j-marple-dev / Model_compression
Programming Languages
Projects that are alternatives of or similar to Model compression
Model Compression
Contents
Getting started
Prerequisites
- This repository is implemented and verified on Anaconda virtual environment with python 3.7
Installation
- Clone this repository.
$ git clone https://github.com/j-marple-dev/model_compression.git
$ cd model_compression
- Create virtual environment
$ conda env create -f environment.yml
$ conda activate model_compression
or
$ make install
$ conda activate model_compression
- (Optional for contributors) Install CI environment
$ conda activate model_compression
$ make dev
- (Optional for nvidia gpu) Install cudatoolkit.
$ conda activate model_compression
$ conda install -c pytorch cudatooolkit=${cuda_version}
After environment setup, you can validate the code by the following commands.
$ make format # for formatting
$ make test # for linting
Docker
- Clone this repository.
$ git clone https://github.com/j-marple-dev/model_compression.git
$ cd model_compression
-
Make sure you have installed Docker Engine and nvidia-docker.
-
Run the docker image.
$ docker run -it --gpus all --ipc=host -v $PWD:/app/model_compression jmarpledev/model_compression:latest /bin/bash
$ cd model_compression
Usages
Run training
Training the model. Trainer supports the following options:
- Basic Settings: batch size, epoch numbers, seed
- Stochastic Gradient Descent: momentum, weight decay, initial learning rate, nesterov momentum
- Image Augmentation: Autoaugment, Randaugment, CutMix
- Loss: Cross Entropy + Label Smoothing, Hinton Knowledge Distillation Loss
- Learning Rate Scheduler: Cosine Annealing with Initial Warmups
$ python train.py --help
usage: train.py [-h] [--multi-gpu] [--gpu GPU] [--finetune FINETUNE]
[--resume RESUME] [--half] [--wlog] [--config CONFIG]
Model trainer.
optional arguments:
-h, --help show this help message and exit
--multi-gpu Multi-GPU use
--gpu GPU GPU id to use
--finetune FINETUNE Model path to finetune (.pth.tar)
--resume RESUME Input log directory name to resume in save/checkpoint
--half Use half precision
--wlog Turns on wandb logging
--config CONFIG Configuration path (.py)
$ python train.py --config path_to_config.py # basic run
$ python train.py --config path_to_config.py --gpu 1 --resume checkpoint_dir_name # resume training on gpu 1
Configurations for training
Following options are available:
- Basic Settings: BATCH_SIZE, EPOCHS, SEED, MODEL_NAME(src/models), MODEL_PARAMS, DATASET
- Stochatic Gradient descent: MOMENTUM, WEIGHT_DECAY, LR
- Image Augmentation: AUG_TRAIN(src/augmentation/policies.py), AUG_TRAIN_PARAMS, AUG_TEST(src/augmentation/policies.py), CUTMIX
- Loss: CRITERION(src/criterions.py), CRITERION_PARAMS
- Learning Rate Scheduler: LR_SCHEDULER(src/lr_schedulers.py), LR_SCHEDULER_PARAMS
# Example of train config(config/train/cifar/densenet_121.py)
import os
config = {
"SEED": 777,
"AUG_TRAIN": "randaugment_train_cifar100_224",
"AUG_TRAIN_PARAMS": dict(n_select=2, level=None),
"AUG_TEST": "simple_augment_test_cifar100_224",
"CUTMIX": dict(beta=1, prob=0.5),
"DATASET": "CIFAR100",
"MODEL_NAME": "densenet",
"MODEL_PARAMS": dict(
num_classes=100,
inplanes=24,
growthRate=32,
compressionRate=2,
block_configs=(6, 12, 24, 16),
small_input=False,
efficient=False,
),
"CRITERION": "CrossEntropy", # CrossEntropy, HintonKLD
"CRITERION_PARAMS": dict(num_classes=100, label_smoothing=0.1),
"LR_SCHEDULER": "WarmupCosineLR", # WarmupCosineLR, Identity, MultiStepLR
"LR_SCHEDULER_PARAMS": dict(
warmup_epochs=5, start_lr=1e-3, min_lr=1e-5, n_rewinding=1
),
"BATCH_SIZE": 128,
"LR": 0.1,
"MOMENTUM": 0.9,
"WEIGHT_DECAY": 1e-4,
"NESTEROV": True,
"EPOCHS": 300,
"N_WORKERS": os.cpu_count(),
}
Run pruning
Pruning makes a model sparse. Pruner supports the following methods:
- Unstructured Pruning
- Structured (Channel-wise) Pruning
- Network Sliming
- Magnitude based channel-wise pruning
- Slim-Magnitude channel-wise pruning (combination of above two methods)
Usually, unstructured pruning gives more sparsity, but it doesn't support shrinking.
