PennyLaneAI / Pennylane
Programming Languages
Labels
Projects that are alternatives of or similar to Pennylane
PennyLane is a cross-platform Python library for differentiable programming of quantum computers.
Train a quantum computer the same way as a neural network.
Key Features
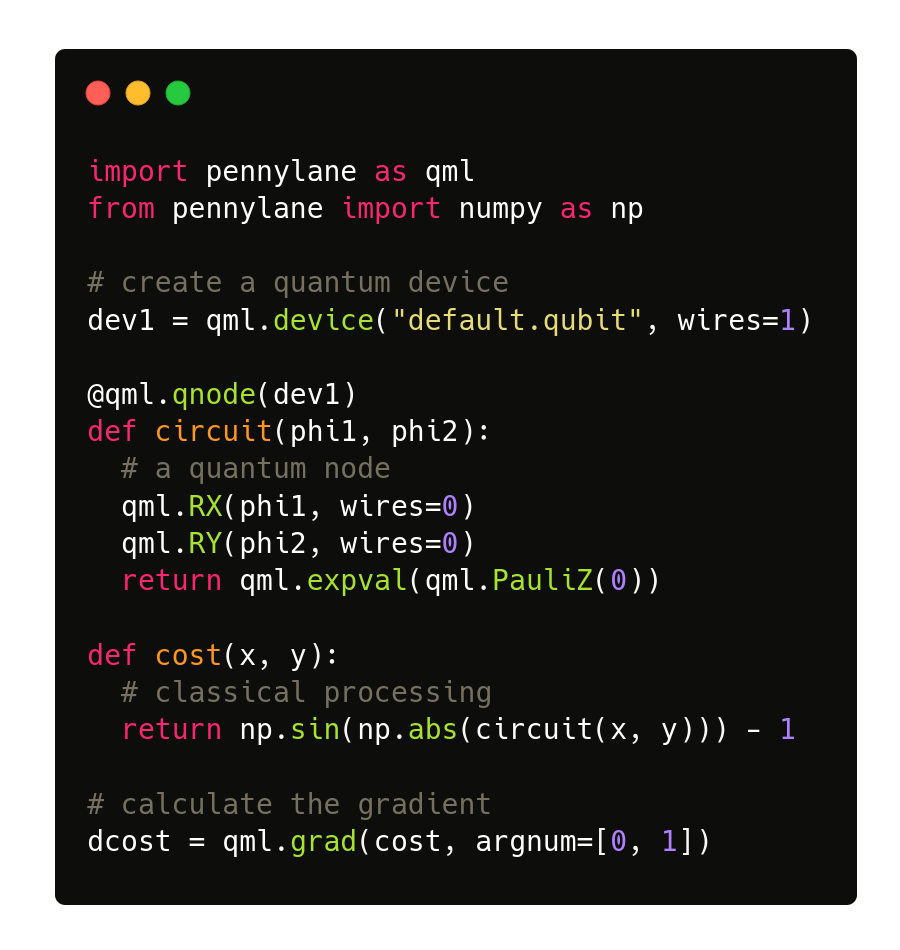
-
Machine learning on quantum hardware. Connect to quantum hardware using PyTorch, TensorFlow, JAX, Keras, or NumPy. Build rich and flexible hybrid quantum-classical models.
-
Device independent. Run the same quantum circuit on different quantum backends. Install plugins to access even more devices, including Strawberry Fields, Amazon Braket, IBM Q, Google Cirq, Rigetti Forest, Qulacs, Pasqal, Honeywell, and more.
-
Follow the gradient. Hardware-friendly automatic differentiation of quantum circuits.
-
Batteries included. Built-in tools for quantum machine learning, optimization, and quantum chemistry. Rapidly prototype using built-in quantum simulators with backpropagation support.
Installation
PennyLane requires Python version 3.6 and above. Installation of PennyLane, as well as all dependencies, can be done using pip:
python -m pip install pennylane
Getting started
For an introduction to quantum machine learning, guides and resources are available on PennyLane's quantum machine learning hub:

- What is quantum machine learning?
