cschwem2er / Stminsights
Programming Languages
Projects that are alternatives of or similar to Stminsights
stminsights
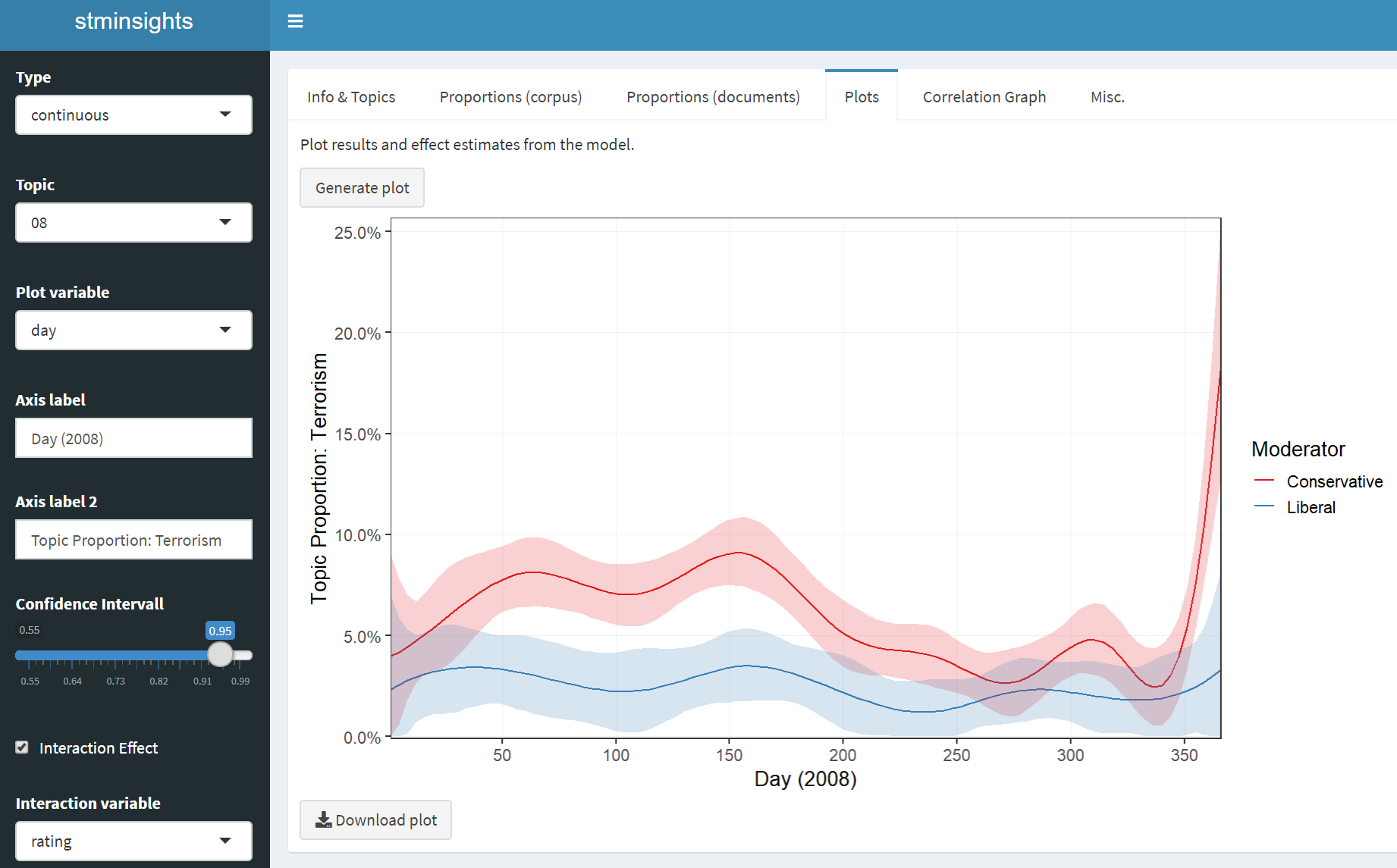
A Shiny Application for Structural Topic Models
This app enables interactive validation, interpretation and visualisation of Structural Topic Models (STM). Stminsights is focused on making your life easier after fitting your STM models. In case you are not familiar with the STM package, the corresponding vignette is an excellent starting point.
How to Install
You can download and install the latest development version of
stminsights by running
devtools::install_github('cschwem2er/stminsights')
.
For Windows users installing from github requires proper setup of Rtools.
stminsights can also be installed from CRAN by running
install.packages('stminsights')
.
How to Use
After loading stminsights you can launch the shiny app in your browser:
library(stminsights)
run_stminsights()
You can then upload a .RData
file which should include:
- one or several
stm
objects. - one or several
estimateEffect
objects. - an object
out
which was used to fit your stm models.
As an example, the following code fits two models and estimates effects
for the Political Blog Corpus. Afterwards, all objects required for
stminsights are stored in stm_poliblog5k.RData
.
library(stm)
out <- list(documents = poliblog5k.docs,
vocab = poliblog5k.voc,
meta = poliblog5k.meta)
poli <- stm(documents = out$documents,
vocab = out$vocab,
data = out$meta,
prevalence = ~ rating * s(day),
K = 20)
prep_poli <- estimateEffect(1:20 ~ rating * s(day), poli,
meta = out$meta)
poli_content <- stm(documents = out$documents,
vocab = out$vocab,
data = out$meta,
prevalence = ~ rating + s(day),
content = ~ rating,
K = 15)
prep_poli_content <- estimateEffect(1:15 ~ rating + s(day), poli_content,
meta = out$meta)
save.image('stm_poliblog5k.RData')
After launching stminsights and uploading the file, all objects are automatically imported and you can select which models and effect estimates to analyze.
In addition to the shiny app, several helper functions are available,
e.g. get_effects()
for storing effect estimates in a tidy dataframe.
How to Deploy on Shiny Server
Deploying stminsights to your own shiny server is simple: Place the file
app.R
, which is located at inst/app
of this package, to a folder in
your server directory and you should be good to go.
Citation
Please cite stminsights if you use it for your publications:
Carsten Schwemmer (2018). stminsights: A Shiny Application for Inspecting
Structural Topic Models. R package version 0.4.0.
https://github.com/cschwem2er/stminsights
A BibTeX entry for LaTeX users is:
@Manual{,
title = {stminsights: A Shiny Application for Inspecting Structural Topic Models},
author = {Carsten Schwemmer},
year = {2018},
note = {R package version 0.4.0},
url = {https://github.com/cschwem2er/stminsights},
}