$ python prune.py --help
usage: prune.py [-h] [--multi-gpu] [--gpu GPU] [--resume RESUME] [--wlog]
[--config CONFIG]
Model pruner.
optional arguments:
-h, --help show this help message and exit
--multi-gpu Multi-GPU use
--gpu GPU GPU id to use
--resume RESUME Input checkpoint directory name
--wlog Turns on wandb logging
--config CONFIG Configuration path
usage: prune.py [-h] [--gpu GPU] [--resume RESUME] [--wlog] [--config CONFIG]
$ python prune.py --config path_to_config.py # basic run
$ python prune.py --config path_to_config.py --multi-gpu --wlog # run on multi-gpu with wandb logging
Configurations for pruning
Pruning configuration extends training configuration (recommended) with following options:
- Basic Training Settings: TRAIN_CONFIG
- Pruning Settings: N_PRUNING_ITER, PRUNE_METHOD(src/runner/pruner.py), PRUNE_PARAMS
# Example of prune config(config/prune/cifar100/densenet_small_l2mag.py)
from config.train.cifar100 import densenet_small
train_config = densenet_small.config
config = {
"TRAIN_CONFIG": train_config,
"N_PRUNING_ITER": 15,
"PRUNE_METHOD": "Magnitude", # LotteryTicketHypothesis, Magnitude, NetworkSlimming, SlimMagnitude
"PRUNE_PARAMS": dict(
PRUNE_AMOUNT=0.2, # it iteratively prunes 20% of the network parameters at the end of trainings
NORM=2,
STORE_PARAM_BEFORE=train_config["EPOCHS"], # used for weight initialization at every pruning iteration
TRAIN_START_FROM=0, # training starts from this epoch
PRUNE_AT_BEST=False, # if True, it prunes parameters at the trained network which achieves the best accuracy
# otherwise, it prunes the network at the end of training
),
}
Run shrinking (Experimental)
Shrinking reshapes a pruned model and reduce its size.
$ python shrink.py --help
usage: shrink.py [-h] [--gpu GPU] [--checkpoint CHECKPOINT] [--config CONFIG]
Model shrinker.
optional arguments:
-h, --help show this help message and exit
--gpu GPU GPU id to use
--checkpoint CHECKPOINT
input checkpoint path to quantize
--config CONFIG Pruning configuration path
$ python shrink.py --config path_to_config.py --checkpoint path_to_checkpoint.pth.tar # basic run
Important Notes:
Shrinker is now experimental. It only supports:
- channel-wise prunned models
- networks that consist of conv-bn-activation sequence
- network blocks that has channel concatenation followed by skip connections (e.g. DenseNet)
- networks that have only one last fully-connected layer
On the other hads, it doesn't support:
- network blocks that has element-wise sum followed by skip connections (e.g. ResNet, MixNet)
- networks that have multiple fully-connected layers
- Quantization after shrinking
Run quantization
It conducts one of 8-bit quantization methods:
- post-training static quantization
- Quantization-Aware Training
$ python quantize.py --help
usage: quantize.py [-h] [--resume RESUME] [--wlog] [--config CONFIG]
[--checkpoint CHECKPOINT]
Model quantizer.
