prashnani / Perceptualimageerror
Programming Languages
Projects that are alternatives of or similar to Perceptualimageerror
Perceptual Image Error Metric (PieAPP v0.1)
This is the repository for the "PieAPP" metric which measures the perceptual error of a distorted image with respect to a reference and the associated dataset.
Technical details about the metric can be found in our paper "PieAPP: Perceptual Image-Error Assessment through Pairwise Preference", published at CVPR 2018, and also on the project webpage. The directions to use the metric can be found in this repository.
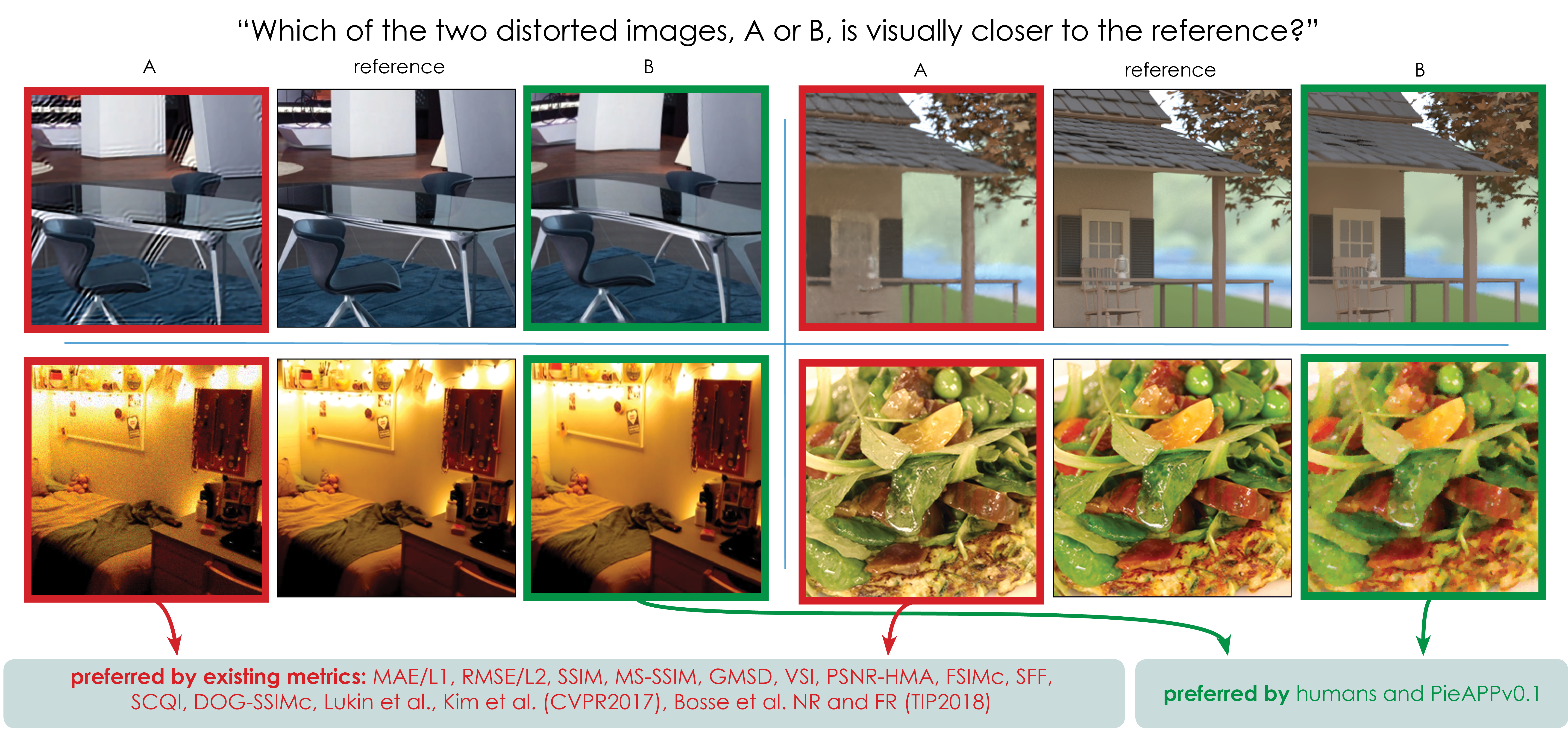
Using PieAPP
In this repo, we provide the Tensorflow and PyTorch implementations of our evaluation code for PieAPP v0.1 along with the trained models. We also provide a Win64 command-line executable.
