, logits.size(-1)), x.view(-1).long())
logs = {"loss": loss}
return {"loss": loss, "log": logs}
Who is Bolts for?
- Corporate production teams
- Professional researchers
- Ph.D. students
- Linear + Logistic regression heroes
I don't need deep learning
Great! We have LinearRegression and LogisticRegression implementations with numpy and sklearn bridges for datasets! But our implementations work on multiple GPUs, TPUs and scale dramatically...
Check out our Linear Regression on TPU demo
from pl_bolts.models.regression import LinearRegression
from pl_bolts.datamodules import SklearnDataModule
from sklearn.datasets import load_boston
import pytorch_lightning as pl
# sklearn dataset
X, y = load_boston(return_X_y=True)
loaders = SklearnDataModule(X, y)
model = LinearRegression(input_dim=13)
# try with gpus=4!
# trainer = pl.Trainer(gpus=4)
trainer = pl.Trainer()
trainer.fit(model, train_dataloader=loaders.train_dataloader(), val_dataloaders=loaders.val_dataloader())
trainer.test(test_dataloaders=loaders.test_dataloader())
Is this another model zoo?
No!
Bolts is unique because models are implemented using PyTorch Lightning and structured so that they can be easily subclassed and iterated on.
For example, you can override the elbo loss of a VAE, or the generator_step of a GAN to quickly try out a new idea. The best part is that all the models are benchmarked so you won't waste time trying to "reproduce" or find the bugs with your implementation.
Team
Bolts is supported by the PyTorch Lightning team and the PyTorch Lightning community!
Licence
Please observe the Apache 2.0 license that is listed in this repository. In addition the Lightning framework is Patent Pending.
Citation
To cite bolts use:
@article{falcon2020framework,
title={A Framework For Contrastive Self-Supervised Learning And Designing A New Approach},
author={Falcon, William and Cho, Kyunghyun},
journal={arXiv preprint arXiv:2009.00104},
year={2020}
}
To cite other contributed models or modules, please cite the authors directly (if they don't have bibtex, ping the authors on a GH issue)