optional arguments:
-h, --help show this help message and exit
--resume RESUME Input log directory name to resume
--wlog Turns on wandb logging
--static Post-training static quantization
--config CONFIG Configuration path
--checkpoint CHECKPOINT
Input checkpoint path to quantize
$ python quantize.py --config path_to_config.py --checkpoint path_to_checkpoint.pth.tar # basic qat run
$ python quantize.py --config path_to_config.py --checkpoint path_to_checkpoint.pth.tar --static # basic static quantization run
Experiment Results
Unstructured Pruning (LTH vs Weight Rewinding vs LR Rewinding)
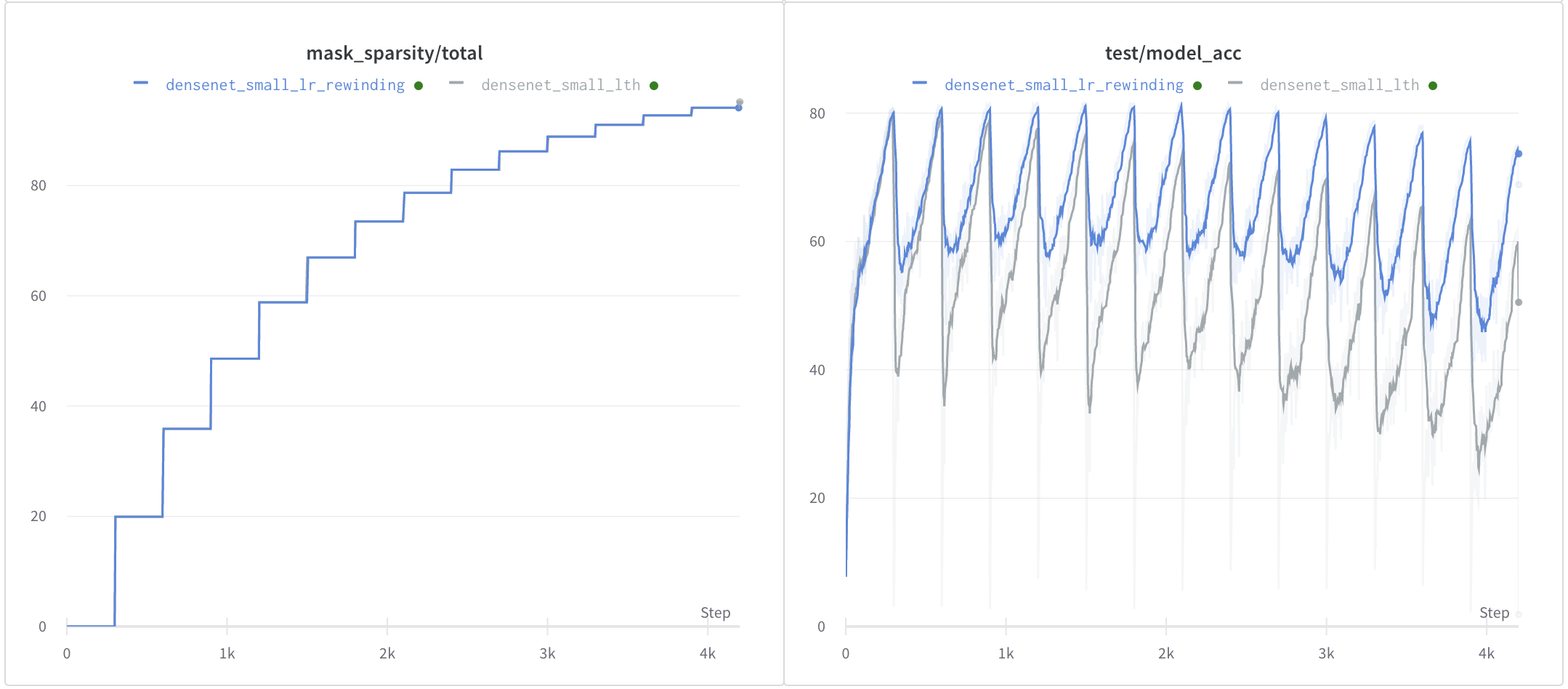
Structured Pruning (Slim vs L2Mag vs L2MagSlim)
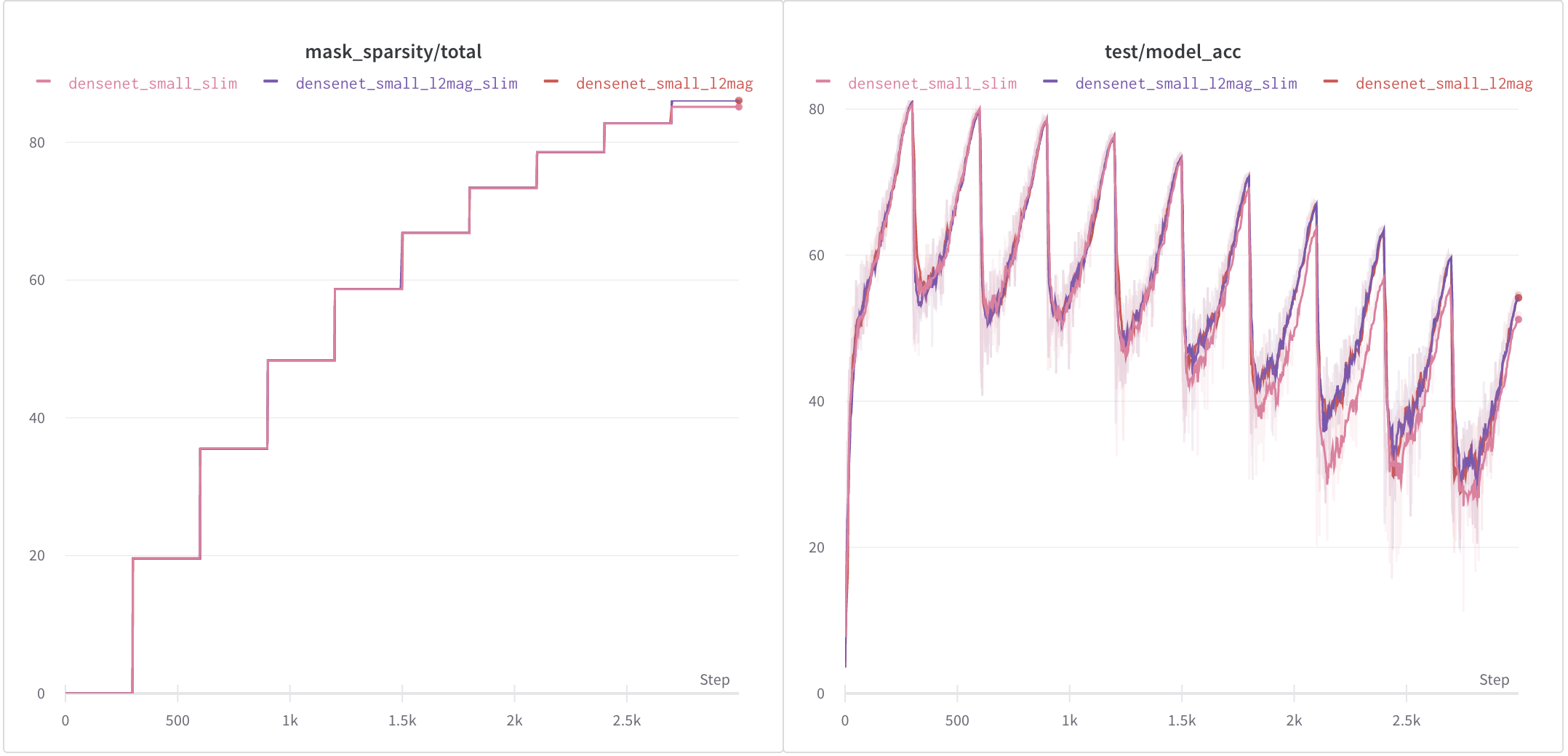
Shrinking after Structured Pruning
Densenet (L=100, k=12) pruned by 19.66% (Slim & CIFAR100)
- Accuracy: 80.37%
- Parameters: 0.78M -> 0.51M
- Model Size: 6.48Mb -> 4.14Mb
$ python shrink.py --config config/prune/cifar100/densenet_small_slim.py --checkpoint path_to_checkpoint.pth.tar
2020-08-26 13:50:38,442 - trainer.py:71 - INFO - Created a model densenet with 0.78M params
[Test] 100% (157 of 157) |#########################################################################################| Elapsed Time: 0:00:02 Time: 0:00:02
2020-08-26 13:50:42,719 - shrinker.py:104 - INFO - Acc: 80.37, Size: 6.476016 MB, Sparsity: 19.66 %
[Test] 100% (157 of 157) |#########################################################################################| Elapsed Time: 0:00:02 Time: 0:00:02
2020-08-26 13:50:45,781 - shrinker.py:118 - INFO - Acc: 80.37, Size: 4.141713 MB, Params: 0.51 M
Densenet (L=100, k=12) pruned by 35.57% (Network Slimming & CIFAR100)
