junyanz / Von
Programming Languages
Projects that are alternatives of or similar to Von
Visual Object Networks
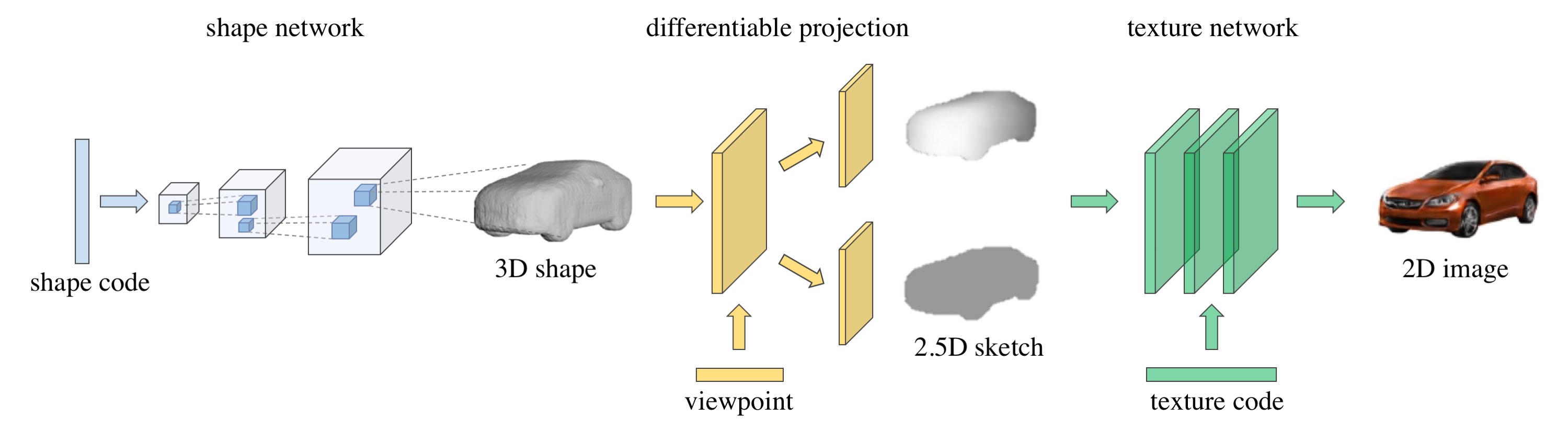
We present Visual Object Networks (VON), an end-to-end adversarial learning framework that jointly models 3D shapes and 2D images. Our model can synthesize a 3D shape, its intermediate 2.5D depth representation, and a 2D image all at once. The VON not only generates realistic images but also enables several 3D operations.
Visual Object Networks: Image Generation with Disentangled 3D Representation.
Jun-Yan Zhu,
Zhoutong Zhang, Chengkai Zhang, Jiajun Wu, Antonio Torralba, Joshua B. Tenenbaum, William T. Freeman.
MIT CSAIL and Google Research.
In NeurIPS 2018.
Example results
(a) Typical examples produced by a recent GAN model [Gulrajani et al., 2017].
(b) Our model produces three outputs: a 3D shape, its 2.5D projection given a viewpoint, and a final image with realistic texture.
(c) Our model allows several 3D applications including editing viewpoint, shape, or texture independently.
