wywu / Reenactgan
Programming Languages
Labels
Projects that are alternatives of or similar to Reenactgan
ReenactGAN: Learning to Reenact Faces via Boundary Transfer
Created by Wayne Wu, Yunxuan Zhang and Ren Li.
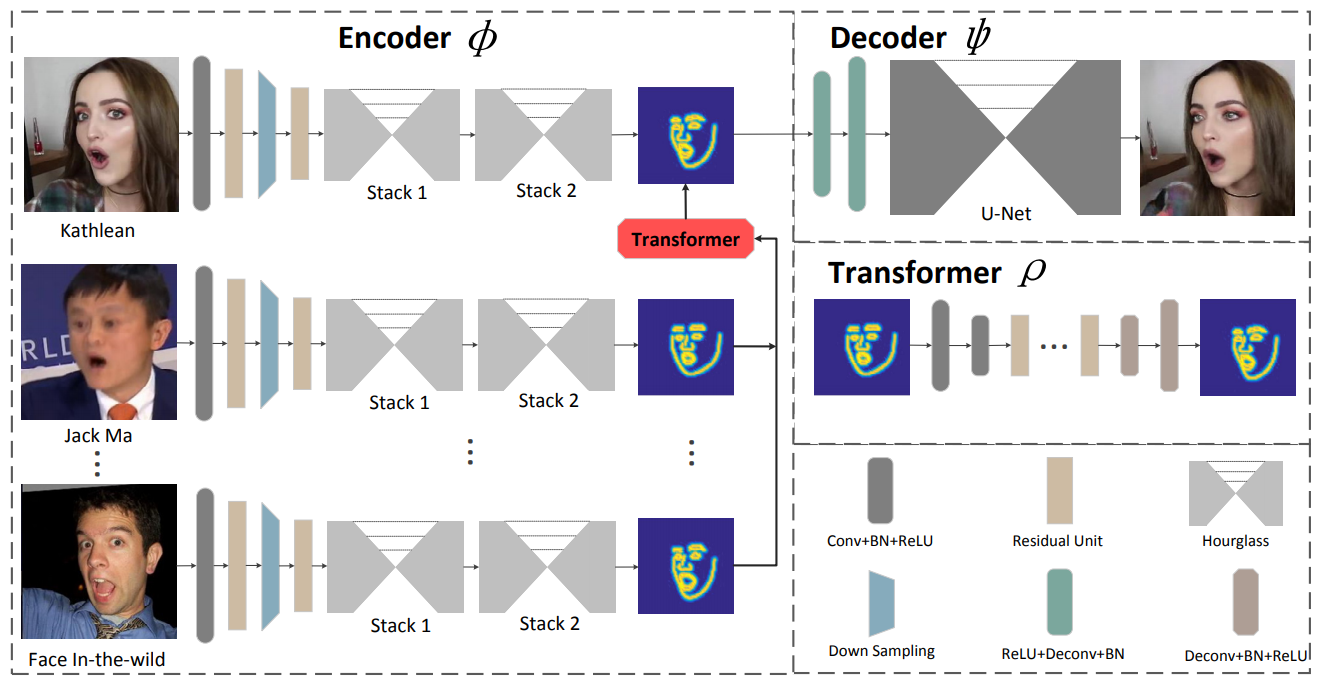
We present a novel learning-based framework for face reenactment. The proposed method, known as ReenactGAN, is capable of transferring facial movements and expressions from an arbitrary person’s monocular video input to a target person’s video. Instead of performing a direct transfer in the pixel space, which could result in structural artifacts, we first map the source face onto a boundary latent space. A transformer is subsequently used to adapt the source face’s boundary to the target’s boundary. Finally, a target-specific decoder is used to generate the reenacted target face. Thanks to the effective and reliable boundary-based transfer, our method can perform photo-realistic face reenactment. In addition, ReenactGAN is appealing in that the whole reenactment process is purely feed-forward, and thus the reenactment process can run in real-time.
Prerequisites
- Linux
- Python 3
- PyTorch 1.1.0 + CUDA 8.0 (Other versions may also work.)
- Dominate
Getting Started
Installing
Clone this repo:
git clone ...
cd ReenactGAN
Training
The bounday encoder is trained on WFLW and Helen dataset, and both of the boundary transformer and decoder are trained on CelebV Dataset. The training of the encoder requires a huge amount of time, so you can get the pretrained encoder at ./pretrained_models/v8_net_boundary_detection.pth.
To train the boundary transformer, run
sh script/train_Transformer.sh
You need to take care of the arguments --root_dir and --which_target. --root_dir refers to the directory of the dataset, and --which_target refers to which person to be the target
0: Emmanuel_Macron
1: Kathleen
2: Jack_Ma
3: Theresa_May
4: Donald_Trump
To train the decoder, run
sh script/train_Decoder.sh
Also, you need to take care of the value of --root_dir, which refers to the directory of the target person.