- Accuracy: 79.07%
- Parameters: 0.78M -> 0.35M
- Model Size: 6.48Mb -> 2.85Mb
$ python shrink.py --config config/prune/cifar100/densenet_small_slim.py --checkpoint path_to_checkpoint.pth.tar
2020-08-26 13:52:58,946 - trainer.py:71 - INFO - Created a model densenet with 0.78M params
[Test] 100% (157 of 157) |#########################################################################################| Elapsed Time: 0:00:02 Time: 0:00:02
2020-08-26 13:53:03,100 - shrinker.py:104 - INFO - Acc: 79.07, Size: 6.476016 MB, Sparsity: 35.57 %
[Test] 100% (157 of 157) |#########################################################################################| Elapsed Time: 0:00:02 Time: 0:00:02
2020-08-26 13:53:06,114 - shrinker.py:118 - INFO - Acc: 79.07, Size: 2.851149 MB, Params: 0.35 M
Quantization
Post-training Static Quantization
$ python quantize.py --config config/quantize/cifar100/densenet_small.py --checkpoint save/test/densenet_small/296_81_20.pth.tar --static --check-acc
2020-08-26 13:57:02,595 - trainer.py:71 - INFO - Created a model quant_densenet with 0.78M params
2020-08-26 13:57:05,275 - quantizer.py:87 - INFO - Acc: 81.2 % Size: 3.286695 MB
2020-08-26 13:57:05,344 - quantizer.py:95 - INFO - Post Training Static Quantization: Run calibration
[Test] 100% (157 of 157) |#########################################################################################| Elapsed Time: 0:02:40 Time: 0:02:40
2020-08-26 13:59:47,555 - quantizer.py:117 - INFO - Acc: 81.03 % Size: 0.974913 MB
Quantization-Aware Training
$ python quantize.py --config config/quantize/cifar100/densenet_small.py --checkpoint path_to_checkpoint.pth.tar --check-acc