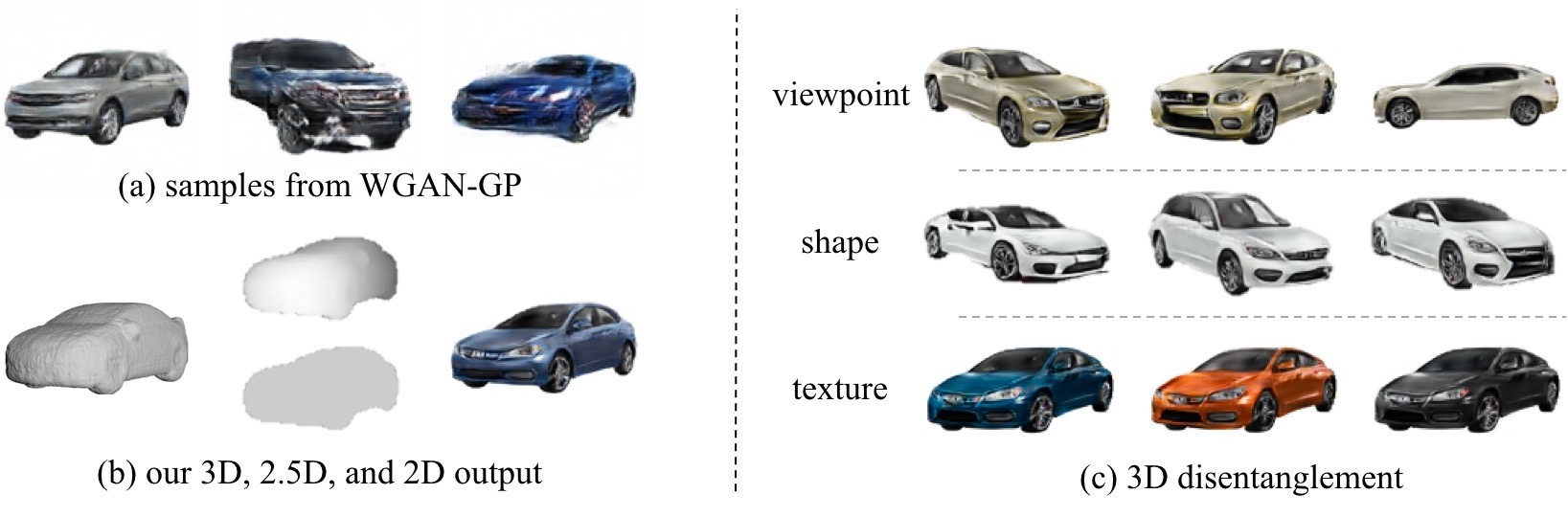
More samples
Below we show more samples from DCGAN [Radford et al., 2016], LSGAN [Mao et al., 2017], WGAN-GP [Gulrajani et al., 2017], and our VON. For our method, we show both 3D shapes and 2D images. The learned 3D prior helps produce better samples.
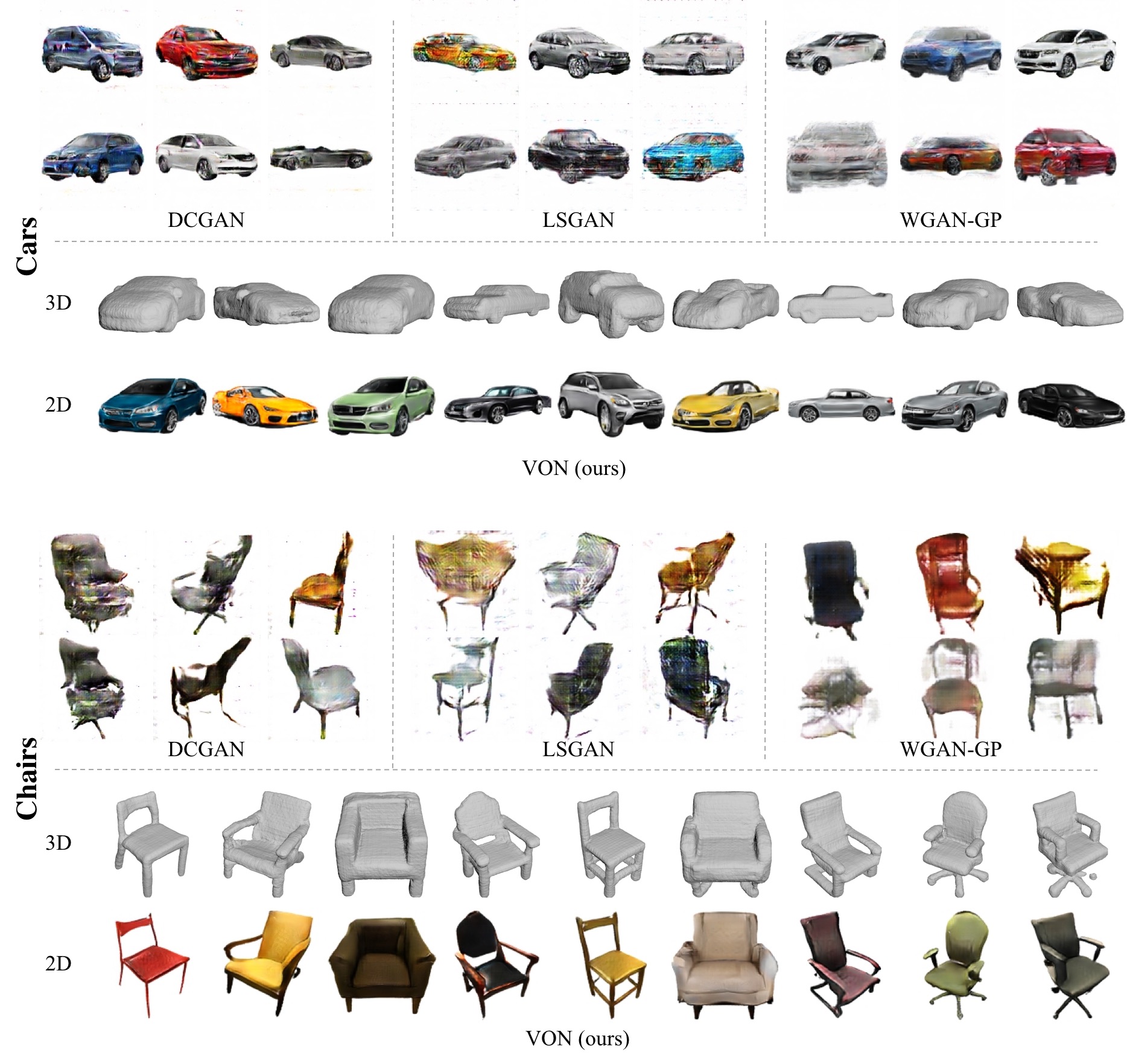
3D object manipulations