Dependencies
The code uses Python 2.7, numpy, opencv and PyTorch 0.3.1 (tested with cuda 9.0; wheel can be found here) (files ending with PT) or Tensorflow 1.4 (files ending with TF).
Expected input and ouput
The input to PieAPPv0.1 are two images: a reference image, R, and a distorted image, A and the output is the PieAPP value of A with respect to R. PieAPPv0.1 outputs a number that quantifies the perceptual error of A with respect to R.
Since PieAPPv0.1 is computed based on a weighted combination of the patchwise errors, the number of patches extracted affects the speed and accuracy of the computed error. We have two modes of operation:
- "Dense" sampling: Selects 64x64 patches with a stride of 6 pixels for PieAPP computation (recommended for performance evaluation of PieAPP, for e.g., when comparing to other image error evaluation methods)
- "Sparse" sampling (default): Selects 64x64 patches with a stride of 27 pixels for PieAPP computation (recommended for high-speed processing, for example when used in a pipeline that requires fast execution time)
For large images, to avoid holding all sampled patches in memory, we recommend fetching patchwise errors and weights for sub-images followed by a weighted averaging of the patchwise errors to get the overall image error (see demo scripts test_PieAPP_TF.py
and test_PieAPP_PT.py
).
PieAPPv0.1 with Tensorflow:
Script test_PieAPP_TF.py
demonstrates the inference using Tensorflow.
Download trained model:
bash scripts/download_PieAPPv0.1_TF_weights.sh
Run the demo script:
python test_PieAPP_TF.py --ref_path <path to reference image> --A_path <path to distorted image> --sampling_mode <dense or sparse> --gpu_id <specify which GPU to use - don't specify this argument if using CPU only>
For example:
python test_PieAPP_TF.py --ref_path imgs/ref.png --A_path imgs/A.png --sampling_mode sparse --gpu_id 0
PieAPPv0.1 with PyTorch:
Script test_PieAPP_PT.py
demonstrates the inference using PyTorch.
Download trained model:
bash scripts/download_PieAPPv0.1_PT_weights.sh
Run the demo script:
python test_PieAPP_PT.py --ref_path <path to reference image> --A_path <path to distorted image> --sampling_mode <dense or sparse> --gpu_id <specify which GPU to use>
For example:
python test_PieAPP_PT.py --ref_path imgs/ref.png --A_path imgs/A.png --sampling_mode sparse --gpu_id 0
PieAPPv0.1 Win64 command-line executable:
We also provide a Win64 command-line executable for PieAPPv0.1. To use it, download the executable, open a Windows command prompt and run the following command:
PieAPPv0.1 --ref_path <path to reference image> --A_path <path to distorted image> --sampling_mode <sampling mode>
For example:
PieAPPv0.1 --ref_path imgs/ref.png --A_path imgs/A.png --sampling_mode sparse
The PieAPP dataset
The dataset subdirectory contains information about the PieAPP dataset, terms of usage, and links to downloading the dataset.
Citing PieAPPv0.1
@InProceedings{Prashnani_2018_CVPR,
author = {Prashnani, Ekta and Cai, Hong and Mostofi, Yasamin and Sen, Pradeep},
title = {PieAPP: Perceptual Image-Error Assessment Through Pairwise Preference},
booktitle = {The IEEE Conference on Computer Vision and Pattern Recognition (CVPR)},
month = {June},
year = {2018}
}
Acknowledgements
This project was supported in part by NSF grants IIS-1321168 and IIS-1619376, as well as a Fall 2017 AI Grant (awarded to Ekta Prashnani).