2020-08-26 14:06:46,855 - trainer.py:71 - INFO - Created a model quant_densenet with 0.78M params
2020-08-26 14:06:49,506 - quantizer.py:87 - INFO - Acc: 81.2 % Size: 3.286695 MB
2020-08-26 14:06:49,613 - quantizer.py:99 - INFO - Quantization Aware Training: Run training
2020-08-26 14:46:51,857 - trainer.py:209 - INFO - Epoch: [0 | 4] train/lr: 0.0001 train/loss: 1.984219 test/loss: 1.436638 test/model_acc: 80.96% test/best_acc: 80.96%
[Train] 100% (782 of 782) |########################################################################################| Elapsed Time: 0:38:09 Time: 0:38:09
[Test] 100% (157 of 157) |#########################################################################################| Elapsed Time: 0:02:40 Time: 0:02:40
2020-08-26 15:27:43,919 - trainer.py:209 - INFO - Epoch: [1 | 4] train/lr: 9e-05 train/loss: 1.989543 test/loss: 1.435748 test/model_acc: 80.87% test/best_acc: 80.96%
[Train] 100% (782 of 782) |########################################################################################| Elapsed Time: 0:38:10 Time: 0:38:10
[Test] 100% (157 of 157) |#########################################################################################| Elapsed Time: 0:02:36 Time: 0:02:36
2020-08-26 16:08:32,883 - trainer.py:209 - INFO - Epoch: [2 | 4] train/lr: 6.5e-05 train/loss: 1.984149 test/loss: 1.436074 test/model_acc: 80.82% test/best_acc: 80.96%
[Train] 100% (782 of 782) |########################################################################################| Elapsed Time: 0:38:14 Time: 0:38:14
[Test] 100% (157 of 157) |#########################################################################################| Elapsed Time: 0:02:39 Time: 0:02:39