Our VON allows several 3D applications such as (left) changing the viewpoint, texture, or shape independently, and (right) interpolating between two objects in shape space, texture space, or both.
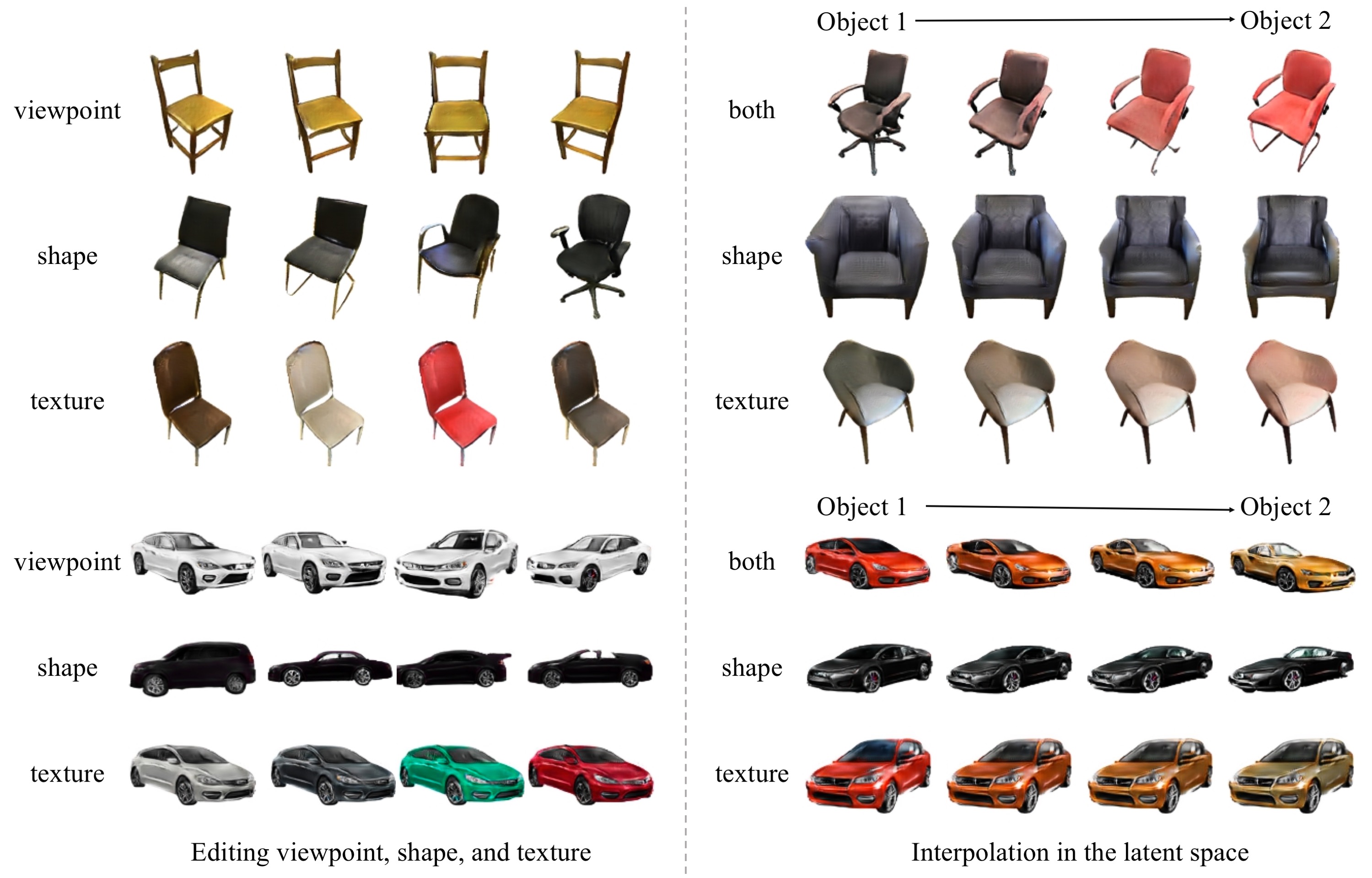
Transfer texture across objects and viewpoints
VON can transfer the texture of a real image to different shapes and viewpoints