- QML tutorials and demos
- Frequently asked questions
- Key concepts of QML
- QML videos
You can also check out our documentation for quickstart guides to using PennyLane, and detailed developer guides on how to write your own PennyLane-compatible quantum device.
Tutorials and demonstrations
Take a deeper dive into quantum machine learning by exploring cutting-edge algorithms on our demonstrations page.
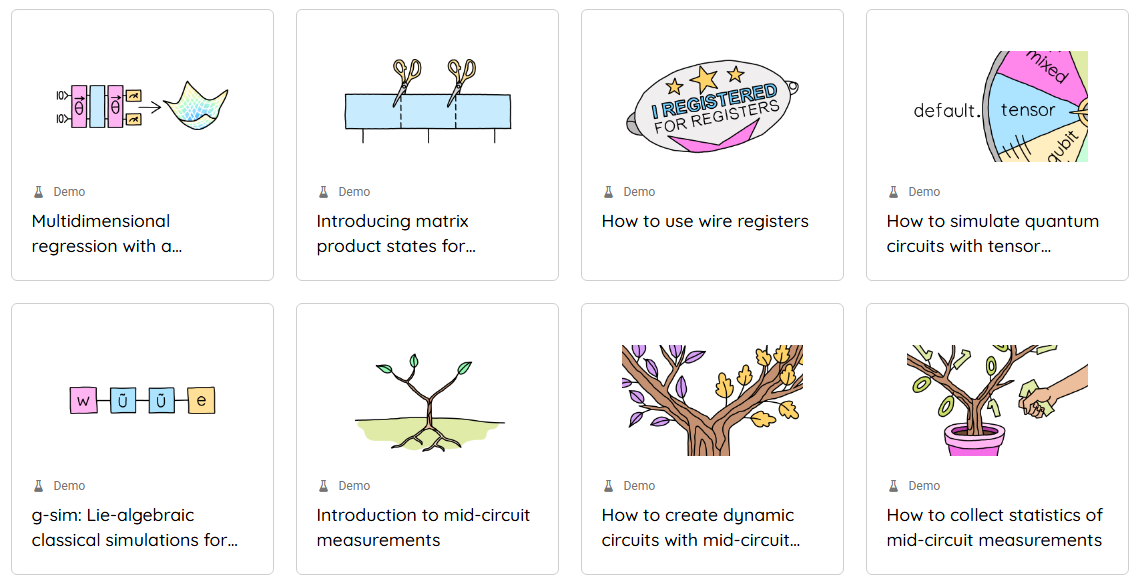
All demonstrations are fully executable, and can be downloaded as Jupyter notebooks and Python scripts.
If you would like to contribute your own demo, see our demo submission guide.
Contributing to PennyLane
We welcome contributions—simply fork the PennyLane repository, and then make a pull request containing your contribution. All contributors to PennyLane will be listed as authors on the releases. All users who contribute significantly to the code (new plugins, new functionality, etc.) will be listed on the PennyLane arXiv paper.
We also encourage bug reports, suggestions for new features and enhancements, and even links to cool projects or applications built on PennyLane.
See our contributions page and our developer hub for more details.
Support
- Source Code: https://github.com/PennyLaneAI/pennylane
- Issue Tracker: https://github.com/PennyLaneAI/pennylane/issues
If you are having issues, please let us know by posting the issue on our GitHub issue tracker.
We also have a PennyLane discussion forum—come join the community and chat with the PennyLane team.
Note that we are committed to providing a friendly, safe, and welcoming environment for all. Please read and respect the Code of Conduct.
Authors
PennyLane is the work of many contributors.
If you are doing research using PennyLane, please cite our paper:
Ville Bergholm, Josh Izaac, Maria Schuld, Christian Gogolin, M. Sohaib Alam, Shahnawaz Ahmed, Juan Miguel Arrazola, Carsten Blank, Alain Delgado, Soran Jahangiri, Keri McKiernan, Johannes Jakob Meyer, Zeyue Niu, Antal Száva, and Nathan Killoran. PennyLane: Automatic differentiation of hybrid quantum-classical computations. 2018. arXiv:1811.04968
License
PennyLane is free and open source, released under the Apache License, Version 2.0.