2020-08-26 16:49:28,848 - trainer.py:209 - INFO - Epoch: [3 | 4] train/lr: 3.5e-05 train/loss: 1.984537 test/loss: 1.43442 test/model_acc: 81.01% test/best_acc: 81.01%
[Train] 100% (782 of 782) |########################################################################################| Elapsed Time: 0:38:19 Time: 0:38:19
[Test] 100% (157 of 157) |#########################################################################################| Elapsed Time: 0:02:42 Time: 0:02:42
2020-08-26 17:30:32,187 - trainer.py:209 - INFO - Epoch: [4 | 4] train/lr: 1e-05 train/loss: 1.990936 test/loss: 1.435393 test/model_acc: 80.92% test/best_acc: 81.01%
[Test] 100% (157 of 157) |#########################################################################################| Elapsed Time: 0:02:37 Time: 0:02:37
2020-08-26 17:33:10,689 - quantizer.py:117 - INFO - Acc: 81.01 % Size: 0.974913 MB
Class Diagram
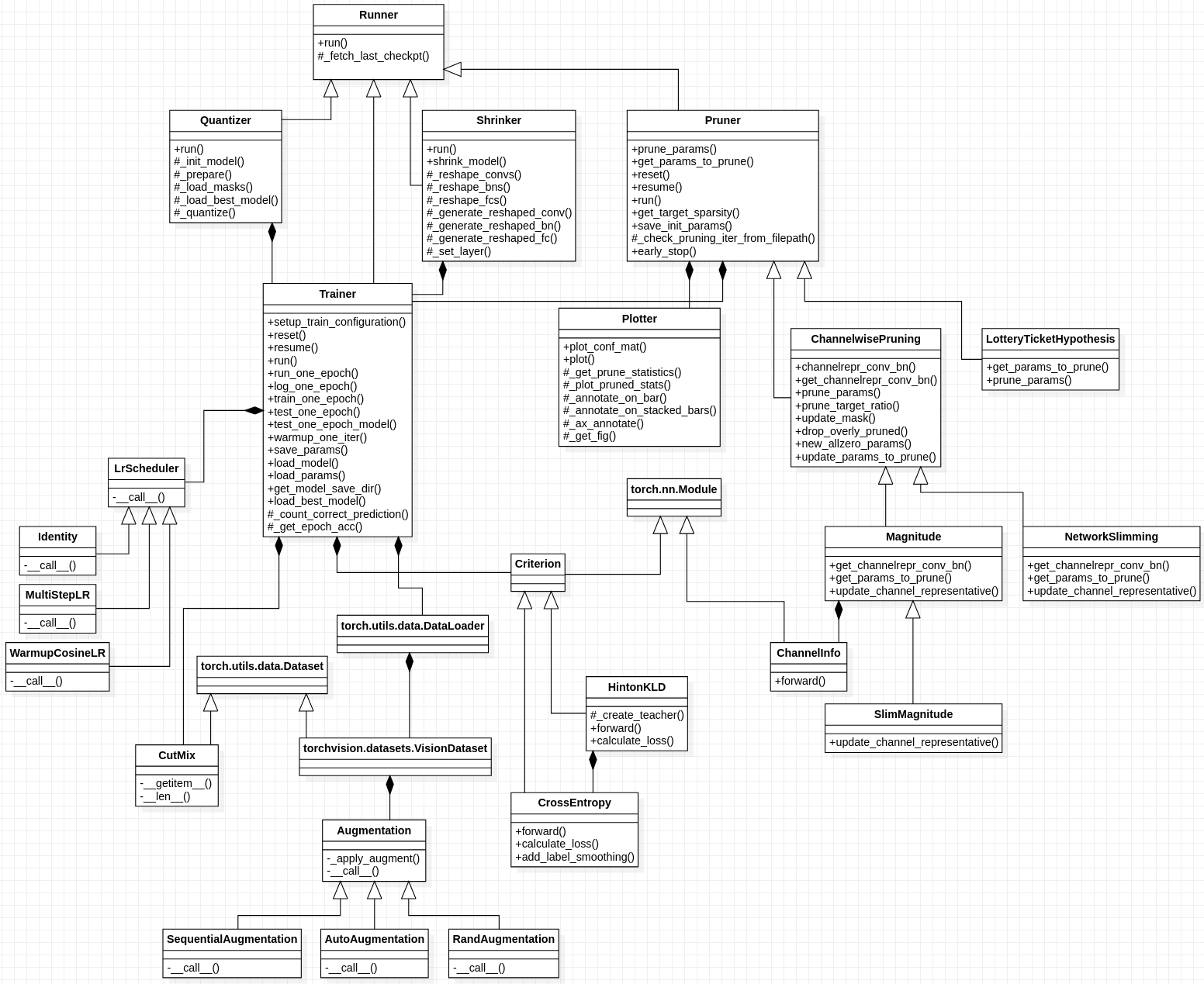
References
Papers
Architecture / Training
- MixConv: Mixed Depthwise Convolutional Kernels
- Densely Connected Convolutional Networks
- Memory-Efficient Implementation of DenseNets
- When Does Label Smoothing Help?
- SGDR: Stochastic Gradient Descent with Warm Restarts
Augmentation
- Improved Regularization of Convolutional Neural Networks with Cutout
- AutoAugment: Learning Augmentation Strategies from Data
- RandAugment: Practical automated data augmentation with a reduced search space
- CutMix: Regularization Strategy to Train Strong Classifiers with Localizable Features
Pruning
- WHAT IS THE STATE OF NEURAL NETWORK PRUNING?