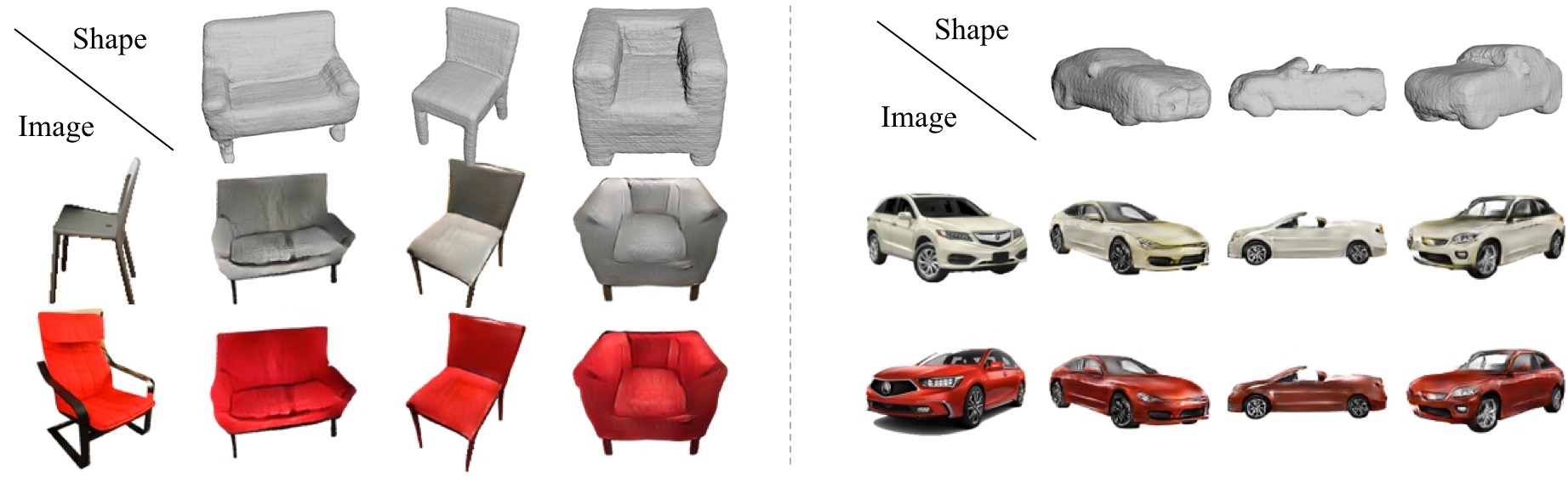
Prerequisites
- Linux (only tested on Ubuntu 16.04)
- Python3 (only tested with python 3.6)
- Anaconda3
- NVCC & GCC (only tested with gcc 6.3.0)
- PyTorch 0.4.1 (does not support 0.4.0)
- Currently not tested with Nvidia RTX GPU series
- Docker Engine and Nvidia-Docker2 if using Docker container.
