hugochan / Bamnet
Programming Languages
Projects that are alternatives of or similar to Bamnet
BAMnet
Code & data accompanying the NAACL2019 paper "Bidirectional Attentive Memory Networks for Question Answering over Knowledge Bases"
Get started
Prerequisites
This code is written in python 3. You will need to install a few python packages in order to run the code.
We recommend you to use virtualenv
to manage your python packages and environments.
Please take the following steps to create a python virtual environment.
- If you have not installed
virtualenv
, install it withpip install virtualenv
. - Create a virtual environment with
virtualenv venv
. - Activate the virtual environment with
source venv/bin/activate
. - Install the package requirements with
pip install -r requirements.txt
.
Run the KBQA system
-
Download the preprocessed data from here and put the data folder under the root directory.
-
Create a folder (e.g.,
runs/WebQ/
) to save model checkpoint. You can download the pretrained models from here. (Note: if you cannot access the above data and pretrained models, please download from here.) -
Please modify the config files in the
src/config/
folder to suit your needs. Note that you can start with modifying only the data folder (e.g.,data_dir
,model_file
,pre_word2vec
) and vocab size (e.g.,vocab_size
,num_ent_types
,num_relations
), and leave other hyperparameters as they are. -
Go to the
BAMnet/src
folder, train the BAMnet modelpython train.py -config config/bamnet_webq.yml
-
Test the BAMnet model (with ground-truth topic entity)
python test.py -config config/bamnet_webq.yml
-
Train the topic entity predictor
python train_entnet.py -config config/entnet_webq.yml
-
Test the topic entity predictor
python test_entnet.py -config config/entnet_webq.yml
-
Test the whole system (BAMnet + topic entity predictor)
python joint_test.py -bamnet_config config/bamnet_webq.yml -entnet_config config/entnet_webq.yml -raw_data ../data/WebQ
