jiangsutx / Srn Deblur
Programming Languages
Projects that are alternatives of or similar to Srn Deblur
Scale-recurrent Network for Deep Image Deblurring
by Xin Tao, Hongyun Gao, Xiaoyong Shen, Jue Wang, Jiaya Jia. (pdf)
Our results on real data
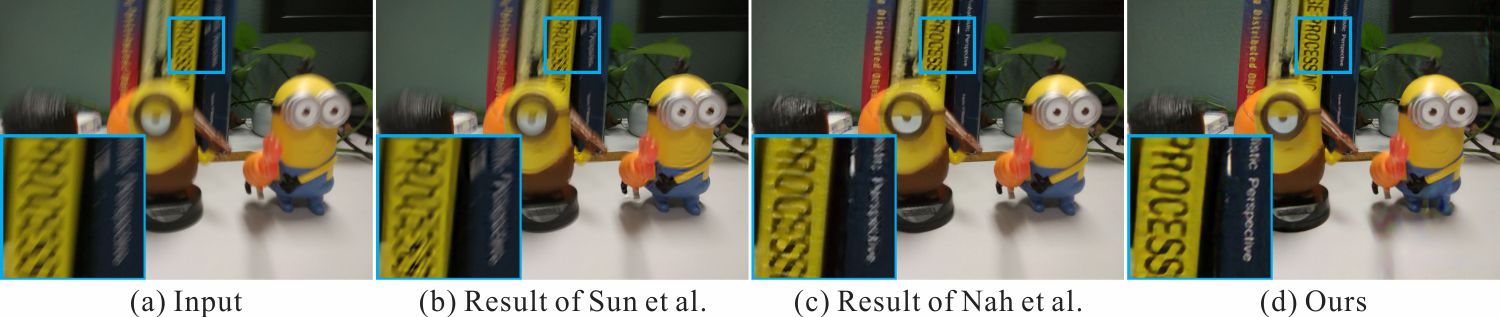
Results on the testing dataset