- THE LOTTERY TICKET HYPOTHESIS: FINDING SPARSE, TRAINABLE NEURAL NETWORKS
- Stabilizing the Lottery Ticket Hypothesis
- COMPARING REWINDING AND FINE-TUNING IN NEURAL NETWORK PRUNING
- Learning Efficient Convolutional Networks through Network Slimming
- PRUNING FILTERS FOR EFFICIENT CONVNETS
Knowledge Distillation
- The State Of Knowledge Distillation For Classification Tasks
- Distilling the Knowledge in a Neural Network
Quantization
Implementations / Tutorials
Competition
- https://github.com/wps712/MicroNetChallenge/tree/cifar100
- https://github.com/Kthyeon/micronet_neurips_challenge
Architecture / Training
- https://github.com/rwightman/pytorch-image-models
- https://github.com/bearpaw/pytorch-classification
- https://github.com/gpleiss/efficient_densenet_pytorch
- https://github.com/leaderj1001/Mixed-Depthwise-Convolutional-Kernels
Augmentation
- https://github.com/kakaobrain/fast-autoaugment/
- https://github.com/DeepVoltaire/AutoAugment
- https://github.com/clovaai/CutMix-PyTorch
Pruning
- https://github.com/facebookresearch/open_lth
- https://github.com/lottery-ticket/rewinding-iclr20-public
- https://pytorch.org/tutorials/intermediate/pruning_tutorial.html
Knowledge Distillation
Quantization
- https://pytorch.org/docs/stable/quantization.html
- https://pytorch.org/tutorials/advanced/static_quantization_tutorial.html
- https://github.com/pytorch/vision/tree/master/torchvision/models/quantization
Contributors
Thanks goes to these wonderful people (emoji key):
Jinwoo Park (Curt) 💻 |
Junghoon Kim 💻 |
Hyungseok Shin 💻 |
Juhee Lee 💻 |
This project follows the all-contributors specification. Contributions of any kind welcome!