gadenbuie / Tidyexplain
Programming Languages
Projects that are alternatives of or similar to Tidyexplain
Tidy Animated Verbs
Garrick Aden-Buie β @grrrck β garrickadenbuie.com. Set operations contributed by Tyler Grant Smith.
-
Mutating Joins β
inner_join()
,left_join()
,right_join()
,full_join()
-
Set Operations β
union()
,union_all()
,intersect()
,setdiff()
-
Learn more about
Background
Usage
Please feel free to use these images for teaching or learning about action verbs from the tidyverse. You can directly download the original animations or static images in svg or png formats, or you can use the scripts to recreate the images locally.
Currently, the animations cover the dplyr two-table verbs and Iβd like to expand the animations to include more verbs from the tidyverse. Suggestions are welcome!
Relational Data
The Relational Data chapter of the R for Data Science book by Garrett Grolemund and Hadley Wickham is an excellent resource for learning more about relational data.
The dplyr two-table verbs vignette and Jenny Bryanβs Cheatsheet for dplyr join functions are also great resources.
gganimate
The animations were made possible by the newly re-written gganimate package by Thomas Lin Pedersen (original by Dave Robinson). The package readme provides an excellent (and quick) introduction to gganimate.
Dynamic Animations
Thanks to an initial push by David Zimmermann, we have begun work toward a packaged set of functions to generate dynamic explanatory animations from users' actual data. Please visit the pkg branch of the tidyexplain repository for more information (or to contribute!).
Mutating Joins
A mutating join allows you to combine variables from two tables. It first matches observations by their keys, then copies across variables from one table to the other.
R for Data Science: Mutating joins