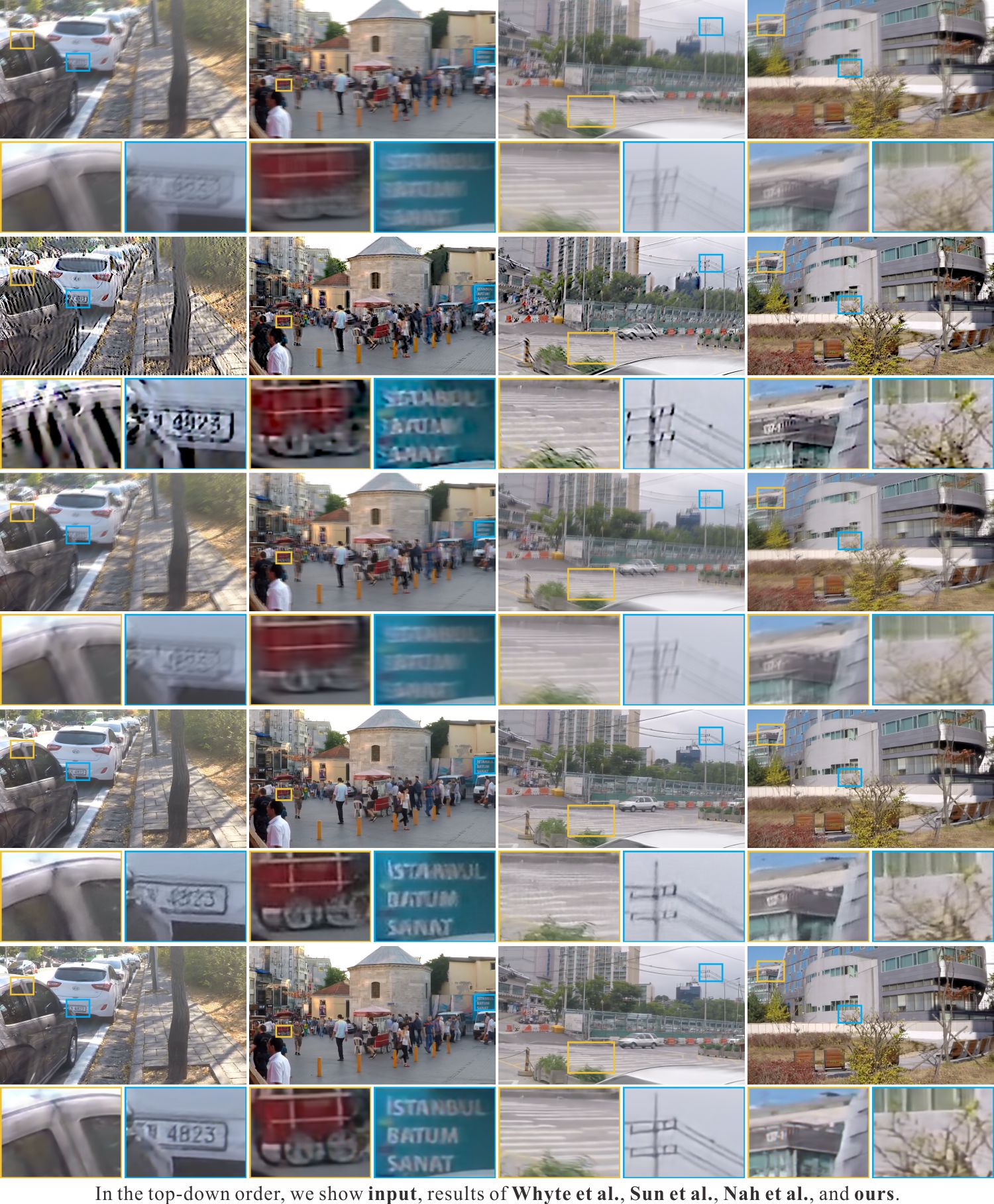
More cases on real photos from previous papers:
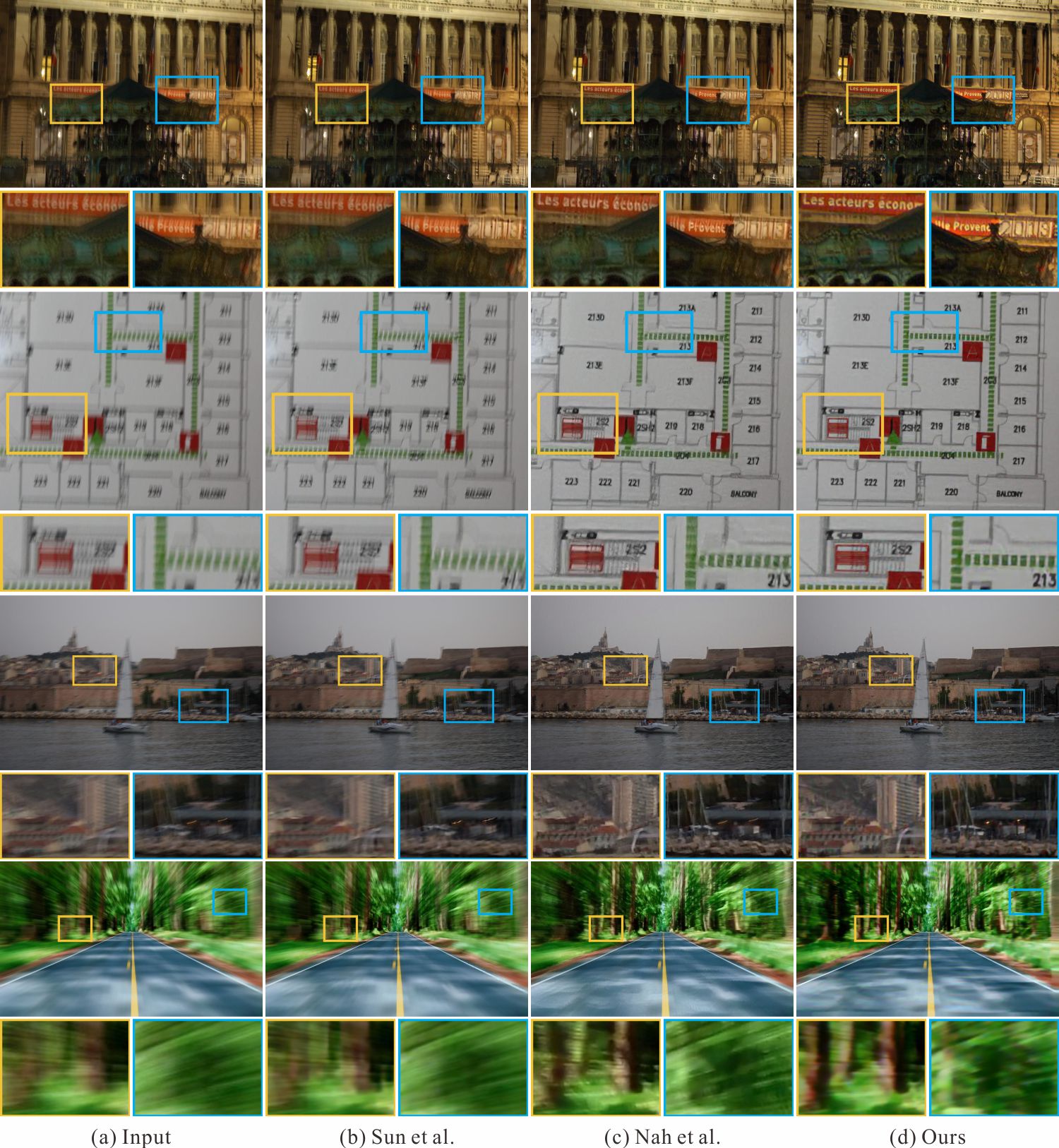
Prerequisites
- Python2.7
- Scipy
- Scikit-image
- numpy
- Tensorflow 1.4 with NVIDIA GPU or CPU (cpu testing is very slow)
Installation
Clone this project to your machine.