Testing
To test the model, run
sh script/move_models.sh ./checkpoints/Transformer_2019-xx-xx_xx-xx-xx/G_BA_xx.pth ./checkpoints/Decoder_2019-xx-xx_xx-xx-xx/xx_net_G.pth trump
sh script/test.sh
The images used for testing is at ./test_imgs/samples/image, and the text file, ./test_imgs/samples/images_list.txt, contains the list of these images. After the testing, you will get a floder named results, which contains the images of the real and reenacted faces, the boundaries and the transformed boundaries of the real faces. Here are some results.
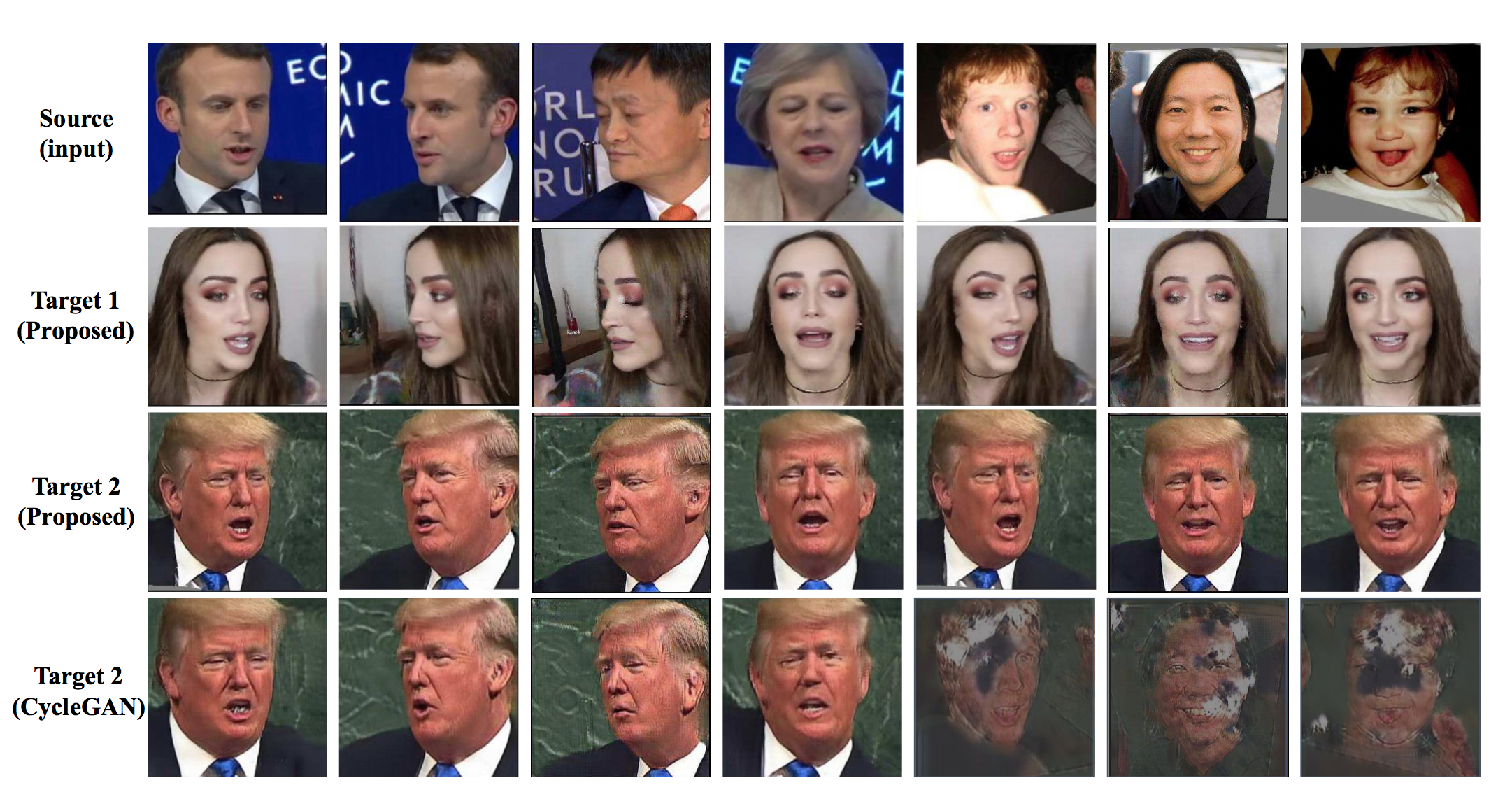
You can get our trained models from Decoder and Transformer.
Citation
If you find ReenactGAN helpful for your research, please cite:
inproceedings{wayne2018reenactgan,
author = {Wu, Wayne and Zhang, Yunxuan and Li, Cheng and Qian, Chen and Loy, Chen Change},
title = {ReenactGAN: Learning to Reenact Faces via Boundary Transfer},
booktitle = {ECCV},
month = September,
year = {2018}
}
Questions
Please contact [email protected]