Preprocess the dataset on your own
-
Go to the
BAMnet/src
folder, to prepare data for the BAMnet model, run the following cmd:python build_all_data.py -data_dir ../data/WebQ -fb_dir ../data/WebQ -out_dir ../data/WebQ
-
To prepare data for the topic entity predictor model, run the following cmd:
python build_all_data.py -dtype ent -data_dir ../data/WebQ -fb_dir ../data/WebQ -out_dir ../data/WebQ
Note that in the message printed out, your will see some data statistics such as vocab_size
, num_ent_types
, num_relations
. These numbers will be used later when modifying the config files.
-
Download the pretrained Glove word ebeddings glove.840B.300d.zip.
-
Unzip the file and convert glove format to word2vec format using the following cmd:
python -m gensim.scripts.glove2word2vec --input glove.840B.300d.txt --output glove.840B.300d.w2v
-
Fetch the pretrained Glove vectors for our vocabulary.
python build_pretrained_w2v.py -emb glove.840B.300d.w2v -data_dir ../data/WebQ -out ../data/WebQ/glove_pretrained_300d_w2v.npy -emb_size 300
Architecture
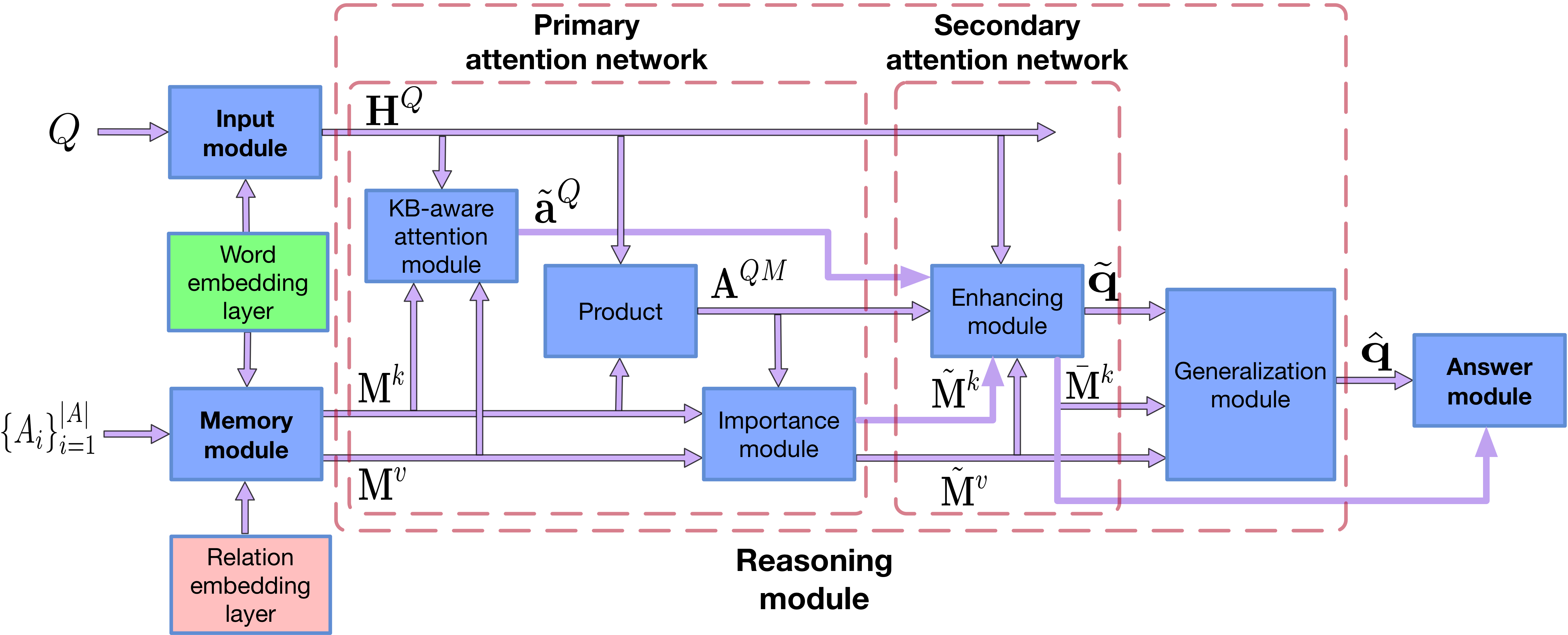
Experiment results on WebQuestions
Results on WebQuestions test set. Bold: best in-category performance.
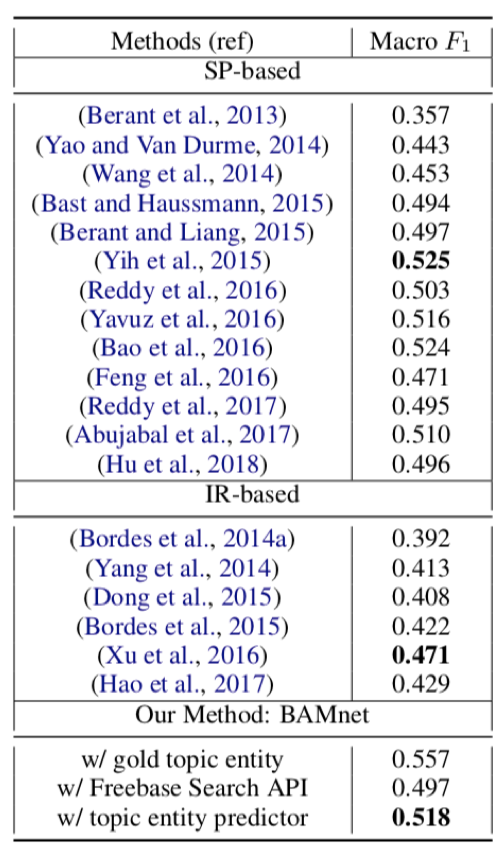
Predicted answers of BAMnet w/ and w/o bidirectional attention on the WebQuestions test set
Attention heatmap generated by the reasoning module
Reference
If you found this code useful, please consider citing the following paper:
Yu Chen, Lingfei Wu, Mohammed J. Zaki. "Bidirectional Attentive Memory Networks for Question Answering over Knowledge Bases." In Proc. 2019 Annual Conference of the North American Chapter of the Association for Computational Linguistics (NAACL-HLT2019). June 2019.
@article{chen2019bidirectional,
title={Bidirectional Attentive Memory Networks for Question Answering over Knowledge Bases},
author={Chen, Yu and Wu, Lingfei and Zaki, Mohammed J},
journal={arXiv preprint arXiv:1903.02188},
year={2019}
}