git clone https://github.com/jiangsutx/SRN-Deblur.git
cd SRN-Deblur
Testing
Download pretrained models through: download_model.sh
inside checkpoints/
.
To test blur images in a folder, just use arguments
--input_path=<TEST_FOLDER>
and save the outputs to --output_path=<OUTPUT_FOLDER>
.
For example:
python run_model.py --input_path=./testing_set --output_path=./testing_res
If you have a GPU, please include --gpu
argument, and add your gpu id to your command.
Otherwise, use --gpu=-1
for CPU.
python run_model.py --gpu=0
To test the model, pre-defined height and width of tensorflow
placeholder should be assigned.
Our network requires the height and width be multiples of 16
.
When the gpu memory is enough, the height and width could be assigned to
the maximum to accommodate all the images.
Otherwise, the images will be downsampled by the largest scale factor to be fed into the placeholder. And results will be upsampled to the original size.
According to our experience, --height=720
and --width=1280
work well
on a Gefore GTX 1050 TI with 4GB memory. For example,
python run_model.py --height=720 --width=1280
Evaluation
The quantitative results of PSNR and SSIM in the paper is
calculated using MATLAB built-in function psnr()
and ssim()
based
on the generated color results.
Training
We trained our model using the dataset from
DeepDeblur_release.
Please put the dataset into training_set/
. And the provided datalist_gopro.txt
can be used to train the model.
Hyper parameters such as batch size, learning rate, epoch number can be tuned through command line:
python run_model.py --phase=train --batch=16 --lr=1e-4 --epoch=4000
Models
We provided 3 models (training settings) for testing:
-
--model=lstm
: This model implements exactly the same structure in our paper. Current released model weights should producePSNR=30.19, SSIM=0.9334
on GOPRO testing dataset. -
--model=gray
: According to our further experiments after paper acceptance, we are able to get a slightly better model by tuning parameters, even without LSTM. This model should produce visually sharper and quantitatively better results. -
--model=color
: Previous models are trained on gray images, and may produce color ringing artifacts. So we train a model directly based on RGB images. This model keeps better color consistency, but the results are less sharp.
How to choose
If you would like to compare performance against our method, you can use
model gray
and lstm
.
If you want to restore blurry images you can try gray
and color
.
And color
is very useful in low-light noisy images.
Reference
If you use any part of our code, or SRN-Deblur is useful for your research, please consider citing:
@inproceedings{tao2018srndeblur,
title={Scale-recurrent Network for Deep Image Deblurring},
author={Tao, Xin and Gao, Hongyun and Shen, Xiaoyong and Wang, Jue and Jia, Jiaya},
booktitle={IEEE Conference on Computer Vision and Pattern Recognition (CVPR)},
year={2018}
}
Contact
We are glad to hear if you have any suggestions and questions.
Please send email to [email protected]
Reference
[1] Sun et al.
J. Sun, W. Cao, Z. Xu, and J. Ponce. Learning a convolutional
neural network for non-uniform motion blur removal. In CVPR, pages 769–777. IEEE, 2015.
[2] Nah et al.
S. Nah, T. H. Kim, and K. M. Lee. Deep multi-scale convolutional
neural network for dynamic scene deblurring. pages 3883–3891, 2017.
[3] Whyte et al.
O. Whyte, J. Sivic, A. Zisserman, and J. Ponce. Nonuniform
deblurring for shaken images. International Journal on Computer Vision, 98(2):168–186, 2012.