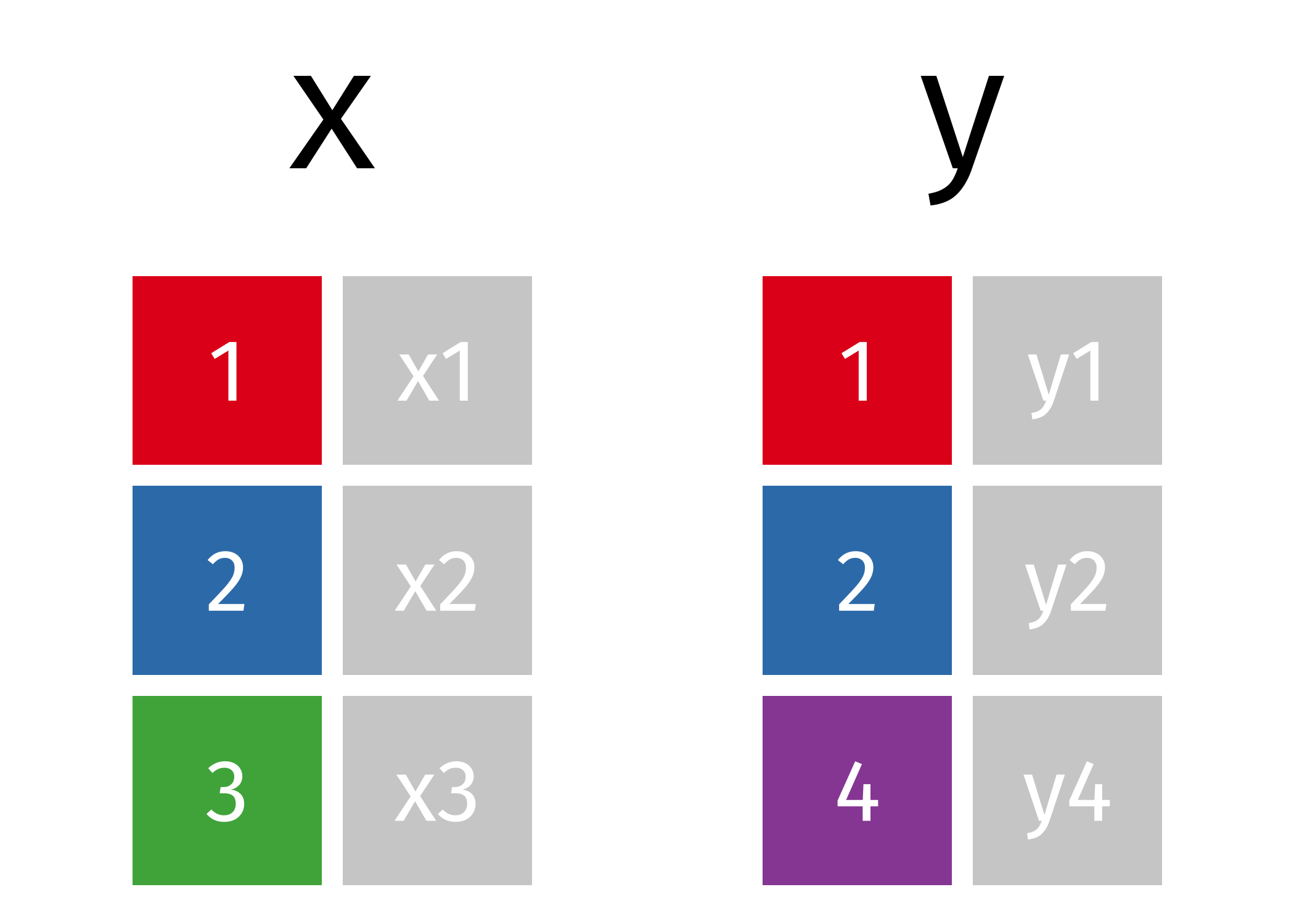
x
#> # A tibble: 3 x 2
#> id x
#> <int> <chr>
#> 1 1 x1
#> 2 2 x2
#> 3 3 x3
y
#> # A tibble: 3 x 2
#> id y
#> <int> <chr>
#> 1 1 y1
#> 2 2 y2
#> 3 4 y4
Inner Join
All rows from
x
where there are matching values iny
, and all columns fromx
andy
.
inner_join(x, y, by = "id")
#> # A tibble: 2 x 3
#> id x y
#> <int> <chr> <chr>
#> 1 1 x1 y1
#> 2 2 x2 y2
Left Join
All rows from
x
, and all columns fromx
andy
. Rows inx
with no match iny
will haveNA
values in the new columns.
left_join(x, y, by = "id")
#> # A tibble: 3 x 3
#> id x y
#> <int> <chr> <chr>
#> 1 1 x1 y1
#> 2 2 x2 y2
#> 3 3 x3 <NA>
Left Join (Extra Rows in y)
β¦ If there are multiple matches between
x
andy
, all combinations of the matches are returned.
y_extra # has multiple rows with the key from `x`
#> # A tibble: 4 x 2
#> id y
#> <dbl> <chr>
#> 1 1 y1
#> 2 2 y2
#> 3 4 y4
#> 4 2 y5
left_join(x, y_extra, by = "id")
#> # A tibble: 4 x 3
#> id x y
#> <dbl> <chr> <chr>
#> 1 1 x1 y1
#> 2 2 x2 y2
#> 3 2 x2 y5
#> 4 3 x3 <NA>
Right Join
All rows from y, and all columns from
x
andy
. Rows iny
with no match inx
will haveNA
values in the new columns.
right_join(x, y, by = "id")
#> # A tibble: 3 x 3
#> id x y
#> <int> <chr> <chr>
#> 1 1 x1 y1
#> 2 2 x2 y2
#> 3 4 <NA> y4
Full Join
All rows and all columns from both
x
andy
. Where there are not matching values, returnsNA
for the one missing.
full_join(x, y, by = "id")
#> # A tibble: 4 x 3
#> id x y
#> <int> <chr> <chr>
#> 1 1 x1 y1
#> 2 2 x2 y2
#> 3 3 x3 <NA>
#> 4 4 <NA> y4
Filtering Joins
Filtering joins match observations in the same way as mutating joins, but affect the observations, not the variables. β¦ Semi-joins are useful for matching filtered summary tables back to the original rows. β¦ Anti-joins are useful for diagnosing join mismatches.