Getting Started
Installation
- Clone this repo:
git clone -b master --single-branch https://github.com/junyanz/VON.git
cd VON
- Install PyTorch 0.4.1+ and torchvision from http://pytorch.org and other dependencies (e.g., visdom and dominate). You can install all the dependencies by the following:
conda create --name von --file pkg_specs.txt
source activate von
- Compile our rendering kernel by running the following:
bash install.sh
We only test this step with gcc 6.3.0
. If you need to recompile the kernel, please run bash clean.sh
first before you recompile it.
- If you can not compile the custom kernels, we provide a Dockerfile for building a working container. To use the Dockerfile, you need to install Docker Engine and Nvidia-Docker2 for using Nvidia GPUs inside the docker container. To build the docker image, run:
sudo docker build ./../von -t von
To access the container, run:
sudo docker run -it --runtime=nvidia --ipc=host von /bin/bash
Then, to compile the kernels, simply:
cd /app/von
source activate von
./install.sh
- (Optional) Install blender for visualizing generated 3D shapes. After installation, please add blender to the PATH environment variable.
Generate 3D shapes, 2.5D sketches, and images
- Download our pretrained models:
bash ./scripts/download_model.sh
- Generate results with the model
bash ./scripts/figures.sh 0 car df
The test results will be saved to an HTML file here: ./results/*/*/index.html
.
Model Training
- To train a model, download the training dataset (distance functions and images). For example, if we would like to train a car model with distance function representation on GPU 0.
bash ./scripts/download_dataset.sh
- To train a 3D generator:
bash ./scripts/train_shape.sh 0 car df
- To train a 2D texture network using ShapeNet real shapes:
bash ./scripts/train_texture_real.sh 0 car df 0
- To train a 2D texture network using pre-trained 3D generator:
bash ./scripts/train_texture.sh 0 car df 0
- Jointly finetune 3D and 2D generative models:
bash ./scripts/train_full.sh 0 car df 0
- To view training results and loss plots, go to http://localhost:8097 in a web browser. To see more intermediate results, check out
./checkpoints/*/web/index.html
Citation
If you find this useful for your research, please cite the following paper.
@inproceedings{VON,
title={Visual Object Networks: Image Generation with Disentangled 3{D} Representations},
author={Jun-Yan Zhu and Zhoutong Zhang and Chengkai Zhang and Jiajun Wu and Antonio Torralba and Joshua B. Tenenbaum and William T. Freeman},
booktitle={Advances in Neural Information Processing Systems (NeurIPS)},
year={2018}
}
Acknowledgements
This work is supported by NSF #1231216, NSF #1524817, ONR MURI N00014-16-1-2007, Toyota Research Institute, Shell, and Facebook. We thank Xiuming Zhang, Richard Zhang, David Bau, and Zhuang Liu for valuable discussions. This code borrows from the CycleGAN & pix2pix repo.