tidymodels / Tidypredict
Programming Languages
Labels
Projects that are alternatives of or similar to Tidypredict
The main goal of tidypredict
is to enable running predictions inside
databases. It reads the model, extracts the components needed to
calculate the prediction, and then creates an R formula that can be
translated into SQL. In other words, it is able to parse a model such as
this one:
model <- lm(mpg ~ wt + cyl, data = mtcars)
tidypredict
can return a SQL statement that is ready to run inside the
database. Because it uses dplyr
’s database interface, it works with
several databases back-ends, such as MS SQL:
tidypredict_sql(model, dbplyr::simulate_mssql())
## <SQL> 39.6862614802529 + (`wt` * -3.19097213898374) + (`cyl` * -1.5077949682598)
Installation
Install tidypredict
from CRAN using:
# install.packages("tidypredict")
Or install the development version using devtools
as follows:
# install.packages("remotes")
# remotes::install_github("tidymodels/tidypredict")
Functions
tidypredict
has only a few functions, and it is not expected that
number to grow much. The main focus at this time is to add more models
to support.
Function | Description |
---|---|
tidypredict_fit() |
Returns an R formula that calculates the prediction |
tidypredict_sql() |
Returns a SQL query based on the formula from tidypredict_fit()
|
tidypredict_to_column() |
Adds a new column using the formula from tidypredict_fit()
|
tidypredict_test() |
Tests tidyverse predictions against the model’s native predict() function |
tidypredict_interval() |
Same as tidypredict_fit() but for intervals (only works with lm and glm ) |
tidypredict_sql_interval() |
Same as tidypredict_sql() but for intervals (only works with lm and glm ) |
parse_model() |
Creates a list spec based on the R model |
as_parsed_model() |
Prepares an object to be recognized as a parsed model |
How it works
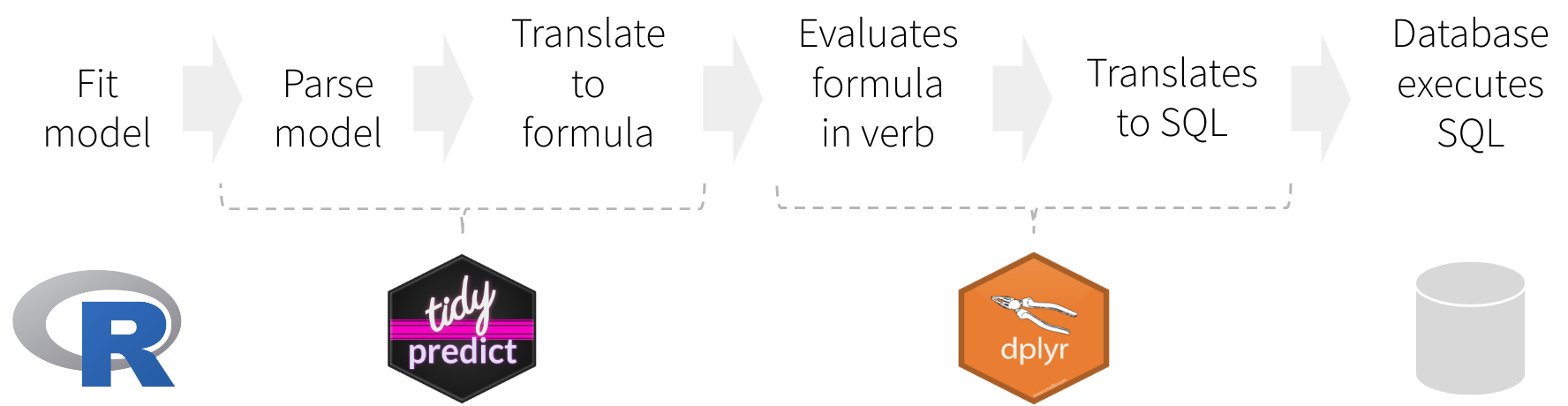
Instead of translating directly to a SQL statement, tidypredict
creates an R formula. That formula can then be used inside dplyr
. The
overall workflow would be as illustrated in the image above, and
described here:
- Fit the model using a base R model, or one from the packages listed in Supported Models
-
tidypredict
reads model, and creates a list object with the necessary components to run predictions -
tidypredict
builds an R formula based on the list object -
dplyr
evaluates the formula created bytidypredict
-
dplyr
translates the formula into a SQL statement, or any other interfaces. - The database executes the SQL statement(s) created by
dplyr
Parsed model spec
tidypredict
writes and reads a spec based on a model. Instead of
simply writing the R formula directly, splitting the spec from the
formula adds the following capabilities:
- No more saving models as
.rds
- Specifically for cases when the model needs to be used for predictions in a Shiny app. - Beyond R models - Technically, anything that can write a proper
spec, can be read into
tidypredict
. It also means, that the parsed model spec can become a good alternative to using PMML.
Supported models
The following models are supported by tidypredict
:
- Linear Regression -
lm()
- Generalized Linear model -
glm()
- Random Forest models -
randomForest::randomForest()
- Random Forest models, via
ranger
-ranger::ranger()
- MARS models -
earth::earth()
- XGBoost models -
xgboost::xgb.Booster.complete()
- Cubist models -
Cubist::cubist()
- Tree models, via
partykit
-partykit::ctree()
parsnip
tidypredict
supports models fitted via the parsnip
interface. The
ones confirmed currently work in tidypredict
are:
-
lm()
-parsnip
:linear_reg()
with “lm” as the engine. -
randomForest::randomForest()
-parsnip
:rand_forest()
with “randomForest” as the engine. -
ranger::ranger()
-parsnip
:rand_forest()
with “ranger” as the engine. -
earth::earth()
-parsnip
:mars()
with “earth” as the engine.
broom
The tidy()
function from broom works with linear models parsed via
tidypredict
pm <- parse_model(lm(wt ~ ., mtcars))
tidy(pm)
## # A tibble: 11 x 2
## term estimate
## <chr> <dbl>
## 1 (Intercept) -0.231
## 2 mpg -0.0417
## 3 cyl -0.0573
## 4 disp 0.00669
## 5 hp -0.00323
## 6 drat -0.0901
## 7 qsec 0.200
## 8 vs -0.0664
## 9 am 0.0184
## 10 gear -0.0935
## 11 carb 0.249
Contributing
This project is released with a Contributor Code of Conduct. By contributing to this project, you agree to abide by its terms.
-
For questions and discussions about tidymodels packages, modeling, and machine learning, please post on RStudio Community.
-
If you think you have encountered a bug, please submit an issue.
-
Either way, learn how to create and share a reprex (a minimal, reproducible example), to clearly communicate about your code.
-
Check out further details on contributing guidelines for tidymodels packages and how to get help.