R for Data Science: Filtering Joins
Semi Join
All rows from
x
where there are matching values iny
, keeping just columns fromx
.
semi_join(x, y, by = "id")
#> # A tibble: 2 x 2
#> id x
#> <int> <chr>
#> 1 1 x1
#> 2 2 x2
Anti Join
All rows from
x
where there are not matching values iny
, keeping just columns fromx
.
anti_join(x, y, by = "id")
#> # A tibble: 1 x 2
#> id x
#> <int> <chr>
#> 1 3 x3
Set Operations
Set operations are occasionally useful when you want to break a single complex filter into simpler pieces. All these operations work with a complete row, comparing the values of every variable. These expect the x and y inputs to have the same variables, and treat the observations like sets.
R for Data Science: Set operations
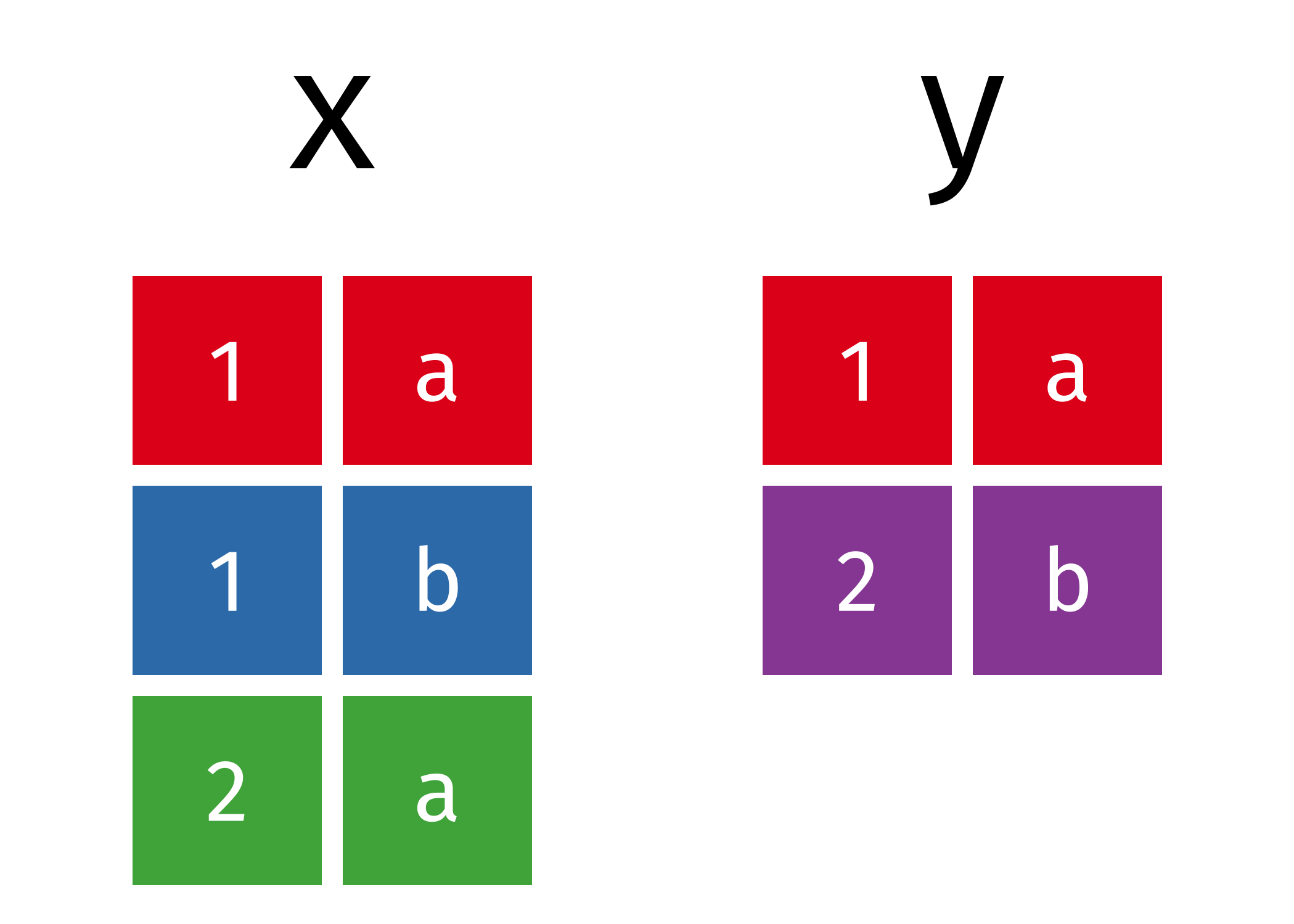
x
#> # A tibble: 3 x 2
#> x y
#> <chr> <chr>
#> 1 1 a
#> 2 1 b
#> 3 2 a
y
#> # A tibble: 2 x 2
#> x y
#> <chr> <chr>
#> 1 1 a
#> 2 2 b
Union
All unique rows from
x
andy
.
union(x, y)
#> # A tibble: 4 x 2
#> x y
#> <chr> <chr>
#> 1 2 b
#> 2 2 a
#> 3 1 b
#> 4 1 a
union(y, x)
#> # A tibble: 4 x 2
#> x y
#> <chr> <chr>
#> 1 2 a
#> 2 1 b
#> 3 2 b
#> 4 1 a
Union All
All rows from
x
andy
, keeping duplicates.
union_all(x, y)
#> # A tibble: 5 x 2
#> x y
#> <chr> <chr>
#> 1 1 a
#> 2 1 b
#> 3 2 a
#> 4 1 a
#> 5 2 b
Intersection
Common rows in both
x
andy
, keeping just unique rows.
intersect(x, y)
#> # A tibble: 1 x 2
#> x y
#> <chr> <chr>
#> 1 1 a
Set Difference
