MIC-DKFZ / Trixi
Programming Languages
Labels
Projects that are alternatives of or similar to Trixi
Finally get some structure into your machine learning experiments. trixi (Training & Retrospective Insights eXperiment Infrastructure) is a tool that helps you configure, log and visualize your experiments in a reproducible fashion.
Contribute
We're always grateful for contributions, even small ones! We're PhD students and this is just a side project, so there will always be something to improve.
The best way is to create pull requests on Github. Fork the repository and work either directly on develop or create a feature branch, whichever you like best. Then go to "Pull requests" on our Github, select "New pull request" and "compare across forks". Select our develop as base and your work as head/compare.
We currently don't support the full Github workflow, because we have to mirror from our working repository to Github, but don't worry, we can export the pull requests and apply them so that your contribution will still appear on Github :)
Features
trixi consists of three parts:
-
Logging API
Log whatever data you like in whatever way you like to whatever backend you like. -
Experiment Infrastructure
Standardize your experiment, let the framework do all the inconvenient stuff, and simply start, resume, change and finetune all your experiments. -
Experiment Browser
Compare, combine and visually inspect the results of your experiments.
An implementation diagram is given here.
Logging API
The Logging API provides a standardized way for logging results to different backends. The Logging API supports (among others):
- Values
- Text
- Plots (Bar, Line, Scatter, Piechart, ...)
- Images (Single, Grid)
And offers different Backends, e.g. :
- Visdom (visdom-loggers)
- Tensorboard (tensorboard-loggers)
- Matplotlib / Seaborn (plt-loggers)
- Local Disk (file-loggers)
- Telegram & Slack (message-loggers)
And an experiment-logger for logging your experiments, which uses a file logger to automatically create a structured directory and allows storing of config, results, plots, dict, array, images, etc. That way your experiments will always have the same structure on disk.
Here are some examples:
- Files:
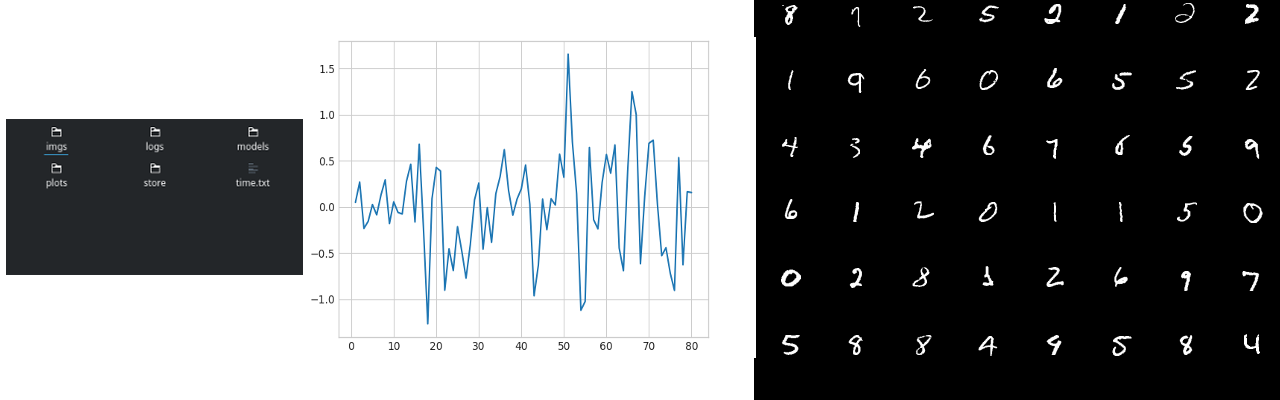
- Telegram:
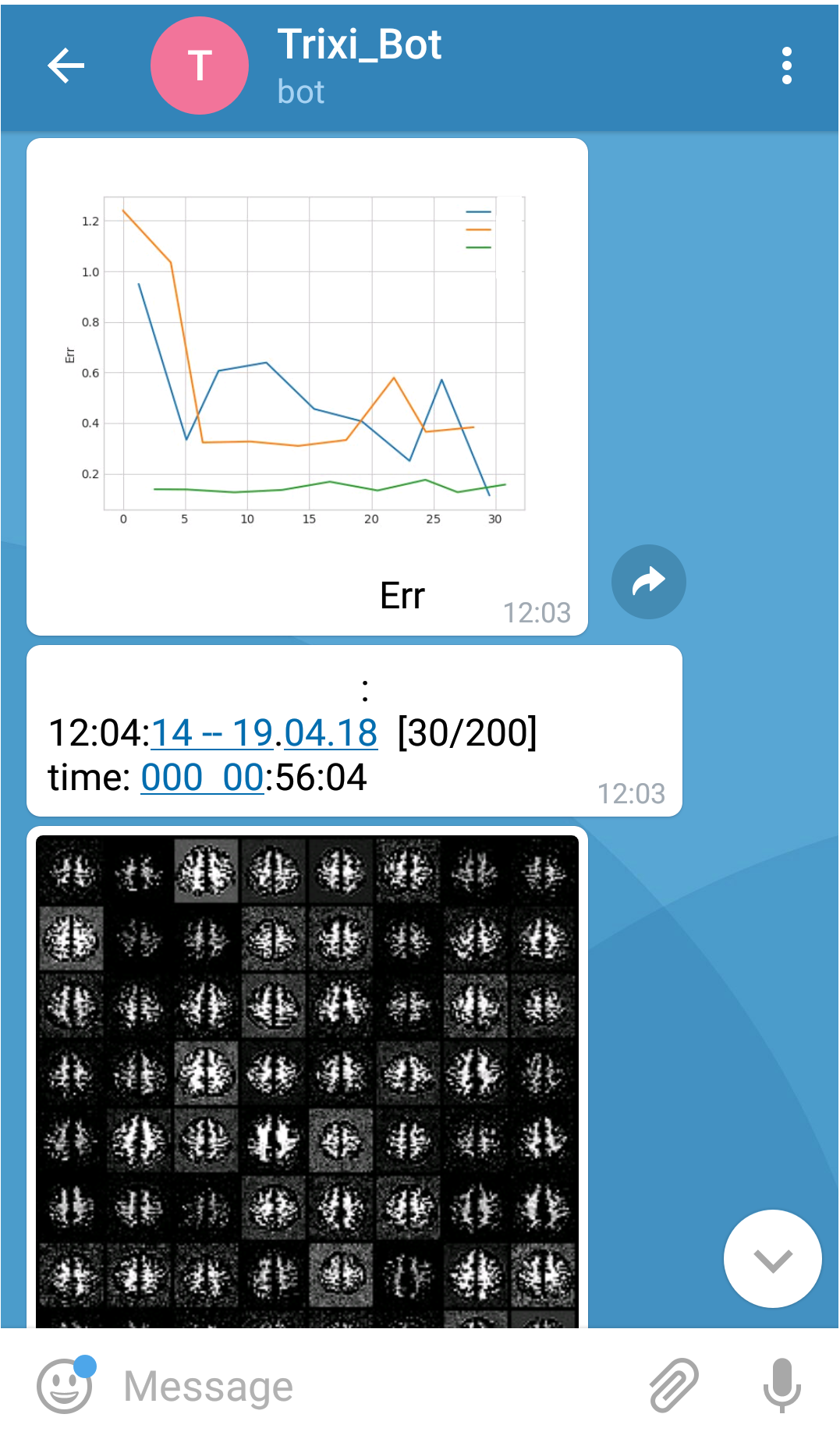
Experiment Infrastructure
