siemanko / Tensorflow Deepq
Labels
Projects that are alternatives of or similar to Tensorflow Deepq
This reposity is now obsolte!
Check out the new simpler, better performing and more complete implementation that we released at OpenAI:
https://github.com/openai/baselines
(scroll for docs of the obsolete version)
Reinforcement Learning using Tensor Flow
Quick start
Check out Karpathy game in notebooks
folder.
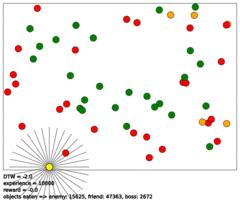
The image above depicts a strategy learned by the DeepQ controller. Available actions are accelerating top, bottom, left or right. The reward signal is +1 for the green fellas, -1 for red and -5 for orange.
Requirements
future==0.15.2
euclid==0.1
-
inkscape
(for animation gif creation)
How does this all fit together.
tf_rl
has controllers and simulators which can be pieced together using simulate function.
Using human controller.
Want to have some fun controlling the simulation by yourself? You got it!
Use tf_rl.controller.HumanController
in your simulation.
To issue commands run in terminal
python3 tf_rl/controller/human_controller.py
For it to work you also need to have a redis server running locally.
Writing your own controller
To write your own controller define a controller class with 3 functions:
-
action(self, observation)
given an observation (usually a tensor of numbers) representing an observation returns action to perform. -
store(self, observation, action, reward, newobservation)
called each time a transition is observed fromobservation
tonewobservation
. Transition is a consequence ofaction
and has associatedreward
-
training_step(self)
if your controller requires training that is the place to do it, should not take to long, because it will be called roughly every action execution.
Writing your own simulation
To write your own simulation define a simulation class with 4 functions:
-
observe(self)
returns a current observation -
collect_reward(self)
returns the reward accumulated since the last time function was called. -
perform_action(self, action)
updates internal state to reflect the fact thataciton
was executed -
step(self, dt)
update internal state as ifdt
of simulation time has passed. -
to_html(self, info=[])
generate an html visualization of the game.info
can be optionally passed an has a list of strings that should be displayed along with the visualization
Creating GIFs based on simulation
The simulate
method accepts save_path
argument which is a folder where all the consecutive images will be stored.
To make them into a GIF use scripts/make_gif.sh PATH
where path is the same as the path you passed to save_path
argument