All rows from
x
which are not also rows iny
, keeping just unique rows.
setdiff(x, y)
#> # A tibble: 2 x 2
#> x y
#> <chr> <chr>
#> 1 1 b
#> 2 2 a
setdiff(y, x)
#> # A tibble: 1 x 2
#> x y
#> <chr> <chr>
#> 1 2 b
Tidy Data
Tidy data follows the following three rules:
- Each variable has its own column.
- Each observation has its own row.
- Each value has its own cell.
Many of the tools in the tidyverse expect data to be formatted as a tidy dataset and the tidyr package provides functions to help you organize your data into tidy data.
wide
#> # A tibble: 2 x 4
#> id x y z
#> <int> <chr> <chr> <chr>
#> 1 1 a c e
#> 2 2 b d f
long
#> # A tibble: 6 x 3
#> id key val
#> <int> <chr> <chr>
#> 1 1 x a
#> 2 2 x b
#> 3 1 y c
#> 4 2 y d
#> 5 1 z e
#> 6 2 z f
Spread and Gather
spread(data, key, value)
Spread a key-value pair across multiple columns. Use it when an a column contains observations from multiple variables.
gather(data, key = "key", value = "value", ...)
Gather takes multiple columns and collapses into key-value pairs, duplicating all other columns as needed. You use
gather()
when you notice that your column names are not names of variables, but values of a variable.
gather(wide, key, val, x:z)
#> # A tibble: 6 x 3
#> id key val
#> <int> <chr> <chr>
#> 1 1 x a
#> 2 2 x b
#> 3 1 y c
#> 4 2 y d
#> 5 1 z e
#> 6 2 z f
spread(long, key, val)
#> # A tibble: 2 x 4
#> id x y z
#> <int> <chr> <chr> <chr>
#> 1 1 a c e
#> 2 2 b d f