The Experiment Infrastructure provides a unified way to configure, run, store and evaluate your results. It gives you an experiment interface, for which you can implement the training, validation and testing. Furthermore it automatically provides you with easy access to the Logging API and stores your config as well as the results for easy evaluation and reproduction. There is an abstract Experiment class and a PytorchExperiment with many convenience features.
For more info, visit the Documentation.
Experiment Browser
(We're currently remaking this from scratch, expect major improvements :))
The Experiment Browser offers a complete overview of experiments along with all config parameters and results.
It also allows to combine and/or compare different experiments, giving you an interactive comparison highlighting differences in the configs and a detailed view of all images,
plots, results and logs of each experiment, with live plots and more.
Installation
Install trixi:
pip install trixi
Or to always get the newest version you can install trixi directly via git:
git clone https://github.com/MIC-DKFZ/trixi.git
cd trixi
pip install -e .
Documentation
The docs can be found here: trixi.rtfd.io
Or you can build your own docs using Sphinx.
Sphinx Setup
Install Sphinx (fixed to 1.7.0 for now because of issues with Readthedocs):
pip install sphinx==1.7.0
Generate HTML:
path/to/PROJECT/doc$ make html
index.html will be at:
path/to/PROJECT/doc/_build/html/index.html
Notes
- Rerun
make html
each time existing modules are updated (this will automatically call sphinx-apidoc) - Do not forget indent or blank lines
- Code with no classes or functions is not automatically captured using apidoc
Example Documentation
We use Google style docstrings:
def show_image(self, image, name, file_format=".png", **kwargs):
"""
This function shows an image.
Args:
image(np.ndarray): image to be shown
name(str): image title
"""
Examples
Examples can be found here for:
- Visdom-Logger
- Experiment-Logger
- Experiment Infrastructure (with a simple MNIST Experiment example and resuming and comparison of different hyperparameters)
- U-Net Example
How to Cite
If you use trixi in your project, we'd appreciate a citation, for example like this
@misc{trixi2017,
author = {Zimmerer, David and Petersen, Jens and Köhler, Gregor and Wasserthal, Jakob and Adler, Tim and Wirkert, Sebastian and Ross, Tobias},
title = {trixi - Training and Retrospective Insight eXperiment Infrastructure},
year = {2017},
publisher = {GitHub},
journal = {GitHub Repository},
howpublished = {\url{https://github.com/MIC-DKFZ/trixi}},
doi = {10.5281/zenodo.1345136}
}